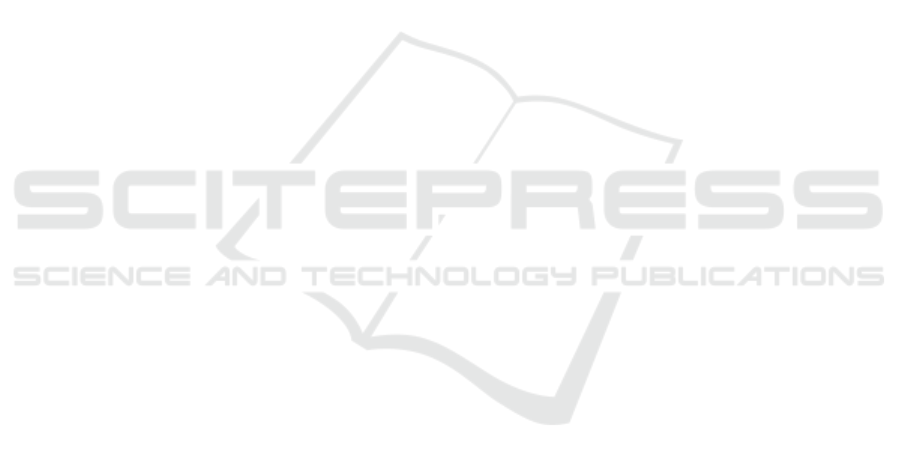
Kammoun, W., Ennaji, A. (2004). Reconnaissance de
textes arabes à vocabulaire ouvert. CIFED, France.
LeCun, Y., Bengio, Y. (1995). Convolutional networks for
images, speech, and time series. The handbook of brain
theory and neural networks, 3361(10), 1995.Bai, J.,
Chen, Z., Feng, B. ,Xu, B. (2014). Image character
recognition using deep convolutional neural network
learned from different languages.IEEE International
Conference on Image Processing (ICIP), pp. 2560-
2564.
Yuan, A., Bai, G., Jiao, L., Liu, Y. (2012). Offline
handwritten english character recognition based on
convolutional neural network. Document Analysis
Systems (DAS), 10th IAPR International Workshop, pp.
125-129.
Wu, C., Fan, W., He, Y., Sun, J., Naoi, S. (2014).
Handwritten character recognition by alternately
trained relaxation convolutional neural network.
Frontiers in Handwriting Recognition (ICFHR), 14th
International Conference, pp. 291-296.
Zhong, Z., Jin, L., Feng, Z. (2015). Multi-font printed
chinese character recognition using multipooling
convolutional neural network.Document Analysis and
Recognition (ICDAR), 13th International Conference,
pp. 96-100.
Yang, W., Jin, L., Xie, Z., Feng, Z. (2015). Improved deep
convolutional neural network for online handwritten
chinese character recognition using domain-specific
knowledge. Proc. Document Analysis and Recognition
(ICDAR), 13th International Conference, pp. 551-555.
He, M., Zhang, S., Mao, H., Jin, L. (2015). Recognition
confidence analysis of handwritten chinese character
with CNN.Proc. Document Analysis and Recognition
(ICDAR), 13th International Conference, pp. 61-65.
Zhong, Z., Jin, L., Xie, Z. (2015). High performance offline
handwritten Chinese character recognition using
GoogLeNet and directional feature maps.Proc.
Document Analysis and R (ICDAR), 13th International
Conference, pp. 846-850.
Kim, I.-J., Xie, X. (2015). Handwritten hangul recognition
using deep convolutional neural networks.
International Journal on Document Analysis and
Recognition (IJDAR), 18, pp. 1-3.
Anil, R., Manjusha, K., Kumar, S.S., Soman, K.P. (2015).
Convolutional neural networks for the recognition of
malayalam characters.Proc. Document Analysis and
Recognition (ICDAR), 13th International Conference,
pp. 1041-1045.
Acharya, S., Pant, A.K., Gyawali, P.K. (2015). Deep
learning based large scale handwritten devenegari
character recognition. 9th International Conference on
Software, Knowledge, Information Management and
Applications (SKIMA), pp. 1-6.
Singh, P., Verma, A., Chaudhari, N.S. (2016). Deep
convolutional neural network classifier for handwritten
devanagari character recognition. Information Systems
Design and Intelligent Applications: Proceedings of
Third International Conference INDIA, 2, pp. 551-561.
Soman, S.T., Nandigam, A., Chakravarthv, V.S. (2013). An
efficient multiclassifier system based on convolutional
neural network for offline handwritten Teugu character
recognition.Proc. Communications (NCC), 2013
National Conference, pp. 1-5.
Fukushima, K. (1980). Neocognitron: A self-organizing
neural network model for a mechanism of pattern
recognition unaffected by shift in position. Biological
cybernetics, 36(4):193–202.
LeCun, Y., Boser, B., Denker, J.S., Henderson, D., Howard,
R.E., Hubbardet, W., Jackel, L.D. (1990). Handwritten
digit recognition with a backpropagation network.
Advances in Neural Information Processing Systems,
pages 396–404.
Poisson, E., Viard-Gaudin, C. (2001). Réseaux de neurones
à convolution: reconnaissance de l’écriture manuscrite
non contrainte.Polytechnic School of the University of
Nantes, France.
Graves, A., Fernandez, S., Liwicki, M., Bunke, H.,
Schmidhuber, J. (2008). Unconstrained online
handwriting recognition with recurrent neural
networks.Advances in Neural Information Processing
Systems, 20:1-8.
Graves, A., Schmidhuber, J. (2009). Offline handwriting
recognition with multidimensional recurrent neural
networks.Advances in Neural Information Processing
Systems, 21:545-552.
Su, B., Lu, S. (2015). Accurate scene text recognition based
on recurrent neural network. In: Cremers D., Reid I.,
Saito H., Yang MH. (eds) Computer Vision -- ACCV
2014. ACCV 2014. Lecture Notes in Computer Science,
vol 9003. Springer, Cham.
Graves, A., Fernandez, S., Liwicki, M., Bunke, H.,
Schmidhuber, J. (2007). Unconstrained online
handwriting recongnition with recurrent neural
networks.Advances in Neural Information Processing
Systems.
Mioulet, L. (2015). Reconnaissance de l’écriture
manuscrite avec des réseaux récurrents, PhD Thesis,
University of Rouen.
Hamdi, Y., et al. (2019). Hybrid DBLSTM-SVM based
Beta-elliptic-CNN Models for Online Arabic
Characters Recognition. 2019 InternationalConference
on Document Analysis and Recognition (ICDAR).
IEEE.
Agrawal, T., Urolagin, S. (2020). 2-way Arabic Sign
Language translator using CNNLSTM architecture and
NLP.Accepted in ACM, Ei Compendex and Scopus, ISI
Web of Science, International Conference on Big Data
Engineering and Technology BDET-20.
Al Omari, M., Al-Hajj, M., Sabra, A., Hammami, N.
(2019).Hybrid CNNs-LSTM Deep Analyzer for Arabic
Opinion Mining.2019 Sixth International Conference
on Social Networks Analysis, Management and Security
(SNAMS).
Alayba, AM., Palade, V., England, M., Iqbal, R. (2018). A
combined CNN and LSTM model for arabic sentiment
analysis.In International Cross-Domain Conference for
machine learning and knowledge Extraction, pp.197-
191.
ICPRAM 2022 - 11th International Conference on Pattern Recognition Applications and Methods
220