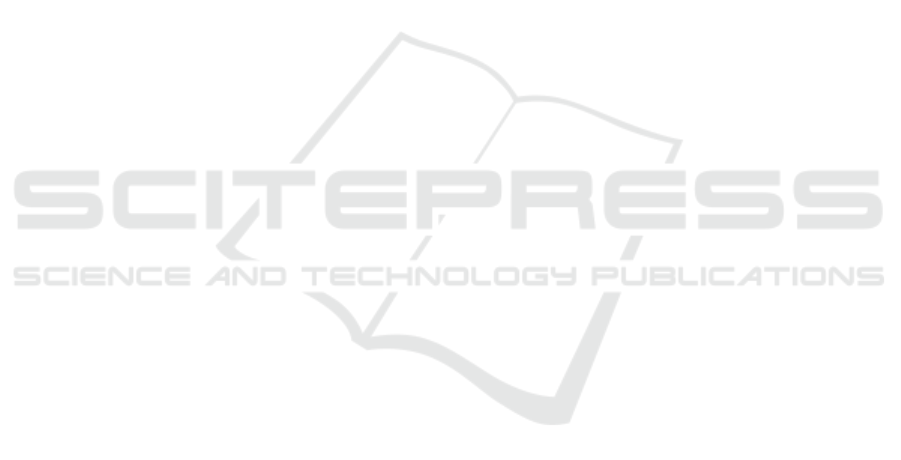
5 CONCLUSION AND FUTURE
WORK
We presented in this paper an optimization-based
approach for ACC reference generation taking into
account the uncertainty associated with sensors
information. As a benchmark for ACC system
decision making, our optimization approach can
generate a reference that meets the needs of safety,
comfort, and effectiveness. According to a statistical
analysis of the simulation results, our chance-
constrained based stochastic model can produce more
robust solutions.
For future work, we propose three open research
challenges that have the merit to be addressed:
development of an increasingly sophisticated vehicle
model, modeling of uncertainty involving dependent
random variables, and formulation of objectives that
involve penalties for undesired behavior. The solution
to those challenges will allow us to build a more
general framework to accommodate different needs
for reference generation problem. Furthermore, we
will use this optimization-based reference generation
framework for other autonomous driving functions,
such as lane keeping assistance (LKA) and collision
avoidance.
REFERENCES
Alnaser, A. J., Akbas, M. I., Sargolzaei, A., and
Razdan, R. (2019). Autonomous vehicles scenario
testing framework and model of computation. SAE
International Journal of Connected and Automated
Vehicles, 2(4).
Chehardoli, H. (2020). Robust optimal control and
identification of adaptive cruise control systems in the
presence of time delay and parameter uncertainties.
Journal of Vibration and Control, 26(17-18):1590–
1601.
Djoudi, A., Coquelin, L., and Regnier, R. (2020).
A simulation-based framework for functional
testing of automated driving controllers. In 2020
IEEE 23rd International Conference on Intelligent
Transportation Systems (ITSC), pages 1–6. IEEE.
Goldfarb, D. and Idnani, A. (1983). A numerically stable
dual method for solving strictly convex quadratic
programs. Mathematical programming, 27(1):1–33.
Kim, S. (2012). Design of the adaptive cruise control
systems: An optimal control approach. PhD thesis,
UC Berkeley.
Lattarulo, R., Heß, D., Matute, J. A., and Perez, J. (2018).
Towards conformant models of automated electric
vehicles. In 2018 IEEE International Conference on
Vehicular Electronics and Safety (ICVES), pages 1–6.
IEEE.
Lattarulo, R., Pérez, J., and Dendaluce, M. (2017).
A complete framework for developing and testing
automated driving controllers. IFAC-PapersOnLine,
50(1):258–263.
Levine, W. and Athans, M. (1966). On the optimal error
regulation of a string of moving vehicles. IEEE
Transactions on Automatic Control, 11(3):355–361.
Li, S., Li, K., Rajamani, R., and Wang, J. (2010).
Model predictive multi-objective vehicular adaptive
cruise control. IEEE Transactions on control systems
technology, 19(3):556–566.
Liang, C.-Y. and Peng, H. (1999). Optimal adaptive cruise
control with guaranteed string stability. Vehicle system
dynamics, 32(4-5):313–330.
Lunze, J. (2018). Adaptive cruise control with guaranteed
collision avoidance. IEEE Transactions on Intelligent
Transportation Systems, 20(5):1897–1907.
Mehra, A., Ma, W.-L., Berg, F., Tabuada, P., Grizzle, J. W.,
and Ames, A. D. (2015). Adaptive cruise control:
Experimental validation of advanced controllers on
scale-model cars. In 2015 American Control
Conference (ACC), pages 1411–1418. IEEE.
Naus, G., Ploeg, J., Van de Molengraft, M., Heemels, W.,
and Steinbuch, M. (2010). Design and implementation
of parameterized adaptive cruise control: An
explicit model predictive control approach. Control
Engineering Practice, 18(8):882–892.
Prékopa, A. (2013). Stochastic programming, volume 324.
Springer Science & Business Media.
Rasshofer, R. H., Spies, M., and Spies, H. (2011).
Influences of weather phenomena on automotive laser
radar systems. Advances in Radio Science, 9(B.
2):49–60.
Schmied, R., Waschl, H., and Del Re, L. (2015). Extension
and experimental validation of fuel efficient predictive
adaptive cruise control. In 2015 American Control
Conference (ACC), pages 4753–4758. IEEE.
Seppelt, B. D. and Lee, J. D. (2015). Modeling driver
response to imperfect vehicle control automation.
Procedia Manufacturing, 3:2621–2628.
Shakouri, P., Czeczot, J., and Ordys, A. (2015). Simulation
validation of three nonlinear model-based controllers
in the adaptive cruise control system. Journal of
Intelligent & Robotic Systems, 80(2):207–229.
Takahama, T. and Akasaka, D. (2018). Model predictive
control approach to design practical adaptive cruise
control for traffic jam. International journal of
automotive engineering, 9(3):99–104.
Weißmann, A., Görges, D., and Lin, X. (2018). Energy-
optimal adaptive cruise control combining model
predictive control and dynamic programming. Control
Engineering Practice, 72:125–137.
Zhu, Y., Zhao, D., and Zhong, Z. (2018). Adaptive optimal
control of heterogeneous cacc system with uncertain
dynamics. IEEE Transactions on Control Systems
Technology, 27(4):1772–1779.
Optimization of Adaptive Cruise Control under Uncertainty
285