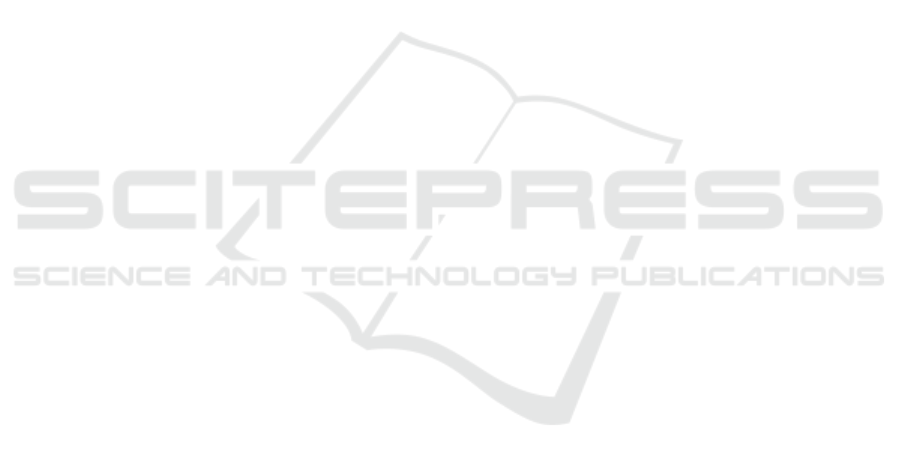
that interact with one another via an exchange, how-
ever in our research the exchange is not a financial
exchange, but is instead a betting exchange. Betting
exchanges, an innovation from the dot-com boom of
the late 1990s, have been a massive disruptor of the
global betting/bookmaking industry over the past two
decades. The primary innovator in this space was
the British company called Betfair, whose founders
recognised that just as a financial exchange acts as
a platform that enables traders to seek and identify
potential counter-parties to trade with (i.e., the ex-
change matches buyers to sellers, and sellers to buy-
ers), so the same technology could be used to enable
bettors to seek and identify potential counter-parties
to a bet (i.e., the exchange matches bettors who want
to bet that some event E will happen, to bettors who
want to bet that E will not happen). The development
of high-technology online betting exchanges enabled
further innovation, introducing a new type of betting
that would have been difficult or impracticable to im-
plement without computerised technology: specifi-
cally, betting exchanges developed the capability to
offer so-called in-play (or in-game or in-race) betting,
where bettors can continue to bet on the outcome of
an event such as a horse-race after it has started, with
betting continuing potentially right up until the mo-
ment that the event ends and the winner is known.
As with the work of Lomas & Cliff, we use estab-
lished methods from the OD literature to give change-
able opinions to the agents in our ABM, but our
agents are opinionated bettors rather than Lomas &
Cliff’s opinionated traders. To do this, we have used
a newly-developed ABM of a betting exchange with
in-play betting on track-racing events such as horse-
races or sports-car races. This model is the open-
source Bristol Betting Exchange (BBE) described by
(Cliff, 2021; Cliff et al., 2021), various implementa-
tions of which are freely available on GitHub. BBE
offers a minimal abstract simulation of a track-race
event, sufficient to make the dynamics of the simu-
lated betting exchange interestingly realistic, and in-
cludes a base set of types of different betting strate-
gies: bettor-agents can each be instantiated with one
of these strategies, which vary in their degree of ra-
tionality and in their accuracy of predicting the final
outcome of the race.
The behavior of human bettors, as individuals and
in aggregate (i.e., as populations of bettors) has long
been studied by economists and psychologists inter-
ested in how we make economic decisions in situa-
tions of risk and uncertainty. An extensive literature
survey is presented in (Cliff, 2021) which reveals that,
to the best of our knowledge, the work we report here
is the first ever ABM exploration of narrative eco-
nomics and opinion dynamics in a betting context.
In our model, over the duration of any one experi-
ment, each agent interacts with some number of other
agents in the population, with each atomic event being
a pairwise interaction between two agents. When two
agents (denoted here as A1 and A2) interact, the opin-
ion of A1 might be altered in response to the opin-
ion of A2, and/or the opinion of A2 might alter in re-
sponse to the opinion of A1. Whether the opinions of
A1 and/or A2 alter at all, and how much they change
by if they do, depends on the particular OD model in
use. This approach, of agents privately communicat-
ing with one another in pairs and their opinions pos-
sibly altering in response, is entirely standard within
the OD literature: indeed, in the vast majority of OD
papers, that is all that is studied. The link between
this mainstream style of OD and narrative economics
was established by (Lomas and Cliff, 2021) to which
the reader is referred for further details. Because in
this paper we move beyond the mainstream OD ap-
proach, we will denote this aspect of the OD model in
our system as the local opinions for each bettor: that
is, this influence on the bettor’s opinion comes from
local interactions with other bettors. However, in our
model this is not the only influence.
In real life, we might meet a friend for a chat over
morning coffee, and our friend might tell us her hot tip
for which horse is going to win a race tomorrow; in
the course of the rest of the day, we might meet other
people and pass on our friend’s tip to them: in the
course of these interactions, peoples’ opinions about
the outcome of tomorrow’s race are altered via their
private local interactions. However, surely this is not
the only factor of significance. There are two other
additional factors that we model here.
The first additional factor is one that we refer to
as the global opinion available to an individual bettor.
In contrast to tips passed among friends, anyone who
looks at the betting exchange’s real-time information
about the market for tomorrow’s horse-race can read-
ily see the aggregated opinions of very large numbers
of bettors, each of whom is sufficiently sure of their
opinions that they have put money down, i.e. paid a
stake for their bet into the exchange, in the expecta-
tion that their personal opinion is correct. In this way,
an individual bettor’s opinion can be influenced by the
combined opinions of everyone who has placed a bet
in the exchange’s market for that event, i.e. the over-
all market sentiment. If our friend’s hot tip is that
horse H1 will win the race, but currently the vast ma-
jority of bets, or of total money wagered, is indicating
a common belief that a different horse H2 will instead
win, we may well come to doubt the wisdom of our
tipster friend, and so in this way our opinion can be
ICAART 2022 - 14th International Conference on Agents and Artificial Intelligence
226