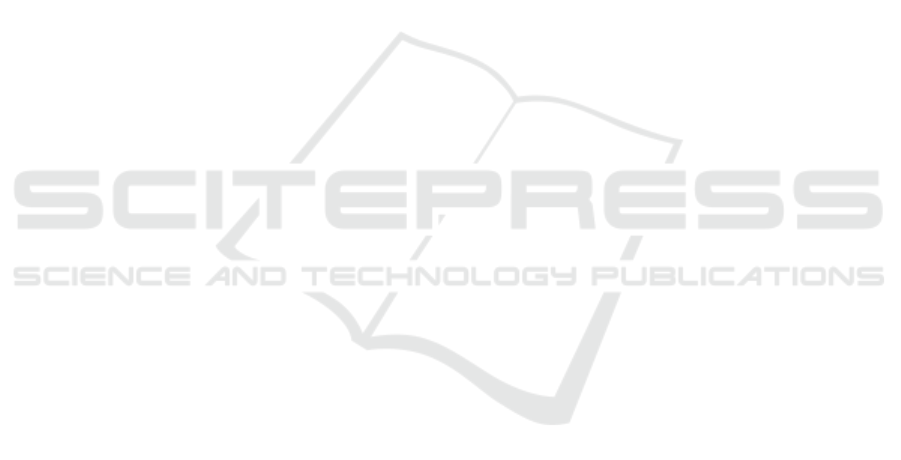
However, we have a 4-period waiting for maintain-
ing s2c3. With the fact that its maintenance occupies
2 periods, we know that s2c3 cannot start the main-
tenance in period 4 since s3c3 is being maintained
during periods 4 and 5, and there is no enough re-
source to maintain s2c3 and s3c3 together in period 5
(n
2
+ n
3
> N
5
). Besides, s2c3 cannot start the main-
tenance in period 6 due to lack of available resources
in period 7 (n
2
> N
7
). Finally, its maintenance is con-
ducted in periods 8 and 9 since n
2
< N
8
and n
2
< N
9
.
5 CONCLUSIONS AND
PERSPECTIVES
In this paper, we addressed RUL-based maintenance
optimization in generic complex production systems.
Component-level RUL information was used to ar-
range redundancy in each stage to guarantee the avail-
ability of the system. Besides, resource limitation
constraints were integrated with respect to real-life
applications and scenarios. The purpose is to sat-
isfy client demands with minimum overall cost dur-
ing the maintenance planning horizon. We provided a
mixed-integer linear programming approach to cope
with problem instances. Through different test in-
stances, we showed the efficiency of our approach to
reach the optimal solutions of the addressed problems
in different complex systems.
Our future work will focus on (i) considering
setup cost when activating standby components; (ii)
Considering the probabilities or quantiles of the RUL
of component; (iii) Developing more efficient algo-
rithms and heuristics; (iv) Taking multi-site mainte-
nance optimization into account.
ACKNOWLEDGEMENTS
This work is supported by the project Maintenance
Pr
´
evisionelle et Optimisation of IRT SystemX.
REFERENCES
https://www.ibm.com/docs/en/icos/12.7.1.0?topic=smippqt-
miqcp-mixed-integer-programs-quadratic-terms-in-
constraints. MIQCP: mixed integer programs with
quadratic terms in the constraints.
Bahria, N., Chelbi, A., Bouchriha, H., and Dridi, I. H.
(2019). Integrated production, statistical process con-
trol, and maintenance policy for unreliable manufac-
turing systems. International Journal of Production
Research 57(8): 2548-2570.
Camci, F. (2009). System maintenance scheduling with
prognostics information using genetic algorithm.
IEEE Transactions on Reliability 58(3): 539-552.
Camci, F., Medjaher, K., Atamuradov, V., and Berdinya-
zov, A. (2019). Integrated maintenance and mission
planning using remaining useful life information. En-
gineering optimization 51(10): 1794-1809.
Chen, Z., Li, Y., Xia, T., and Pan, E. (2019). Hidden Markov
model with auto-correlated observations for remain-
ing useful life prediction and optimal maintenance
policy. Reliability Engineering & System Safety 184:
123-136.
De Jonge, B. and Scarf, P. A. (2020). A review on mainte-
nance optimization. European Journal of Operational
Research 285(3): 805-824.
Dong, W., Liu, S., Cao, Y., Javed, S. A., and Du, Y. (2020).
Reliability modeling and optimal random preventive
maintenance policy for parallel systems with dam-
age self-healing. Computers & Industrial Engineering
142: 106359.
Do, P., Voisin, A., Levrat, E., and Lung, B. (2015). A proac-
tive condition-based maintenance strategy with both
perfect and imperfect maintenance actions. Reliability
Engineering & System Safety 133: 22-32.
Lei, Y., Li, N., Guo, L., Li, N., Yan, T., and Lin, J. (2018).
Machinery health prognostics: A systematic review
from data acquisition to RUL prediction. Mechanical
Systems and Signal Processing 104: 799-834.
Lei, X. and Sandborn, P. A. (2018). Maintenance scheduling
based on remaining useful life predictions for wind
farms managed using power purchase agreements. Re-
newable Energy 116: 188-198.
Rivera-G
´
omez, H., Gharbi, A., Kenn
´
e, J. P., Monta ˜no-
Arango, O., and Corona-Armenta, J. R. (2020). Joint
optimization of production and maintenance strategies
considering a dynamic sampling strategy for a deteri-
orating system. Computers & Industrial Engineering
140: 106273.
Si, X. S., Wang, W., Hu, C. H., and Zhou, D. H. (2011).
Remaining useful life estimation - a review on the sta-
tistical data driven approaches. European Journal of
Operations Research 213: 1-14.
Wu, S. and Castro, I. T. (2020). Maintenance policy for a
system with a weighted linear combination of degra-
dation processes. European Journal of Operational
Research 280(1): 124-133.
Xenos, D. P., Kopanos, G. M., Cicciotti, M., and Thornhilla,
N. F. (2016). Operational optimization of networks
of compressors considering condition-based mainte-
nance. Computers & Chemical Engineering 84: 117-
131.
Ye, Y., Grossmann, I. E., Pinto, J. M., and Ramaswamy,
S. (2019). Modeling for reliability optimization of
system design and maintenance based on Markov
chain theory. Computers & Chemical Engineering
124: 381-404.
Zhou, H., Wang, S., Qi, F., and Gao, S. (2019). Maintenance
modeling and operation parameters optimization for
complex production line under reliability constraints.
Annals of Operations Research 1-17.
Zhu, Z., Xiang, Y., and Zeng, B. (2021). Multicomponent
maintenance optimization: a stochastic programming
approach. INFORMS Journal on Computing 33(3):
898-914.
ICORES 2022 - 11th International Conference on Operations Research and Enterprise Systems
176