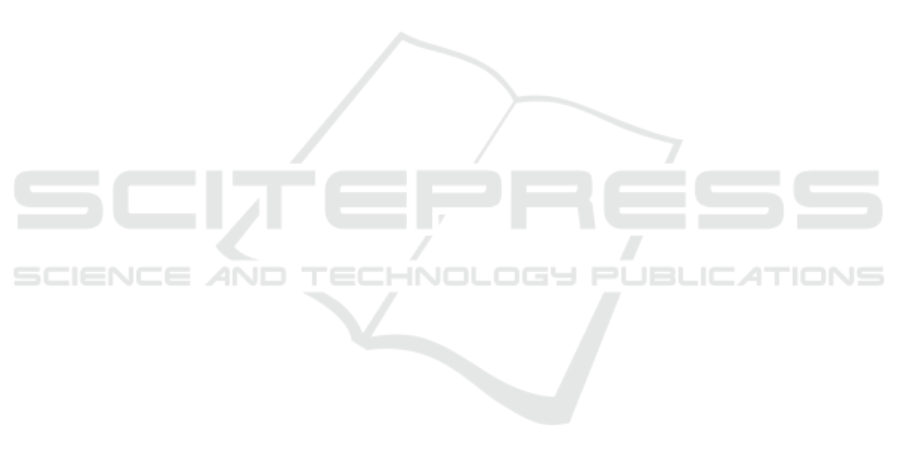
Hadji Misheva, B., Hirsa, A., Osterrieder, J., Kulkarni, O.,
and Fung Lin, S. (2021). Explainable ai in credit
risk management. Credit Risk Management (March
1, 2021).
Helal, S. (2016). Subgroup discovery algorithms: a survey
and empirical evaluation. Journal of Computer Sci-
ence and Technology, 31(3):561–576.
Herrera, F., Carmona, C. J., Gonz
´
alez, P., and Del Jesus,
M. J. (2011). An overview on subgroup discovery:
foundations and applications. Knowledge and infor-
mation systems, 29(3):495–525.
Hind, M., Wei, D., Campbell, M., Codella, N. C., Dhurand-
har, A., Mojsilovi
´
c, A., Natesan Ramamurthy, K., and
Varshney, K. R. (2019). Ted: Teaching ai to explain
its decisions. In Proceedings of the 2019 AAAI/ACM
Conference on AI, Ethics, and Society, pages 123–
129.
Hornik, K., Gr
¨
un, B., and Hahsler, M. (2005). arules-a com-
putational environment for mining association rules
and frequent item sets. Journal of Statistical Software,
14(15):1–25.
Imparato, A. (2013). Interactive subgroup discovery. Mas-
ter’s thesis, Universit
`
a degli studi di Padova.
Kl
¨
osgen, W. (1996). Explora: A multipattern and multi-
strategy discovery assistant. In Advances in Knowl-
edge Discovery and Data Mining, pages 249–271.
Kodinariya, T. M. and Makwana, P. R. (2013). Review on
determining number of cluster in k-means clustering.
International Journal, 1(6):90–95.
Kuzlu, M., Cali, U., Sharma, V., and G
¨
uler,
¨
O. (2020).
Gaining insight into solar photovoltaic power gener-
ation forecasting utilizing explainable artificial intelli-
gence tools. IEEE Access, 8:187814–187823.
Lavra
ˇ
c, N., Cestnik, B., Gamberger, D., and Flach, P.
(2004). Decision support through subgroup discov-
ery: three case studies and the lessons learned. Ma-
chine Learning, 57(1):115–143.
Lemmerich, F., Becker, M., and Puppe, F. (2013).
Difference-based estimates for generalization-aware
subgroup discovery. In ECML PKDD, pages 288–303.
Springer.
Lin, C.-F. (2018). Application-grounded evaluation of pre-
dictive model explanation methods. Master’s thesis,
Eindhoven University of Technology.
Lundberg, S. M. and Lee, S.-I. (2017). A unified approach
to interpreting model predictions. In Proceedings of
the 31st International Conference on Neural Informa-
tion Processing Systems, NIPS’17, page 4768–4777.
