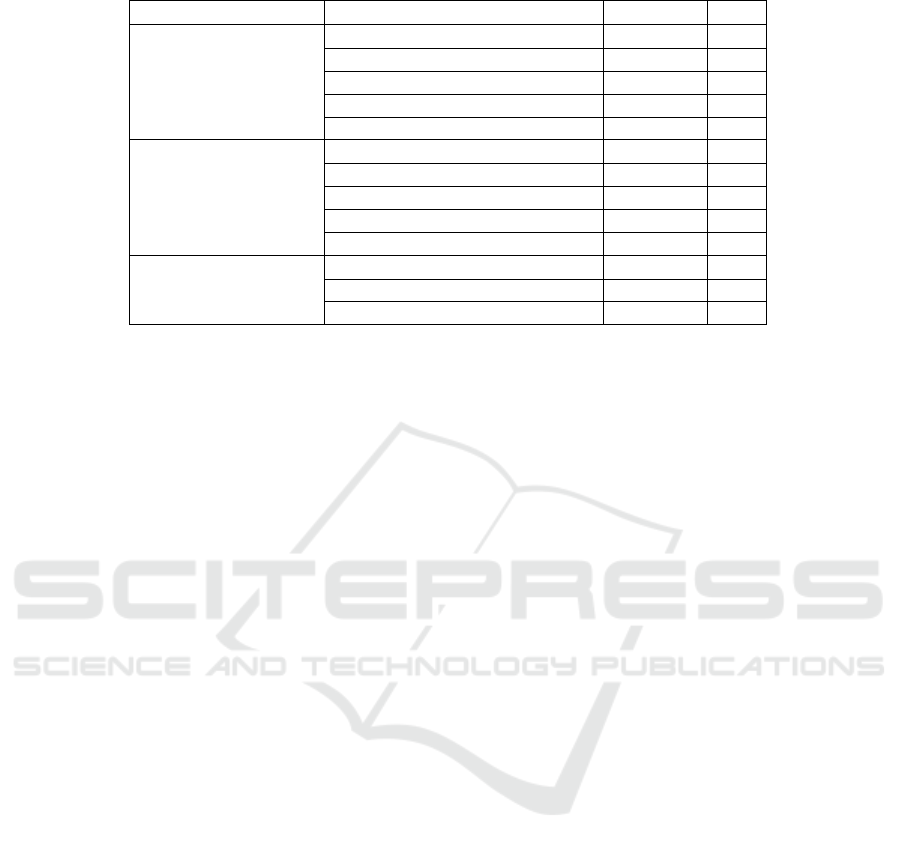
Table 5: A summary of models’ performances in terms of accuracy and F1.
Method Model Accuracy F1
Word-based
SVM 48.41 0.44
NB 50.08 0.46
SWAT 18.73 0.06
ET 43.41 0.34
Our Word-based model 52.59 0.48
Topic-based
ETM 46.21 0.44
ATM 21.16 0.06
SLTM 27.43 0.22
MSTM 19.40 0.06
Our Topic-based model 48.12 0.42
Deep Learning-based
LSTM 45.34 0.41
AttBiLSTM 47.22 0.43
Our Deep learning-based model 55.55 0.49
more advanced models that depend on deep learning-
based methods and neural topic models. On the other
hand, we will attempt to improve the prediction per-
formance for emotions with low precision and recall.
REFERENCES
Alsaedi, A., Brooker, P., Grasso, F., and Thomason, S.
(2021). A survey of social emotion prediction meth-
ods. In Proceedings of the 10th International Confer-
ence on Data Science, Technology and Applications.
SCITEPRESS-Science and Technology Publications.
Alvarez-Melis, D. and Saveski, M. (2016). Topic Modeling
in Twitter: Aggregating Tweets by Conversations. In
Proceedings of the Tenth International AAAI Confer-
ence onWeb and Social Media.
Baker, C. F., Fillmore, C. J., and Lowe, J. B. (1998). The
berkeley framenet project. In 36th Annual Meeting
of the Association for Computational Linguistics and
17th International Conference on Computational Lin-
guistics, Volume 1, pages 86–90.
Bao, S., Xu, S., Zhang, L., Yan, R., Su, Z., Han, D., and
Yu, Y. (2009). Joint emotion-topic modeling for so-
cial affective text mining. In Proceedings - IEEE In-
ternational Conference on Data Mining, ICDM, pages
699–704. IEEE.
Bao, S., Xu, S., Zhang, L., Yan, R., Su, Z., Han, D., and
Yu, Y. (2011). Mining Social Emotions from Affec-
tive Text. IEEE Transactions on Knowledge and Data
Engineering, 24(9):1658–1670.
Blei, D. M. and McAuliffe, J. D. (2010). Supervised topic
models. arXiv preprint arXiv:1003.0783.
Blei, D. M., Ng, A. Y., and Jordan, M. I. (2003). Latent
dirichlet allocation. Journal of machine Learning re-
search, 3(Jan):993–1022.
Bostan, L., Kim, E., and Klinger, R. (2019). GoodNew-
sEveryone: A Corpus of News Headlines Annotated
with Emotions, Semantic Roles, and Reader Percep-
tion. arXiv:1912.03184v3 [cs.CL].
Buechel, S. and Hahn, U. (2017). EMOBANK: Studying
the impact of annotation perspective and representa-
tion format on dimensional emotion analysis. In 15th
Conference of the European Chapter of the Associa-
tion for Computational Linguistics, EACL 2017, vol-
ume 2, pages 578–585.
Chaumartin, F. R. (2007). UPAR7: A knowledge-based
system for headline sentiment tagging. In Agirre, E.,
M
`
arquez, L., and Wicentowski, R., editors, Proceed-
ings of the 4th International Workshop on Semantic
Evaluations (SemEval-2007), pages 422–425. ACL.
Fan, W. and Gordon, M. D. (2014). The power of social me-
dia analytics. Communications of the ACM, 57(6):74–
81.
Guan, X., Peng, Q., Li, X., and Zhu, Z. (2019). Social
Emotion Prediction with Attention-based Hierarchical
Neural Network. In 2019 IEEE 4th Advanced Infor-
mation Technology, Electronic and Automation Con-
trol Conference (IAEAC), pages 1001–1005.
Hochreiter, S. and Schmidhuber, J. (1997). Long short-term
memory. Neural Comput., 9(8):1735–1780.
Katz, P., Singleton, M., and Wicentowski, R. (2007).
SWAT-MP: The SemEval-2007 systems for task 5 and
task 14. In ACL 2007 - SemEval 2007 - Proceedings
of the 4th International Workshop on Semantic Evalu-
ations, pages 308–313.
Kingma, D. P. and Ba, J. (2015). Adam: A Method for
Stochastic Optimization. In Bengio, Y. and LeCun,
Y., editors, 3rd International Conference on Learn-
ing Representations, ICLR 2015, San Diego, CA, USA,
May 7-9, 2015, Conference Track Proceedings.
Krebs, F., Lubascher, B., Moers, T., Schaap, P., and
Spanakis, G. (2018). Social emotion mining
techniques for facebook posts reaction prediction.
ICAART 2018 - Proceedings of the 10th Interna-
tional Conference on Agents and Artificial Intelli-
gence, 2:211–220.
Lerner, J. S. and Keltner, D. (2000). Beyond valence: To-
ward a model of emotion-specific influences on judge-
ment and choice. Cognition & emotion, 14(4):473–
493.
Improving Social Emotion Prediction with Reader Comments Integration
291