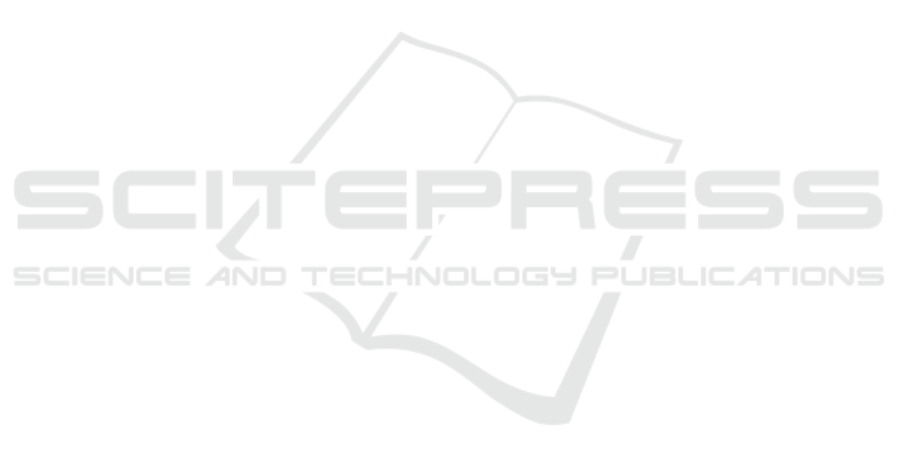
REFERENCES
Badrinarayanan, V., Kendall, A., and Cipolla, R. (2017).
SegNet: A Deep Convolutional Encoder-Decoder Ar-
chitecture for Image Segmentation. IEEE Transac-
tions on Pattern Analysis and Machine Intelligence,
39:2481–2495.
Bochkovskiy, A., Wang, C.-Y., and Liao, H. (2020).
YOLOv4: Optimal Speed and Accuracy of Object De-
tection. ArXiv, abs/2004.10934.
Fan, Z., Wu, Y., Lu, J., and Li, W. (2018). Automatic Pave-
ment Crack Detection Based on Structured Predic-
tion with the Convolutional Neural Network. ArXiv,
abs/1802.02208.
Inzerillo, L., Di Mino, G., and Roberts, R. (2018). Image-
based 3D reconstruction using traditional and UAV
datasets for analysis of road pavement distress. Au-
tomation in Construction, 96:457–469.
Jenkins, M., Carr, T. A., Iglesias, M. I., Buggy, T., and
Morison, G. (2018). A Deep Convolutional Neu-
ral Network for Semantic Pixel-Wise Segmentation
of Road and Pavement Surface Cracks. 2018 26th
European Signal Processing Conference (EUSIPCO),
pages 2120–2124.
Jocher, G., Stoken, A., Borovec, J., NanoCode012, Chaura-
sia, A., TaoXie, Changyu, L., V, A., Laughing,
tkianai, yxNONG, Hogan, A., lorenzomammana,
AlexWang1900, Hajek, J., Diaconu, L., Marc, Kwon,
Y., oleg, wanghaoyang0106, Defretin, Y., Lohia, A.,
ml5ah, Milanko, B., Fineran, B., Khromov, D., Yiwei,
D., Doug, Durgesh, and Ingham, F. (2021). ultralyt-
ics/yolov5: v5.0 - YOLOv5-P6 1280 models, AWS,
Supervise.ly and YouTube integrations.
Li, S., Gu, X., Xu, X., Xu, D., Zhang, T., Liu, Z., and
Dong, Q. (2021). Detection of concealed cracks from
ground penetrating radar images based on deep learn-
ing algorithm. Construction and Building Materials,
273:121949.
Lin, T.-Y., Doll
´
ar, P., Girshick, R., He, K., Hariharan, B.,
and Belongie, S. (2017). Feature pyramid networks
for object detection. In Proceedings of the IEEE Con-
ference on Computer Vision and Pattern Recognition,
pages 2117–2125.
Majidifard, H., Jin, P., Adu-Gyamfi, Y., and Buttlar, W.
