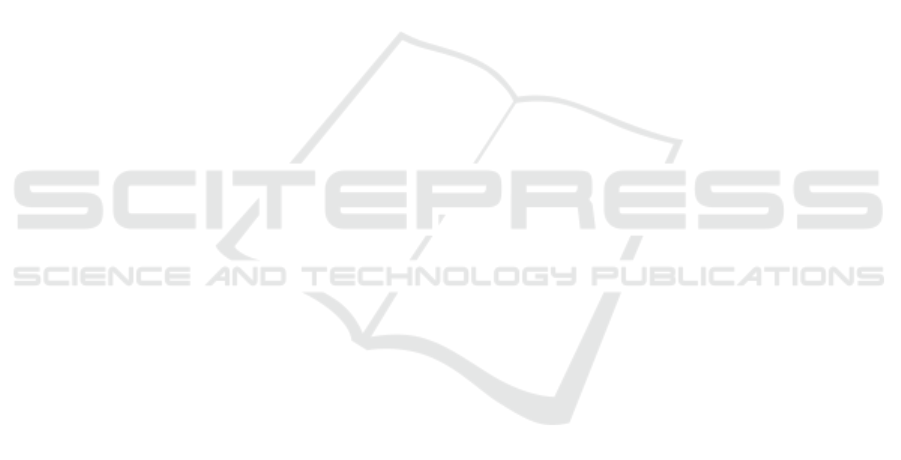
REFERENCES
Agogino, A. K. and Tumer, K. (2012). A multiagent ap-
proach to managing air traffic flow. Autonomous
Agents and Multi-Agent Systems, 24(1):1–25.
Alam, S., Shafi, K., Abbass, H. A., and Barlow, M. (2009).
An ensemble approach for conflict detection in free
flight by data mining. Transportation research part
C: emerging technologies, 17(3):298–317.
Alonso-Ayuso, A., Escudero, L. F., Olaso, P., and Pizarro,
C. (2013). Conflict avoidance: 0-1 linear models for
conflict detection & resolution. Top, 21(3):485–504.
Baker, S. and Korhonen, A.-L. (2017). Initializing neural
networks for hierarchical multi-label text classifica-
tion. Association for Computational Linguistics.
Bayen, A., Grieder, P., Meyer, G., and Tomlin, C. J. (2005).
Langrangian delay predictive model for sector-based
air traffic flow. Journal of guidance, control, and dy-
namics, 28(5):1015–1026.
Bergstra, J. and Bengio, Y. (2012). Random search for
hyper-parameter optimization. The Journal of Ma-
chine Learning Research, 13(1):281–305.
Brittain, M. and Wei, P. (2018). Autonomous aircraft se-
quencing and separation with hierarchical deep rein-
forcement learning. In Proceedings of the Interna-
tional Conference for Research in Air Transportation.
Eby, M. S. and Kelly, W. E. (1999). Free flight separa-
tion assurance using distributed algorithms. In 1999
IEEE Aerospace Conference. Proceedings (Cat. No.
99TH8403), volume 2, pages 429–441. IEEE.
Erzberger, H., Paielli, R. A., Isaacson, D. R., and Eshow,
M. M. (1997). Conflict detection and resolution in the
presence of prediction error. In 1st USA/Europe Air
Traffic Management R&D Seminar, Saclay, France,
pages 17–20. Citeseer.
Gardner, M. W. and Dorling, S. (1998). Artificial neural
networks (the multilayer perceptron)—a review of ap-
plications in the atmospheric sciences. Atmospheric
environment, 32(14-15):2627–2636.
He, H. and Xia, R. (2018). Joint binary neural network
for multi-label learning with applications to emotion
classification. In CCF International Conference on
Natural Language Processing and Chinese Comput-
ing, pages 250–259. Springer.
Hoekstra, J. M. and Ellerbroek, J. (2016). Bluesky atc
simulator project: an open data and open source ap-
proach. In Proceedings of the 7th International Con-
ference on Research in Air Transportation, pages 1–8.
FAA/Eurocontrol USA/Europe.
