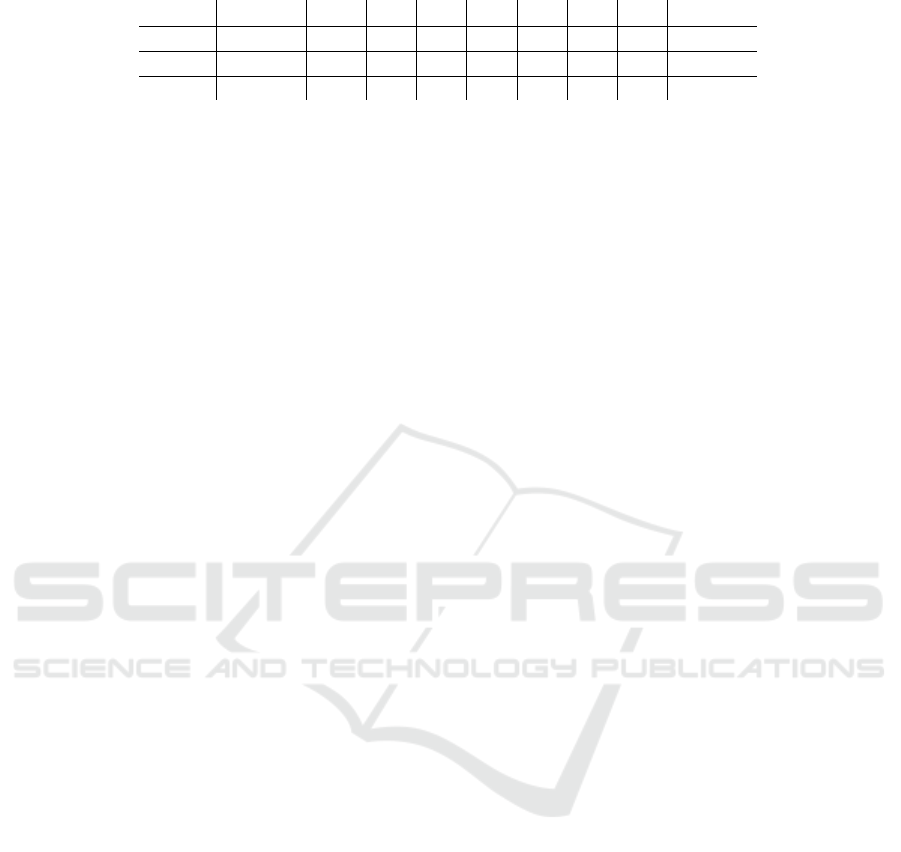
Table 2: Hyper-parameters for the reference Gaussian processes.
GP σ
2
f
l
F
0
l
F
1
l
F
2
l
U
1
l
U
2
l
T
1
l
T
2
σ
2
n
ˆµ
F
S
(θ) 4.4 ·10
5
13.7 2 1.9 1.7 1.4 2.9 2.6 1.6 ·10
3
ˆ
σ
F
S
(θ) 1.7 ·10
3
1.3 1.9 0.2 0.9 0.9 2.3 0.8 335
ˆg 0.3 3 0.7 1.1 0.5 0.5 1 1.3 0.05
Besides a physics-based prior mean function, we
want to investigate other materials, like copper, or dif-
ferent wire diameters. The modification of other gen-
eral conditions could also be considered. Our pro-
posed method can be applied in the same way. How-
ever, we can build on the results from this paper and
examine the field of transfer learning. A good start-
ing point might be the Gaussian processes which were
trained with all the data points from our experiments.
ACKNOWLEDGEMENTS
The research was funded by the Ministry of Eco-
nomic Affairs, Innovation, Digitalisation and Energy
(MWIDE) of the State of North Rhine-Westphalia
within the Leading-Edge Cluster Intelligent Techni-
cal Systems OstWestfalenLippe (it’s OWL) and by
the Federal Ministry of Education and Research of
Germany (BMBF) within the junior research group
DART of the University of Paderborn. The responsi-
bility for the content of this publication lies with the
authors.
The authors would like to thank Yuqi Liu, Jan Her-
bermann and Fabian Reiling for their assistance dur-
ing the experiments.