(2019). Pavement Image Datasets: A New Benchmark
Dataset to Classify and Densify Pavement Distresses.
Transportation Research Record, 2674:328–339.
Mandal, V., Uong, L., and Adu-Gyamfi, Y. (2018). Au-
tomated Road Crack Detection Using Deep Convo-
lutional Neural Networks. 2018 IEEE International
Conference on Big Data (Big Data), pages 5212–
5215.
Nie, M. and Wang, C. (2019). Pavement Crack Detection
based on YOLO v3. 2019 2nd International Con-
ference on Safety Produce Informatization (IICSPI),
pages 327–330.
Ozyesil, O., Voroninski, V., Basri, R., and Singer, A.
(2017). A survey of structure from motion. arXiv
preprint arXiv:1701.08493.
Redmon, J. and Farhadi, A. (2017). YOLO9000: Better,
Faster, Stronger. 2017 IEEE Conference on Computer
Vision and Pattern Recognition (CVPR), pages 6517–
6525.
Redmon, J. and Farhadi, A. (2018). YOLOv3: An Incre-
mental Improvement. ArXiv, abs/1804.02767.
Ronneberger, O., Fischer, P., and Brox, T. (2015). U-Net:
Convolutional Networks for Biomedical Image Seg-
mentation. ArXiv, abs/1505.04597.
Sarmiento, J. (2021). Pavement Distress Detection and Seg-
mentation using YOLOv4 and DeepLabv3 on Pave-
ments in the Philippines. ArXiv, abs/2103.06467.
Sarsam, S. I., Daham, A. M., and Ali, A. M. (2015). Imple-
mentation of Close Range Photogrammetry to Eval-
uate Distresses at Asphalt Pavement Surface. Inter-
national Journal of Transportation Engineering and
Traffic System, 1(1):1–14.
Tan, Y. and Li, Y. (2019). UAV Photogrammetry-Based 3D
Road Distress Detection. ISPRS Int. J. Geo Inf., 8:409.
Wang, C.-Y., Liao, H.-Y. M., Wu, Y.-H., Chen, P.-Y.,
Hsieh, J.-W., and Yeh, I.-H. (2020). CSPNet: A
new backbone that can enhance learning capability of
CNN. In Proceedings of the IEEE/CVF Conference on
Computer Vision and Pattern Recognition Workshops,
pages 390–391.
Wang, K., Liew, J. H., Zou, Y., Zhou, D., and Feng, J.
(2019). Panet: Few-shot image semantic segmenta-
tion with prototype alignment. In Proceedings of the
IEEE/CVF International Conference on Computer Vi-
sion, pages 9197–9206.
Xu, R., Lin, H., Lu, K., Cao, L., and Liu, Y. (2021). A For-
est Fire Detection System Based on Ensemble Learn-
ing. Forests, 12(2):217.
Zhang, A., Wang, K., Fei, Y., Liu, Y., Chen, C., Yang,
G., Li, J., Yang, E., and Qiu, S. (2019). Automated
Pixel-Level Pavement Crack Detection on 3D Asphalt
Surfaces with a Recurrent Neural Network. Comput.
Aided Civ. Infrastructure Eng., 34:213–229.
Zhang, C. and Elaksher, A. (2012). An Unmanned Aerial
Vehicle-Based Imaging System for 3D Measurement
of Unpaved Road Surface Distresses. Comput. Aided
Civ. Infrastructure Eng., 27:118–129.
Zhang, L., Yang, F., Zhang, Y., and Zhu, Y. (2016). Road
crack detection using deep convolutional neural net-
work. 2016 IEEE International Conference on Image
Processing (ICIP), pages 3708–3712.
Zou, Q., Zhang, Z., Li, Q., Qi, X., Wang, Q., and Wang,
S. (2019). DeepCrack: Learning Hierarchical Convo-
lutional Features for Crack Detection. IEEE Transac-
tions on Image Processing, 28:1498–1512.
Towards a Low-cost Vision System for Real-time Pavement Condition Assessment
533