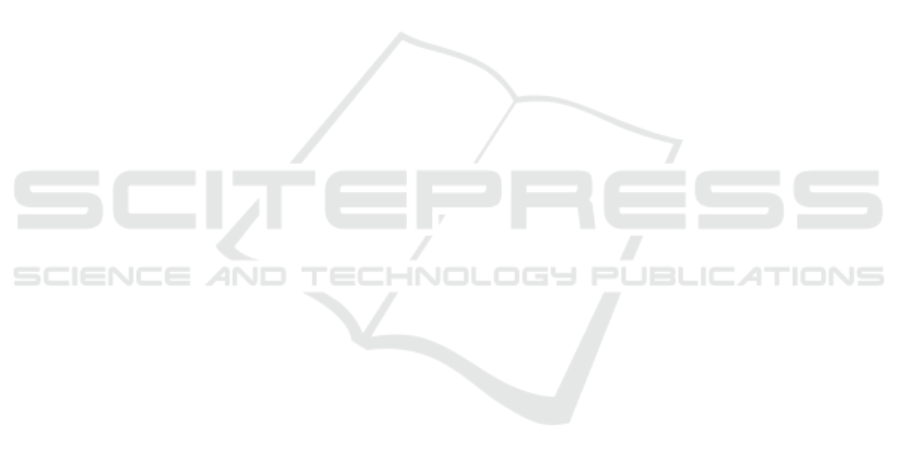
respect to a given normative system, and assigning
punishments to a MORL agent simultaneously learn-
ing to achieve ethical and non-ethical objectives.
NGRL offers more versatility with respect to the
complexity of the norms to be adhered to, than di-
rectly assigning rewards to specific events or with re-
spect to simple constraints. It may be the case that
there is no obvious or coherent way to summarize an
entire normative system by selecting specific events
and assigning punishments to them. By using NGRL,
we expand what kinds of normatively compliant be-
haviour we can learn, and are allowed to specify them
in a more natural way.
Our experimental results showed that NGRL was
effective in producing an agent that learned to avoid
most violations – even in a stochastic environment –
while still pursuing its non-ethical goal. However,
these results also revealed that we achieve optimal
results when we use NGRL in conjunction with the
normative supervisor as originally intended, as a real-
time compliance-checker. NGRL allows us to circum-
vent the weaknesses of the normative supervision ap-
proach – namely, its inability to preemptively avoid
violations – while normative supervision allows us to
maintain a better guarantee of compliance.
As discussed in Sect. 3.3.1, NGRL can be further
developed in its handling of normative conflict and
contrary-to-duty obligations. Moreover, as this ap-
proach applies only to MORL variants of Q-learning,
it will fall prey to the same scaling issues. Adapting
NGRL to be used with Q-learning with function ap-
proximation, for example, will broaden the domains
to which NGRL can applied.
REFERENCES
Abel, D., MacGlashan, J., and Littman, M. L. (2016). Rein-
forcement learning as a framework for ethical decision
making. In AAAI Workshop: AI, Ethics, and Society,
volume 16.
Alshiekh, M., Bloem, R., Ehlers, R., K
¨
onighofer, B.,
Niekum, S., and Topcu, U. (2018). Safe reinforcement
learning via shielding. In Proceedings of the AAAI
Conference on Artificial Intelligence, volume 32.
Balakrishnan, A., Bouneffouf, D., Mattei, N., and Rossi, F.
(2019). Incorporating behavioral constraints in online
AI systems. In Proceedings of the AAAI Conference
on Artificial Intelligence, volume 33, pages 3–11.
Barrett, L. and Narayanan, S. (2008). Learning all opti-
mal policies with multiple criteria. In Proceedings of
the 25th international conference on Machine learn-
ing, pages 41–47.
Boella, G. and van der Torre, L. (2004). Regulative and
constitutive norms in normative multiagent systems.
In Proc. of KR 2004: the 9th International Confer-
ence on Principles of Knowledge Representation and
Reasoning, pages 255–266. AAAI Press.
Broersen, J. M., Cranefield, S., Elrakaiby, Y., Gabbay,
D. M., Grossi, D., Lorini, E., Parent, X., van der Torre,
L. W. N., Tummolini, L., Turrini, P., and Schwarzen-
truber, F. (2013). Normative reasoning and con-
sequence. In Normative Multi-Agent Systems, vol-
ume 4 of Dagstuhl Follow-Ups, pages 33–70. Schloss
Dagstuhl - Leibniz-Zentrum f
¨
ur Informatik.
Chisholm, R. M. (1963). Contrary-to-duty imperatives and
deontic logic. Analysis, 24(2):33–36.
G
´
abor, Z., Kalm
´
ar, Z., and Szepesv
´
ari, C. (1998). Multi-
criteria reinforcement learning. In Proceedings of
the Fifteenth International Conference on Machine
Learning., volume 98, pages 197–205.
Governatori, G. (2018). Practical normative reasoning with
defeasible deontic logic. In Reasoning Web Interna-
tional Summer School, pages 1–25. Springer.
Governatori, G. and Hashmi, M. (2015). No time for com-
pliance. In IEEE 19th International Enterprise Dis-
tributed Object Computing Conference, pages 9–18.
IEEE.
Governatori, G., Olivieri, F., Rotolo, A., and Scannapieco,
S. (2013). Computing strong and weak permissions
in defeasible logic. Journal of Philosophical Logic,
42(6):799–829.
Hasanbeig, M., Abate, A., and Kroening, D. (2019).
Logically-constrained neural fitted q-iteration. In Pro-
ceedings of the 18th International Conference on Au-
tonomous Agents and MultiAgent Systems, AAMAS
’19, page 2012–2014.
Hasanbeig, M., Abate, A., and Kroening, D. (2020). Cau-
tious reinforcement learning with logical constraints.
In Proceedings of the 19th International Conference
on Autonomous Agents and Multiagent Systems, AA-
MAS ’20, Auckland, New Zealand, May 9-13, 2020,
pages 483–491.
Hasanbeig, M., Kantaros, Y., Abate, A., Kroening, D., Pap-
pas, G. J., and Lee, I. (2019). Reinforcement learning
for temporal logic control synthesis with probabilistic
satisfaction guarantees. In Proc. of CDC 2019: the
58th IEEE Conference on Decision and Control.
Jansen, N., K
¨
onighofer, B., Junges, S., Serban, A., and
Bloem, R. (2020). Safe Reinforcement Learning Us-
ing Probabilistic Shields (Invited Paper). In 31st Inter-
national Conference on Concurrency Theory (CON-
CUR 2020), volume 171 of Leibniz International Pro-
ceedings in Informatics (LIPIcs), pages 3:1–3:16.
Jones, A. J. I. and Sergot, M. (1996). A Formal Characteri-
sation of Institutionalised Power. Logic Journal of the
IGPL, 4(3):427–443.
Kasenberg, D. and Scheutz, M. (2018). Norm conflict
resolution in stochastic domains. In Proceedings of
the AAAI Conference on Artificial Intelligence, vol-
ume 32.
Lam, H.-P. and Governatori, G. (2009). The making of
SPINdle. In Proc. of RuleML 2009: International
Symposium on Rule Interchange and Applications,
volume 5858 of LNCS, Heidelberg. Springer.
ICAART 2022 - 14th International Conference on Agents and Artificial Intelligence
452