REFERENCES
Calandra, R., Seyfarth, A., Peters, J., and Deisenroth, M. P.
(2016). Bayesian Optimization for Learning Gaits un-
der Uncertainty. Annals in Mathematics and Artificial
Intelligence, 76:5–23.
D
´
ıaz-Franc
´
es, E. and Rubio, F. J. (2013). On the Existence
of a Normal Approximation to the Distribution of the
Ratio of two Independent Normal Random Variables.
Statistical Papers, 54(2):309–323.
DVS - German Welding Society (2017). Test Procedures for
Wire Bonded Joints (Technical Bulletin DVS 2811).
Geißler, U. (2009). Verbindungsbildung und
Gef
¨
ugeentwicklung beim Ultraschall-Wedge-Wedge-
Bonden von AlSi1-Draht. Technische Universit
¨
at
Berlin, Fakult
¨
at IV - Elektrotechnik und Informatik.
Gogh, B., Benner, T., Seppaenen, H., Tszeng, C., and
Sepehrband, P. (2020). An Oxide Wear Model of Ul-
trasonic Bonding. International Symposium on Micro-
electronics, 2020:222–229.
Gonzalez, J., Dai, Z., Hennig, P., and Lawrence, N. (2016).
Batch Bayesian Optimization via Local Penalization.
In AISTATS 2016; 19th International Conference on
Artificial Intelligence and Statistics.
Harman, G. (2010). Wire Bonding in Microelectronics.
McGraw-Hill.
Hunstig, M., Schaermann, W., Broekelmann, M.,
Holtkaemper, S., Siepe, D., and Hesse, H. J.
(2020). Smart Ultrasonic Welding in Power Elec-
tronics Packaging. In CIPS 2020; 11th International
Conference on Integrated Power Electronics Systems,
pages 1–6.
Jones, D. R., Schonlau, M., and Welch, W. J. (1998).
Efficient Global Optimization of Expensive Black-
Box Functions. Journal of Global Optimization,
13(4):455–492.
Kelly, M. (2017). An Introduction to Trajectory Optimiza-
tion: How to Do Your Own Direct Collocation. SIAM
Review, 59(4):849–904.
Long, Y., Twiefel, J., and Wallaschek, J. (2017). A Re-
view on the Mechanisms of Ultrasonic Wedge-Wedge
Bonding. Journal of Materials Processing Technol-
ogy, 245:241–258.
Mayer, M. and Schwizer, J. (2002). Ultrasonic Bond-
ing: Understanding How Process Parameters Deter-
mine the Strength of Au-Al Bonds. Symposium on
Microelectronics, IMAPS, Denver.
Neumann-Brosig, M., Marco, A., Schwarzmann, D., and
Trimpe, J. S. (2020). Data-efficient Auto-tuning with
Bayesian Optimization: An Industrial Control Study.
IEEE Transactions on Control Systems Technology,
28(5):730–740.
Rasmussen, C. E. and Williams, C. (2006). Gaussian Pro-
cesses for Machine Learning. MIT Press.
Schemmel, R., Krieger, V., Hemsel, T., and Sextro, W.
(2020). Co-Simulation of MATLAB and ANSYS for
Ultrasonic Wire Bonding Process Optimization. In
2020 21st International Conference on Thermal, Me-
chanical and Multi-Physics Simulation and Experi-
ments in Microelectronics and Microsystems.
Shahriari, B., Swersky, K., Wang, Z., Adams, R. P., and
de Freitas, N. (2016). Taking the Human Out of the
Loop: A Review of Bayesian Optimization. Proceed-
ings of the IEEE, 104(1):148–175.
Snoek, J., Larochelle, H., and Adams, R. P. (2012). Prac-
tical Bayesian Optimization of Machine Learning Al-
gorithms. In Pereira, F., Burges, C. J. C., Bottou, L.,
and Weinberger, K. Q., editors, Advances in Neural
Information Processing Systems, volume 25. Curran
Associates, Inc.
Unger, A., Hunstig, M., Meyer, T., Br
¨
okelmann, M., and
Sextro, W. (2018). Intelligent Production of Wire
Bonds using Multi-Objective Optimization – Insights,
Batch Constrained Bayesian Optimization for Ultrasonic Wire Bonding Feed-forward Control Design
393