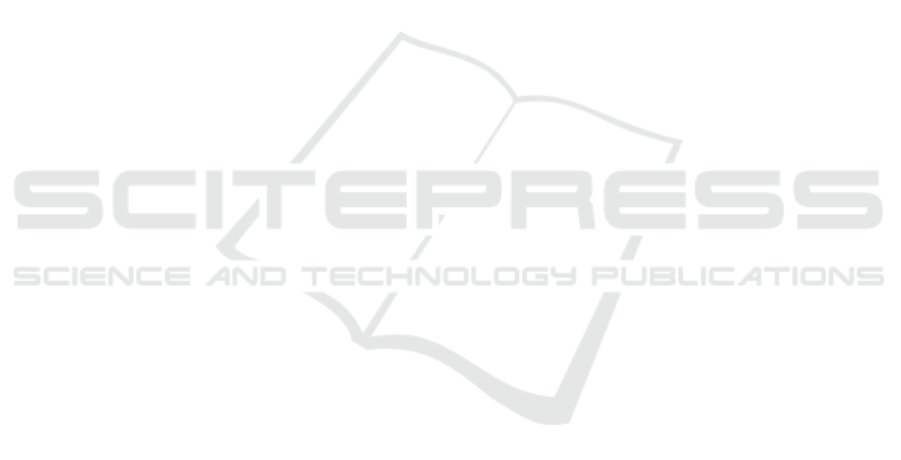
ference on Digital Image Computing: Techniques and
Applications, DICTA 2007, 3-5 December 2007, Ade-
laide, Australia, pages 493–499. IEEE Computer So-
ciety.
He, K., Zhang, X., Ren, S., and Sun, J. (2016). Deep resid-
ual learning for image recognition. In 2016 IEEE Con-
ference on Computer Vision and Pattern Recognition,
CVPR 2016, Las Vegas, NV, USA, June 27-30, 2016,
pages 770–778. IEEE Computer Society.
Jaccard, N., Rogers, T. W., Morton, E. J., and Griffin,
L. D. (2016). Detection of concealed cars in com-
plex cargo x-ray imagery using deep learning. CoRR,
abs/1606.08078.
Kolokytha, S., Flisch, A., L
¨
uthi, T., Plamondon, M., Visser,
W., Schwaninger, A., Hardmeier, D., Costin, M.,
Vienne, C., Sukowski, F., Hassler, U., Dorion, I.,
Gadi, N., Maitrejean, S., Marciano, A., Canonica, A.,
Rochat, E., Koomen, G., and Slegt, M. (2018). Cre-
ating a reference database of cargo inspection x-ray
images using high energy radiographs of cargo mock-
ups. Multim. Tools Appl., 77(8):9379–9391.
Li, B., Zhou, S., Cheng, L., Zhu, R., Hu, T., Anjum, A., He,
Z., and Zou, Y. (2019). A cascade learning approach
for automated detection of locomotive speed sensor
using imbalanced data in ITS. IEEE Access, 7:90851–
90862.
Li, Y., Trinh, H., Haas, N., Otto, C., and Pankanti, S. (2014).
Rail component detection, optimization, and assess-
ment for automatic rail track inspection. IEEE Trans.
Intell. Transp. Syst., 15(2):760–770.
Liu, L., Zhou, F., and He, Y. (2016). Automated visual
inspection system for bogie block key under com-
plex freight train environment. IEEE Trans. Instrum.
Meas., 65(1):2–14.
Liu, Z., Wang, Z., and Xing, Y. (2019). Wagon num-
ber recognition based on the yolov3 detector. In
2019 IEEE 2nd International Conference on Com-
puter and Communication Engineering Technology
(CCET), pages 159–163. IEEE.
Ningning, L., Yuehui, M., Wang, Y., and Wang, N. (2016).
An algorithm of freight train number locating based
on template matching and morphological operations.
Metallurgical and mining industry, (2):102–107.
Otte, T., Bartels, H., Posada Moreno, A. F., Wittenburg, G.,
and Haßler, M. (2020). Holistic data infrastructure
and analytics system for rail freight transport. In 14th
ITS European Congress. 14th ITS European Congress
via 1st Virtual ITS European Congress, online, 9 Nov
2020 - 10 Nov 2020.