McKinsey (2019). Driving impact at scale from automation
and AI. White paper, McKinsey. Online; accessed
October 2021.
Mokhtari, K. E., Higdon, B. P., and Bas¸ar, A. (2019). In-
terpreting financial time series with shap values. In
Proceedings of the 29th Annual International Confer-
ence on Computer Science and Software Engineering,
pages 166–172.
Molnar, C. (2020). Interpretable machine learning. Lulu.
com.
Mueller, S. T., Hoffman, R. R., Clancey, W., Emrey, A., and
Klein, G. (2019). Explanation in human-ai systems: A
literature meta-review, synopsis of key ideas and pub-
lications, and bibliography for explainable ai. arXiv
preprint arXiv:1902.01876.
Pascual, A., Marchini, K., and Van Dyke, A. (2015). Over-
coming False Positives: Saving the Sale and the Cus-
tomer Relationship. White paper, Javelin strategy and
research reports. Online; accessed October 2021.
Quigley, J. and Walls, L. (2007). Trading reliability targets
within a supply chain using shapley’s value. Reliabil-
ity Engineering & System safety, 92(10):1448–1457.
Rai, A. (2020). Explainable AI: From black box to glass
box. Journal of the Academy of Marketing Science,
48(1):137–141.
Ribeiro, M. T., Singh, S., and Guestrin, C. (2016). Why
should I trust you?: Explaining the predictions of any
classifier. In ACM SIGKDD, pages 1135–1144.
Rosenberg, A. and Hirschberg, J. (2007). V-measure: A
conditional entropy-based external cluster evaluation
measure. In EMNLP-CoNLL, pages 410–420.
Shachar, N., Mitelpunkt, A., Kozlovski, T., Galili, T.,
Frostig, T., Brill, B., Marcus-Kalish, M., and Ben-
jamini, Y. (2018). The importance of nonlinear trans-
formations use in medical data analysis. JMIR medi-
cal informatics, 6(2):e27.
Shapley, L. S. (1953). A value for n-person games. Contri-
butions to the Theory of Games, 2(28):307–317.
Sheng, H., Shi, H., et al. (2016). Research on cost allocation
model of telecom infrastructure co-construction based
on value shapley algorithm. International Journal of
Future Generation Communication and Networking,
9(7):165–172.
Song, C., Liu, F., Huang, Y., Wang, L., and Tan, T. (2013).
Auto-encoder based data clustering. In Iberoameri-
can congress on pattern recognition, pages 117–124.
Springer.
Tuff
´
ery, S. (2011). Data mining and statistics for decision
making. John Wiley & Sons.
Utt, J., Springorum, S., K
¨
oper, M., and Im Walde, S. S.
(2014). Fuzzy v-measure-an evaluation method for
cluster analyses of ambiguous data. In LREC, pages
581–587.
Veiber, L., Allix, K., Arslan, Y., Bissyand
´
e, T. F., and Klein,
J. (2020). Challenges towards production-ready ex-
plainable machine learning. In {USENIX} Conference
on Operational Machine Learning (OpML 20).
Vinh, N. X., Epps, J., and Bailey, J. (2010). Information the-
oretic measures for clusterings comparison: Variants,
properties, normalization and correction for chance.
The Journal of Machine Learning Research, 11:2837–
2854.
Wedge, R., Kanter, J. M., Veeramachaneni, K., Rubio,
S. M., and Perez, S. I. (2018). Solving the false posi-
tives problem in fraud prediction using automated fea-
ture engineering. In ECML PKDD, pages 372–388.
Weerts, H. J. (2019). Interpretable machine learning as de-
cision support for processing fraud alerts. Master’s
thesis, Eindhoven University of Technology.
Weerts, H. J., van Ipenburg, W., and Pechenizkiy, M.
(2019). A human-grounded evaluation of shap for
alert processing. In KDD workshop on Explainable
AI.
ICAART 2022 - 14th International Conference on Agents and Artificial Intelligence
402