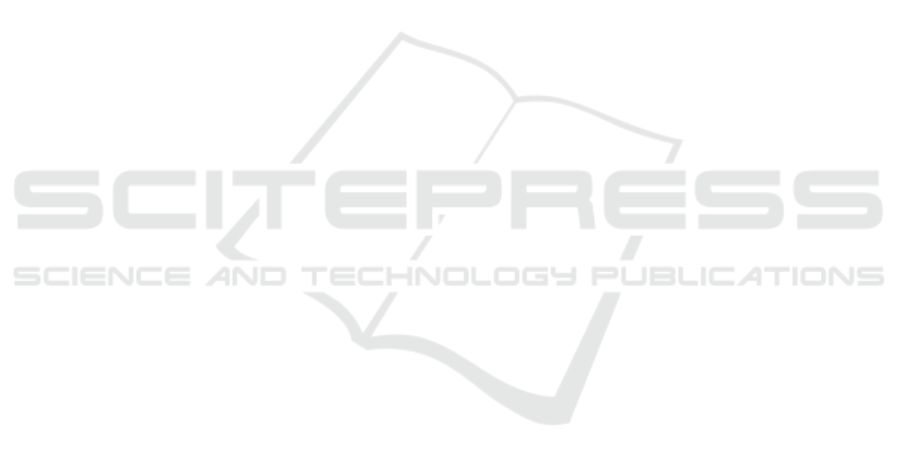
REFERENCES
Chaudhry, A., Dokania, P. K., Ajanthan, T., and Torr, P. H.
(2018). Riemannian walk for incremental learning:
Understanding forgetting and intransigence. In Pro-
ceedings of the European Conference on Computer
Vision (ECCV), pages 532–547.
Fergus, R., Bernal, H., Weiss, Y., and Torralba, A. (2010).
Semantic label sharing for learning with many cate-
gories. In European Conference on Computer Vision,
pages 762–775. Springer.
Goodfellow, I. J., Mirza, M., Xiao, D., Courville, A., and
Bengio, Y. (2013). An empirical investigation of
catastrophic forgetting in gradient-based neural net-
works. arXiv preprint arXiv:1312.6211.
Jung, S., Ahn, H., Cha, S., and Moon, T. (2020). Con-
tinual learning with node-importance based adap-
tive group sparse regularization. arXiv preprint
arXiv:2003.13726.
Kirkpatrick, J., Pascanu, R., Rabinowitz, N., Veness, J.,
Desjardins, G., Rusu, A. A., Milan, K., Quan, J.,
Ramalho, T., Grabska-Barwinska, A., et al. (2017).
Overcoming catastrophic forgetting in neural net-
works. Proceedings of the national academy of sci-
ences, 114(13):3521–3526.
Li, X., Zhou, Y., Wu, T., Socher, R., and Xiong, C. (2019).
Learn to grow: A continual structure learning frame-
work for overcoming catastrophic forgetting. In In-
ternational Conference on Machine Learning, pages
3925–3934. PMLR.
Parisi, G. I., Kemker, R., Part, J. L., Kanan, C., and
Wermter, S. (2019). Continual lifelong learning with
neural networks: A review. Neural Networks, 113:54–
71.
Riemer, M., Klinger, T., Bouneffouf, D., and Franceschini,
M. (2019). Scalable recollections for continual life-
long learning. In Proceedings of the AAAI Conference
on Artificial Intelligence, volume 33, pages 1352–
1359.
Roy, D., Panda, P., and Roy, K. (2020). Tree-CNN: A hi-
erarchical deep convolutional neural network for in-
cremental learning. Neural networks: the official
journal of the International Neural Network Society,
121:148–160.
Russakovsky, O., Deng, J., Su, H., Krause, J., Satheesh, S.,
Ma, S., Huang, Z., Karpathy, A., Khosla, A., Bern-
stein, M., et al. (2015). Imagenet large scale visual
recognition challenge. International journal of com-
puter vision, 115(3):211–252.
Rusu, A. A., Rabinowitz, N. C., Desjardins, G., Soyer,
H., Kirkpatrick, J., Kavukcuoglu, K., Pascanu, R.,
and Hadsell, R. (2016). Progressive neural networks.
arXiv preprint arXiv:1606.04671.
Schwartz, R., Dodge, J., Smith, N. A., and Etzioni, O.
(2020). Green ai. Communications of the ACM,
63(12):54–63.
Shin, H., Lee, J. K., Kim, J., and Kim, J. (2017). Continual
learning with deep generative replay. arXiv preprint
arXiv:1705.08690.
Yoon, J., Yang, E., Lee, J., and Hwang, S. J. (2018). Life-
long learning with dynamically expandable networks.
ICLR.
Zoph, B., Vasudevan, V., Shlens, J., and Le, Q. V. (2018).
Learning transferable architectures for scalable image
recognition. In Proceedings of the IEEE conference on
computer vision and pattern recognition, pages 8697–
8710.
Towards Robust Continual Learning using an Enhanced Tree-CNN
325