Jiang, X.-r., Wen, X.-x., Wu, M.-g., Wang, Z.-k., and Qiu,
X. (2018). A svm approach of aircraft conflict detec-
tion in free flight. J. of Advanced Transportation.
Kim, K., Hwang, I., and Yang, B.-J. (2016). Classifica-
tion of conflict resolution methods using data-mining
techniques. In 16th AIAA Aviation Technology, Inte-
gration, and Operations Conference, page 4075.
Kohavi, R. et al. (1995). A study of cross-validation and
bootstrap for accuracy estimation and model selec-
tion. In Ijcai, volume 14, pages 1137–1145. Montreal,
Canada.
Kuchar, J. K. and Yang, L. C. (2000). A review of
conflict detection and resolution modeling methods.
IEEE Transactions on intelligent transportation sys-
tems, 1(4):179–189.
Lapasset, L., Rahman, M. S., and Mothe, J. (2020). Solv-
ing aircraft conflicts: data resources. In 1st Interna-
tional Conference on Cognitive Aircraft Systems (IC-
CAS 2020), page 76.
Nanduri, A. and Sherry, L. (2016). Anomaly detection
in aircraft data using recurrent neural networks (rnn).
In 2016 Integrated Communications Navigation and
Surveillance (ICNS), pages 5C2–1. IEEE.
Paielli, R. A. and Erzberger, H. (1997). Conflict probability
estimation for free flight. Journal of Guidance, Con-
trol, and Dynamics, 20(3):588–596.
Panchal, G., Ganatra, A., Kosta, Y., and Panchal, D. (2011).
Behaviour analysis of multilayer perceptrons with
multiple hidden neurons and hidden layers. Interna-
tional Journal of Computer Theory and Engineering,
3(2):332–337.
Pavlinovi
´
c, M., Boras, D., and Franceti
´
c, I. (2013). First
steps in designing air traffic control communication
language technology system-compiling spoken corpus
of radiotelephony communication. International Jour-
nal of Computers and Communications, 7(3):73.
Pham, D.-T., Tran, N. P., Alam, S., Duong, V., and Dela-
haye, D. (2019a). A machine learning approach for
conflict resolution in dense traffic scenarios with un-
certainties.
Pham, D.-T., Trant, N. P., Goh, S. K., Alam, S., and
Duong, V. (2019b). Reinforcement learning for two-
aircraft conflict resolution in the presence of uncer-
tainty. In 2019 IEEE-RIVF International Confer-
ence on Computing and Communication Technologies
(RIVF), pages 1–6. IEEE.
Prandini, M., Hu, J., Lygeros, J., and Sastry, S. (2000).
A probabilistic approach to aircraft conflict detection.
IEEE Transactions on intelligent transportation sys-
tems, 1(4):199–220.
Prandini, M., Lygeros, J., Nilim, A., and Sastry, S. (1999).
A probabilistic framework for aircraft conflict detec-
tion. In Guidance, Navigation, and Control Confer-
ence and Exhibit, page 4144.
Rahman, M. S. (2020). Supervised machine learning model
to help controllers solving aircraft conflicts. In AD-
BIS, TPDL and EDA 2020 Common Workshops and
Doctoral Consortium, pages 355–361. Springer.
Sch
¨
afer, M., Strohmeier, M., Lenders, V., Martinovic, I.,
and Wilhelm, M. (2014). Bringing up opensky: A
large-scale ads-b sensor network for research. In Pro-
ceedings of the 13th international symposium on In-
formation processing in sensor networks, pages 83–
94. IEEE Press.
Srinivasamurthy, A., Motlicek, P., Singh, M., Oualil, Y.,
Kleinert, M., Ehr, H., and Helmke, H. (2018). It-
erative learning of speech recognition models for air
traffic control. In Interspeech, pages 3519–3523.
Tsoumakas, G. and Katakis, I. (2007). Multi-label classi-
fication: An overview. International Journal of Data
Warehousing and Mining (IJDWM), 3(3):1–13.
ICAART 2022 - 14th International Conference on Agents and Artificial Intelligence
410