Pahwa, R. S., Chandrasekhar, V. R., Chao, J., Paul, J., Li,
Y., Nwe, M. T. L., Xie, S., James, A., Ambikapathi,
A., and Zeng, Z. (2019). Faultnet: Faulty rail-valves
detection using deep learning and computer vision. In
2019 IEEE Intelligent Transportation Systems Confer-
ence, ITSC 2019, Auckland, New Zealand, October
27-30, 2019, pages 559–566. IEEE.
Peng, Z., Wang, C., Ma, Z., and Liu, H. (2020). A mul-
tifeature hierarchical locating algorithm for hexagon
nut of railway fasteners. IEEE Trans. Instrum. Meas.,
69(3):693–699.
Posada Moreno, A., Klein, C., Haßler, M., Pehar, D.,
Solvay, A., and Kohlschein, C. (2020). Cargo wagon
structural health estimation using computer vision. 8th
Transport Research Arena, TRA2020, pages 04–27.
Ren, S., He, K., Girshick, R. B., and Sun, J. (2015). Faster
R-CNN: towards real-time object detection with re-
gion proposal networks. In Cortes, C., Lawrence,
N. D., Lee, D. D., Sugiyama, M., and Garnett, R.,
editors, Advances in Neural Information Processing
Systems 28: Annual Conference on Neural Informa-
tion Processing Systems 2015, December 7-12, 2015,
Montreal, Quebec, Canada, pages 91–99.
Rocha, R. L., Siravenha, A. C. Q., Gomes, A. C. S., Serejo,
G. L., Silva, A. F. B., Rodrigues, L. M., Braga, J.,
Dias, G., Carvalho, S. R., and de Souza, C. R. B.
(2018). A deep-learning-based approach for auto-
mated wagon component inspection. In Haddad,
H. M., Wainwright, R. L., and Chbeir, R., editors, Pro-
ceedings of the 33rd Annual ACM Symposium on Ap-
plied Computing, SAC 2018, Pau, France, April 09-
13, 2018, pages 276–283. ACM.
Rogers, T. W., Jaccard, N., and Griffin, L. D. (2017). A
deep learning framework for the automated inspec-
tion of complex dual-energy x-ray cargo imagery. In
Anomaly Detection and Imaging with X-Rays (ADIX)
II, volume 10187, page 101870L. International Soci-
ety for Optics and Photonics.
Rogers, T. W., Jaccard, N., Morton, E., and Griffin, L.
(2015). Detection of cargo container loads from x-
ray images. In 2nd IET International Conference on
Intelligent Signal Processing 2015 (ISP), pages 1–6.
IET.
Rong, J., Song, S., Dang, Z., Shi, H., and Cao, Y. (2016).
Rail track irregularity detection method based on com-
puter vision and gesture analysis. Int. J. Online Eng.,
12(12):55–59.
Shang, L., Yang, Q., Wang, J., Li, S., and Lei, W. (2018).
Detection of rail surface defects based on cnn image
recognition and classification. In 2018 20th Interna-
tional Conference on Advanced Communication Tech-
nology (ICACT), pages 45–51. IEEE.
Sun, X., Ding, J., Chiara, G. D., Cheah, L., and Cheung,
N. (2017). A generic framework for monitoring lo-
cal freight traffic movements using computer vision-
based techniques. In 5th IEEE International Con-
ference on Models and Technologies for Intelligent
Transportation Systems, MT-ITS 2017, Naples, Italy,
June 26-28, 2017, pages 63–68. IEEE.
Tuszynski, J., Briggs, J. T., and Kaufhold, J. (2013). A
method for automatic manifest verification of con-
tainer cargo using radiography images. Journal of
Transportation Security, 6(4):339–356.
Union Internationale des Chemins de fer (2020). uic work
programme 2020-2022. Technical report.
Union Internationale des Chemins de fer (2021). Vademe-
cum (List of UIC members).
Visser, W., Schwaninger, A., Hardmeier, D., Flisch, A.,
Costin, M., Vienne, C., Sukowski, F., Hassler, U., Do-
rion, I., Marciano, A., Koomen, G., Slegt, M., and
Canonica, A. C. (2016). Automated comparison of x-
ray images for cargo scanning. In IEEE International
ICPRAM 2022 - 11th International Conference on Pattern Recognition Applications and Methods
430