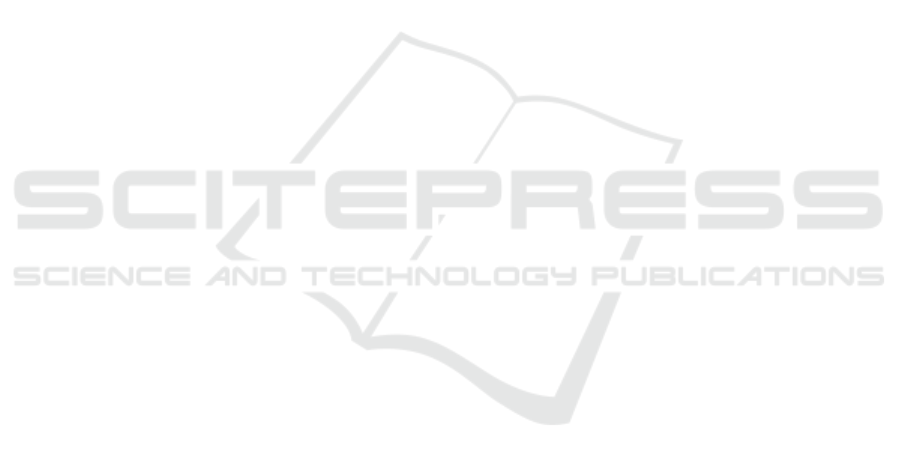
as Discriminators Rather Than Generators. arXiv
preprint arXiv:2003.10555.
Ková
ˇ
r, V., Horák, A., and Jakubí
ˇ
cek, M. (2011). Syn-
tactic Analysis Using Finite Patterns: A New Pars-
ing System for Czech. In Human Language Technol-
ogy. Challenges for Computer Science and Linguis-
tics, pages 161–171, Berlin/Heidelberg. Springer.
Lai, G., Xie, Q., Liu, H., Yang, Y., and Hovy, E.
(2017). RACE: Large-scale ReAding Comprehen-
sion Dataset From Examinations. arXiv preprint
arXiv:1704.04683.
Lan, Z., Chen, M., Goodman, S., Gimpel, K., Sharma,
P., and Soricut, R. (2019). ALBERT: A Lite BERT
for Self-supervised Learning of Language Represen-
tations. arXiv preprint arXiv:1909.11942.
Medved’, M., Sabol, R., and Horák, A. (2020). Efficient
management and optimization of very large machine
learning dataset for question answering. In Horák,
A., editor, Proceedings of the Fourteenth Workshop on
Recent Advances in Slavonic Natural Language Pro-
cessing, RASLAN 2020, pages 23–34, Brno. Tribun
EU.
Medved’, M., Sabol, R., and Horák, A. (2020). Employ-
ing Sentence Context in Czech Answer Selection. In
International Conference on Text, Speech, and Dia-
logue, TSD 2020, pages pp. 112–121. Springer.
Medved’, M. and Horák, A. (2016). AQA: Automatic Ques-
tion Answering System for Czech. In Text, Speech,
and Dialogue, TSD 2016, pages 270–278, Switzer-
land. Springer.
Medved’, M. and Horák, A. (2018). Sentence and word
embedding employed in open question-answering. In
Proceedings of the 10th International Conference on
Agents and Artificial Intelligence (ICAART 2018),
pages 486–492, Setúbal, Portugal. SCITEPRESS -
Science and Technology Publications.
Medved’, M., Sabol, R., and Horák, A. (2020). Improv-
ing RNN-based Answer Selection for Morphologi-
cally Rich Languages. In Proceedings of the 12th
International Conference on Agents and Artificial In-
telligence (ICAART 2020), pages 644–651, Valleta,
Malta. SCITEPRESS - Science and Technology Pub-
lications.
Rajpurkar, P., Zhang, J., Lopyrev, K., and Liang, P. (2016).
SQuAD: 100, 000+ Questions for Machine Compre-
hension of Text. CoRR, abs/1606.05250.
Reimers, N. and Gurevych, I. (2019). Sentence-BERT: Sen-
tence Embeddings using Siamese BERT-Networks. In
Proceedings of the 2019 Conference on Empirical
Methods in Natural Language Processing. Associa-
tion for Computational Linguistics.
Sabol, R., Medved’, M., and Horák, A. (2019). Czech Ques-
tion Answering with Extended SQAD v3.0 Bench-
mark Dataset. In Proceedings of Recent Advances
in Slavonic Natural Language Processing, RASLAN
2019, pages 99–108.
Santos, C. d., Tan, M., Xiang, B., and Zhou, B.
(2016). Attentive pooling networks. arXiv preprint
arXiv:1602.03609.
Sido, J., Pražák, O., P
ˇ
ribá
ˇ
n, P., Pašek, J., Seják, M.,
and Konopík, M. (2021). Czert – Czech BERT-like
Model for Language Representation. arXiv preprint
arXiv:2103.13031.
Straka, M., Náplava, J., Straková, J., and Samuel, D. (2021).
RobeCzech base. LINDAT/CLARIAH-CZ digital li-
brary at the Institute of Formal and Applied Linguis-
tics (ÚFAL), Faculty of Mathematics and Physics,
Charles University.
Warstadt, A., Singh, A., and Bowman, S. R. (2019). Neu-
ral network acceptability judgments. Transactions of
the Association for Computational Linguistics, 7:625–
641.
ICAART 2022 - 14th International Conference on Agents and Artificial Intelligence
394