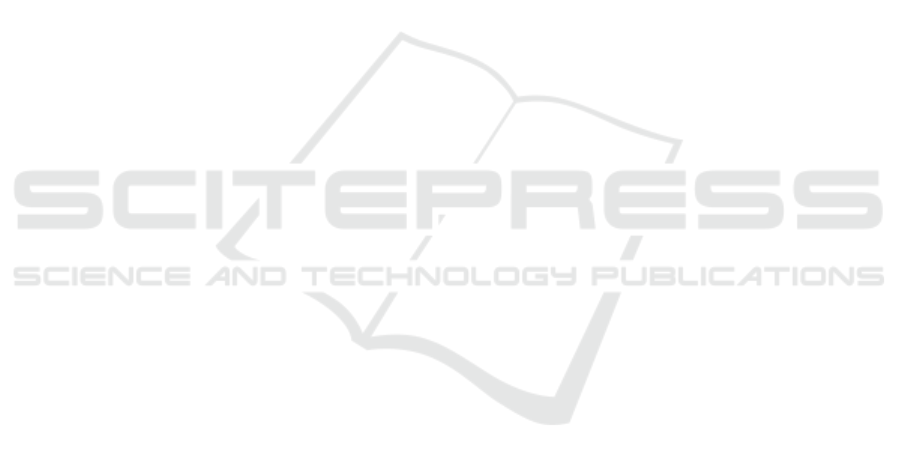
for credibility in modern machine learning. arXiv
preprint arXiv:2011.03395.
Darlow, L. N., Crowley, E. J., Antoniou, A., and Storkey,
A. J. (2018). CINIC-10 is not ImageNet or CIFAR-
10. arXiv preprint arXiv:1810.03505.
d’Ascoli, S., Refinetti, M., Biroli, G., and Krzakala, F.
(2020). Double trouble in double descent: Bias and
variance (s) in the lazy regime. In International
Conference on Machine Learning, pages 2280–2290.
PMLR.
Geiger, M., Jacot, A., Spigler, S., Gabriel, F., Sagun, L.,
d’Ascoli, S., Biroli, G., Hongler, C., and Wyart, M.
(2020). Scaling description of generalization with
number of parameters in deep learning. Journal
of Statistical Mechanics: Theory and Experiment,
2020(2):023401.
He, K., Zhang, X., Ren, S., and Sun, J. (2016). Deep resid-
ual learning for image recognition. In Proceedings of
the IEEE conference on computer vision and pattern
recognition, pages 770–778.
Ishida, T., Yamane, I., Sakai, T., Niu, G., and Sugiyama, M.
(2020). Do we need zero training loss after achieving
zero training error? In International Conference on
Machine Learning, pages 4604–4614. PMLR.
Jacot, A., Gabriel, F., and Hongler, C. (2018). Neural tan-
gent kernel: convergence and generalization in neu-
ral networks. In Proceedings of the 32nd Interna-
tional Conference on Neural Information Processing
Systems, pages 8580–8589.
Lakshminarayanan, B., Pritzel, A., and Blundell, C. (2017).
Simple and scalable predictive uncertainty estimation
using deep ensembles. Advances in Neural Informa-
tion Processing Systems, pages 6402–6413.
Lee, J., Bahri, Y., Novak, R., Schoenholz, S. S., Penning-
ton, J., and Sohl-Dickstein, J. (2017). Deep neu-
ral networks as gaussian processes. arXiv preprint
arXiv:1711.00165.
Lu, S., Nott, B., Olson, A., Todeschini, A., Vahabi, H., Car-
mon, Y., and Schmidt, L. (2020). Harder or different?
a closer look at distribution shift in dataset reproduc-
tion. In ICML Workshop on Uncertainty and Robust-
ness in Deep Learning.
Mei, S. and Montanari, A. (2019). The generalization er-
ror of random features regression: Precise asymp-
totics and double descent curve. arXiv preprint
arXiv:1908.05355.
Miller, J. P., Taori, R., Raghunathan, A., Sagawa, S.,
Koh, P. W., Shankar, V., Liang, P., Carmon, Y., and
Schmidt, L. (2021). Accuracy on the line: on the
strong correlation between out-of-distribution and in-
distribution generalization. In International Confer-
ence on Machine Learning, pages 7721–7735. PMLR.
Nakkiran, P., Kaplun, G., Bansal, Y., Yang, T., Barak, B.,
and Sutskever, I. (2019). Deep double descent: Where
bigger models and more data hurt. arXiv preprint
arXiv:1912.02292.
Opper, M., Kinzel, W., Kleinz, J., and Nehl, R. (1990).
On the ability of the optimal perceptron to gener-
alise. Journal of Physics A: Mathematical and Gen-
eral, 23(11):L581.
Rasmussen, C. E. (2003). Gaussian processes in machine
learning. In Summer school on machine learning,
pages 63–71. Springer.
Rath-Manakidis, P. (2021). Interaction of ensembling and
double descent in deep neural networks. Master’s the-
sis, Cognitive Science, Ruhr University Bochum, Ger-
many.
Recht, B., Roelofs, R., Schmidt, L., and Shankar, V. (2018).
Do CIFAR-10 classifiers generalize to CIFAR-10?
arXiv preprint arXiv:1806.00451.
Russakovsky, O., Deng, J., Su, H., Krause, J., Satheesh, S.,
Ma, S., Huang, Z., Karpathy, A., Khosla, A., Bern-
stein, M., et al. (2015). Imagenet large scale visual
recognition challenge. International journal of com-
puter vision, 115(3):211–252.
Simonyan, K. and Zisserman, A. (2014). Very deep con-
volutional networks for large-scale image recognition.
arXiv preprint arXiv:1409.1556.
Yang, Z., Yu, Y., You, C., Steinhardt, J., and Ma, Y. (2020).
Rethinking bias-variance trade-off for generalization
of neural networks. In International Conference on
Machine Learning, pages 10767–10777. PMLR.
ICPRAM 2022 - 11th International Conference on Pattern Recognition Applications and Methods
40