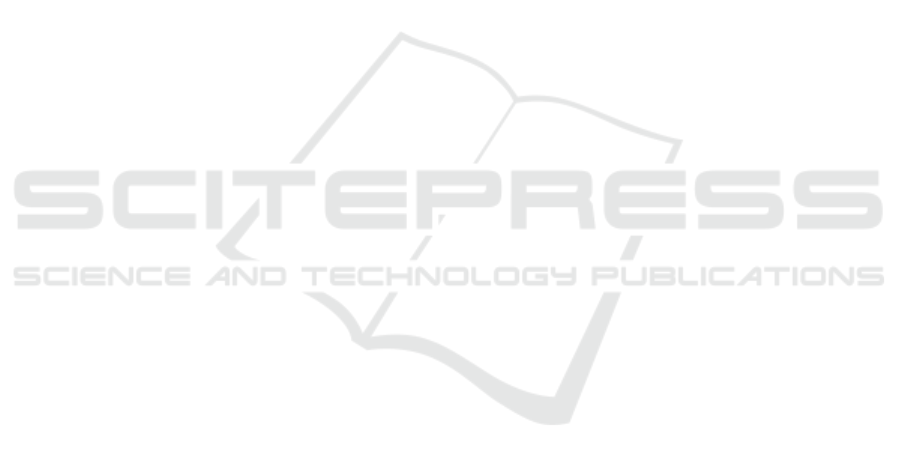
existing solutions to look up context based control pa-
rameters with the demonstrated inference system. It
appears promising to extend the control parameters
to infer, e.g., covariances for localization filters de-
pending on the traction to expect on certain surfaces
or additional behavioral strategies like acoustic or op-
tical signalling when expecting to act in the vicinity
of humans or animals. Also strategies like not opti-
mizing for shortest paths but instead following right
hand rules when driving along paths or streets might
be beneficial. In a wider perspective the extension
towards probabilistic reasoning appears sensible to
cope with information not easily conveyed with sim-
ple facts.
The generation of fine-grained navigation
costmaps provides the foundation for further work:
As a future avenue we plan to use the semantic
representation to map detected obstacles and annotate
additional information. In the long term there are
many kinds of obstacles which might move in the
scale of minutes, hours or sometimes days. Instead
of just adding them to the costmap and remove them,
once not seen anymore, it might be better to actively
check once a certain amount of time has passed or
not regard them for path planning.
Using semantic reasoning technologies can be an
important contribution to add to the flexibility and
thus robustness of robots expected to act in complex
environments without human supervision. Making
knowledge about the environment explicit can add
to the explainability of artificial intelligent decisions
made by robots as well as to the ease in identifying
relevant rules and facts with the help of experts in the
respective field like agriculture.
ACKNOWLEDGEMENTS
The DFKI Niedersachsen Lab (DFKI NI) is spon-
sored by the Ministry of Science and Culture of
Lower Saxony and the VolkswagenStiftung. The
paper describes work that has been developed in
the context of the funded projects Experimentier-
feld Agro-Nordwest (BMEL, 28DE103E18), DAKIS
(BMBF, 031B0729B) and ZLA (NiMWK, Volkswa-
genstiftung, ZDIN 11-76251-14-3/19).
REFERENCES
Bergerman, M., Billingsley, J., Reid, J., and Henten, E. V.
(2016). Robotics in Agriculture and Forestry. In Sicil-
iano, B. and Khatib, O., editors, Springer Handbook
of Robotics, chapter 56, pages 1463–1492. Springer.
Buschka, P. (2005). An investigation of hybrid maps for
mobile robots. PhD thesis, University
¨
Orebro.
Cosgun, A. and Christensen, H. I. (2018). Context-aware
robot navigation using interactively built semantic
maps. Paladyn, Journal of Behavioral Robotics,
9(1):254–276.
Crespo, J., Castillo, J. C., Mozos, O. M., and Barber, R.
(2020). Semantic Information for Robot Navigation:
A Survey. Applied Sciences, 10(2):497.
Deeken, H., Wiemann, T., and Hertzberg, J. (2019). A
spatio-semantic approach to reasoning about agricul-
tural processes. Applied Intelligence, 49(11):3821–
3833.
Deeken, H., Wiemann, T., Lingemann, K., and Hertzberg,
J. (2015). SEMAP - a semantic environment mapping
framework. In 2015 European Conference on Mobile
Robots (ECMR), pages 1–6. IEEE.
Egerstedt, M., Pauli, J. N., Notomista, G., and Hutchinson,
S. (2018). Robot ecology: Constraint-based control
design for long duration autonomy. Annual Reviews
in Control, 46:1–7.
Forgy, C. L. (1982). Rete: A fast algorithm for the many
pattern/many object pattern match problem. Artificial
Intelligence, 19(1):17–37.
Fox, D., Burgard, W., and Thrun, S. (1997). The dy-
namic window approach to collision avoidance. IEEE
Robotics Automation Magazine, 4(1):23–33.
Galindo, C., Saffiotti, A., Coradeschi, S., Buschka, P.,
Fernandez-Madrigal, J., and Gonzalez, J. (2005).
Multi-hierarchical semantic maps for mobile robotics.
In 2005 IEEE/RSJ International Conference on Intel-
ligent Robots and Systems, pages 2278–2283. IEEE.
Hoellmann, M., Kisliuk, B., Krause, J. C., Tieben, C.,
Mock, A., Sebastian, P., Igelbrink, F., Wiemann,
T., Martinez, S. F., Stiene, S., and Hertzberg, J.
(2020). Towards Context-Aware Navigation for Long-
Term Autonomy in Agricultural Environments. In
2020 IEEE/RSJ International Conference on Intelli-
gent Robots and Systems (IROS), Las Vegas.
Kostavelis, I. and Gasteratos, A. (2015). Semantic mapping
for mobile robotics tasks: A survey. Robotics and
Autonomous Systems, 66:86–103.
Kuipers, B. (2000). The Spatial Semantic Hierarchy. Artifi-
cial Intelligence, 119(1-2):191–233.
Kunze, L., Burbridge, C., Alberti, M., Thippur, A.,
Folkesson, J., Jensfelt, P., and Hawes, N. (2014).
Combining top-down spatial reasoning and bottom-
up object class recognition for scene understanding.
In 2014 IEEE/RSJ International Conference on Intel-
ligent Robots and Systems, pages 2910–2915. IEEE.
Kunze, L., Hawes, N., Duckett, T., Hanheide, M., and Kra-
jnik, T. (2018). Artificial Intelligence for Long-Term
Robot Autonomy: A Survey. IEEE Robotics and Au-
tomation Letters, 3(4):4023–4030.
Lang, D. and Paulus, D. (2014). Semantic Maps for
Robotics. In Proceedings of the Workshop on AI
Robotics at the IEEE International Conference on
Robotics and Automation.
Langley, P., Meadows, B., and Sridharan, M. (2017). Ex-
plainable agency for intelligent autonomous systems.
ICAART 2022 - 14th International Conference on Agents and Artificial Intelligence
162