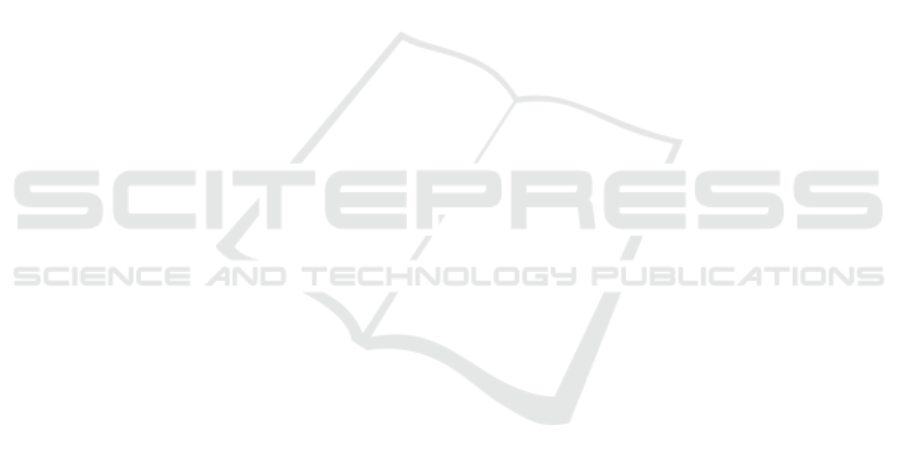
Computer Engineering, Chalmers University of Tech-
nology, G
¨
oteborg, Sweden.
Friedman, J. (2000). Greedy function approximation: A
gradient boosting machine. The Annals of Statistics,
29.
Friedman, J. and Popescu, B. (2008). Predictive learning
via rule ensembles. The Annals of Applied Statistics,
2.
Goldstein, A., Kapelner, A., Bleich, J., and Pitkin, E.
(2015). Peeking inside the black box: Visualizing
statistical learning with plots of individual conditional
expectation. journal of Computational and Graphical
Statistics, 24(1):44–65.
Hindy, H., Brosset, D., Bayne, E., Seeam, A., Tachtatzis,
C., Atkinson, R., and Bellekens, X. (2018). A taxon-
omy and survey of intrusion detection system design
techniques, network threats and datasets. Technical
report, University of Strathclyde, Glasgow.
Hu, Y., Yang, A., Li, H., Sun, Y., and Sun, L. (2018). A
survey of intrusion detection on industrial control sys-
tems. International Journal of Distributed Sensor Net-
works, 14(8):1550147718794615.
Inoue, J., Yamagata, Y., Chen, Y., Poskitt, C. M., and Sun,
J. (2017). Anomaly detection for a water treatment
system using unsupervised machine learning. In 2017
IEEE international conference on data mining work-
shops (ICDMW), pages 1058–1065. IEEE.
Khan, A. A. Z. (2019). Misuse intrusion detection using
machine learning for gas pipeline scada networks. In
Proceedings of the International Conference on Secu-
rity and Management (SAM), pages 84–90.
Kravchik, M. and Shabtai, A. (2018). Detecting cyber at-
tacks in industrial control systems using convolutional
neural networks. In Proceedings of the 2018 Work-
shop on Cyber-Physical Systems Security and Pri-
vaCy, pages 72–83.
Lai, Y., Zhang, J., and Liu, Z. (2019). Industrial anomaly
detection and attack classification method based on
convolutional neural network. Security and Commu-
nication Networks, 2019.
Lavrova, D., Zegzhda, D., and Yarmak, A. (2019). Using
gru neural network for cyber-attack detection in auto-
mated process control systems. In 2019 IEEE Interna-
tional Black Sea Conference on Communications and
Networking (BlackSeaCom), pages 1–3. IEEE.
Li, H., Wei, F., and Hu, H. (2019). Enabling dynamic net-
work access control with anomaly-based ids and sdn.
In Proceedings of the ACM International Workshop
on Security in Software Defined Networks & Network
Function Virtualization, pages 13–16.
Linardatos, P., Papastefanopoulos, V., and Kotsiantis, S.
(2021). Explainable ai: A review of machine learn-
ing interpretability methods. Entropy, 23(1):18.
Marino, D. L., Wickramasinghe, C. S., and Manic, M.
(2018). An adversarial approach for explainable ai in
intrusion detection systems. In 44th Annual Confer-
ence of the IEEE Industrial Electronics Society, pages
3237–3243. IEEE.
Markov, Z. and Russell, I. (2006). An introduction to the
weka data mining system. ACM SIGCSE Bulletin,
38(3):367–368.
Mathur, A. P. and Tippenhauer, N. O. (2016). Swat: a wa-
ter treatment testbed for research and training on ics
security. In 2016 International Workshop on Cyber-
physical Systems for Smart Water Networks, pages
31–36. IEEE.
Mitchell, R. and Chen, I.-R. (2014). A survey of intrusion
detection techniques for cyber-physical systems. ACM
Computing Surveys (CSUR), 46(4):1–29.
Morris, T. and Gao, W. (2014). Industrial control system
traffic data sets for intrusion detection research. In In-
ternational Conference on Critical Infrastructure Pro-
tection, pages 65–78. Springer.
Morris, T. H., Thornton, Z., and Turnipseed, I. (2015). In-
dustrial control system simulation and data logging
for intrusion detection system research. 7th annual
southeastern cyber security summit, pages 3–4.
Papernot, N., McDaniel, P., Goodfellow, I., Jha, S., Celik,
Z. B., and Swami, A. (2017). Practical black-box at-
tacks against machine learning.
Perez, R. L., Adamsky, F., Soua, R., and Engel, T. (2018).
Machine learning for reliable network attack detection
in scada systems. In 2018 17th IEEE International
Conference On Trust, Security And Privacy In Com-
puting And Communications/12th IEEE International
Conference On Big Data Science And Engineering
(TrustCom/BigDataSE), pages 633–638. IEEE.
Scarfone, K. and Mell, P. (2007). Guide to intrusion de-
tection and prevention systems (idps). NIST special
publication, 800(2007):94.
Shirazi, S. N., Gouglidis, A., Syeda, K. N., Simpson, S.,
Mauthe, A., Stephanakis, I. M., and Hutchison, D.
(2016). Evaluation of anomaly detection techniques
for scada communication resilience. In 2016 Re-
silience Week (RWS), pages 140–145. IEEE.
Sokolov, A. N., Pyatnitsky, I. A., and Alabugin, S. K.
(2019). Applying methods of machine learning in the
task of intrusion detection based on the analysis of in-
dustrial process state and ics networking. FME Trans-
actions, 47(4):782–789.
Sommer, R. and Paxson, V. (2010). Outside the closed
world: On using machine learning for network intru-
sion detection. In 2010 IEEE Symposium on Security
and Privacy, pages 305–316.
Tavallaee, M., Bagheri, E., Lu, W., and Ghorbani, A. A.
(2009). A detailed analysis of the kdd cup 99 data
set. In 2009 IEEE symposium on computational intel-
ligence for security and defense applications, pages
1–6. IEEE.
Wang, M., Zheng, K., Yang, Y., and Wang, X. (2020). An
explainable machine learning framework for intrusion
detection systems. IEEE Access, 8:73127–73141.
Zolanvari, M., Teixeira, M. A., Gupta, L., Khan, K. M.,
and Jain, R. (2019). Machine learning-based network
vulnerability analysis of industrial internet of things.
IEEE Internet of Things Journal, 6(4):6822–6834.
Towards a Better Understanding of Machine Learning based Network Intrusion Detection Systems in Industrial Networks
325