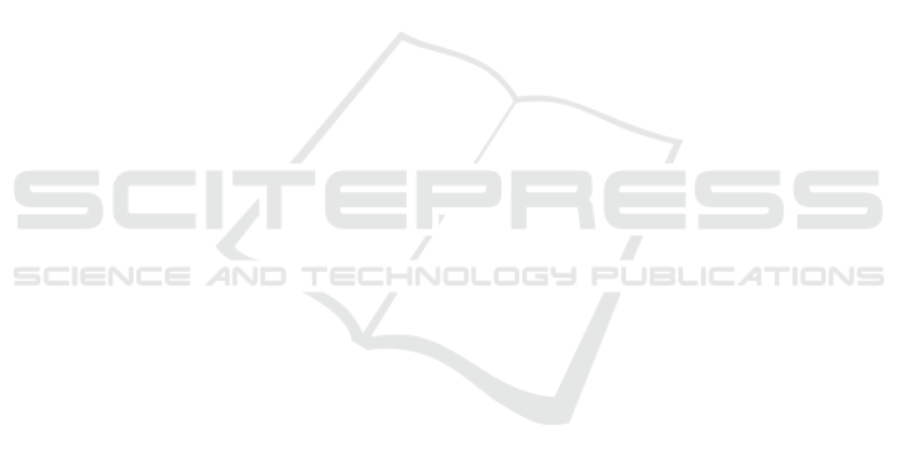
coordination and waiting times. Management Science,
65(5):1969–1994.
El-Bouri, R., Taylor, T., Youssef, A., Zhu, T., and Clifton,
D. A. (2021). Machine learning in patient flow: a re-
view. Progress in Biomedical Engineering.
Fatma, N., Mohd, S., Ramamohan, V., and Mustafee, N.
(2020). Primary healthcare delivery network simu-
lation using stochastic metamodels. In 2020 Winter
Simulation Conference (WSC), pages 818–829. IEEE.
Fatma, N. and Ramamohan, V. (2021a). Patient diversion
across primary health centers using real time delay
predictors. In 2021 Institute of Industrial and Systems
Engineers (IISE) Annual Conference & Expo.
Fatma, N. and Ramamohan, V. (2021b). Patient diversion
using real-time delay prediction across healthcare fa-
cility networks. http://web.iitd.ac.in/
∼
mez188287/NF.
pdf, accessed 29
th
November 2021.
Guti
´
errez, J. M. P., Sicilia, M.-
´
A., Sanchez-Alonso, S.,
and Garc
´
ıa-Barriocanal, E. (2021). Predicting length
of stay across hospital departments. IEEE Access,
9:44671–44680.
Hijry, H. and Olawoyin, R. (2020). Application of ma-
chine learning algorithms for patient length of stay
prediction in emergency department during hajj. In
2020 IEEE International Conference on Prognostics
and Health Management (ICPHM), pages 1–8. IEEE.
Hopp, W. J. and Spearman, M. L. (2011). Factory physics.
Waveland Press.
Ibrahim, R. (2018). Sharing delay information in ser-
vice systems: a literature survey. Queueing Systems,
89(1):49–79.
IPHS-Guidelines (2012). Guidelines for Primary Health
Centres. Directorate General of Health Services, New
Delhi, India.
Li, M., Vanberkel, P., and Carter, A. J. (2019). A review
on ambulance offload delay literature. Health Care
Management Science, 22(4):658–675.
Liu, Y., Zhong, L., Yuan, S., and van de Klundert, J. (2018).
Why patients prefer high-level healthcare facilities: a
qualitative study using focus groups in rural and urban
china. BMJ Global Health, 3(5):e000854.
Marquinez, J. T., Saur
´
e, A., Cataldo, A., and Ferrer, J.-C.
(2021). Identifying proactive icu patient admission,
transfer and diversion policies in a public-private hos-
pital network. European Journal of Operational Re-
search.
Morley, C., Unwin, M., Peterson, G. M., Stankovich, J., and
Kinsman, L. (2018). Emergency department crowd-
ing: a systematic review of causes, consequences and
solutions. PloS One, 13(8):e0203316.
Mustafee, N. and Powell, J. (2020). Providing real-time
information for urgent care. Impact, 2021(1):25–29.
Nezamoddini, N. and Khasawneh, M. T. (2016). Model-
ing and optimization of resources in multi-emergency
department settings with patient transfer. Operations
Research for Health Care, 10:23–34.
Piermarini, C. and Roma, M. (2021). A simulation-based
optimization approach for analyzing the ambulance
diversion phenomenon in an emergency department
network. arXiv preprint arXiv:2108.04162.
Ramirez-Nafarrate, A., Fowler, J. W., and Wu, T. (2011).
Design of centralized ambulance diversion policies
using simulation-optimization. In Proceedings of
the 2011 Winter Simulation Conference (WSC), pages
1251–1262. IEEE.
Rao, K. D. and Sheffel, A. (2018). Quality of clinical care
and bypassing of primary health centers in india. So-
cial Science & Medicine, 207:80–88.
Shaaban, A. N., Peleteiro, B., and Martins, M. R. O. (2021).
Statistical models for analyzing count data: predictors
of length of stay among hiv patients in portugal using
a multilevel model. BMC Health Services Research,
21(1):1–17.
Sharma, R., Prakash, A., Chauhan, R., and Dhibar, D. P.
(2021). Overcrowding an encumbrance for an emer-
gency health-care system: A perspective of health-
care providers from tertiary care center in northern in-
dia. Journal of Education and Health Promotion, 10.
Shi, P., Helm, J. E., Deglise-Hawkinson, J., and Pan, J.
(2021). Timing it right: Balancing inpatient conges-
tion vs. readmission risk at discharge. Operations Re-
search.
Shoaib, M. and Ramamohan, V. (2021). Simulation mod-
elling and analysis of primary health centre opera-
tions. arXiv preprint arXiv:2104.12492.
Song, H., Tucker, A. L., and Murrell, K. L. (2015). The
diseconomies of queue pooling: An empirical investi-
gation of emergency department length of stay. Man-
agement Science, 61(12):3032–3053.
Turgeman, L., May, J. H., and Sciulli, R. (2017). Insights
from a machine learning model for predicting the hos-
pital length of stay (los) at the time of admission. Ex-
pert Systems with Applications, 78:376–385.
van der Ham, R. (2018). salabim: discrete event simula-
tion and animation in python. Journal of Open Source
Software, 3(27):767.
Verburg, I. W., de Keizer, N. F., de Jonge, E., and Peek,
N. (2014). Comparison of regression methods for
modeling intensive care length of stay. PloS One,
9(10):e109684.
Wachtel, G. and Elalouf, A. (2020). Addressing overcrowd-
ing in an emergency department: an approach for
identifying and treating influential factors and a real-
life application. Israel Journal of Health Policy Re-
search, 9(1):1–12.
Whiteside, T., Kane, E., Aljohani, B., Alsamman, M., and
Pourmand, A. (2020). Redesigning emergency depart-
ment operations amidst a viral pandemic. The Amer-
ican Journal of Emergency Medicine, 38(7):1448–
1453.
Whitt, W. (1999). Predicting queueing delays. Management
Science, 45(6):870–888.
Zhou, Q., Fan, L., Lai, X., Tan, L., and Zhang, X. (2019).
Estimating extra length of stay and risk factors of mor-
tality attributable to healthcare-associated infection at
a chinese university hospital: a multi-state model.
BMC Infectious Diseases, 19(1):1–7.
ICORES 2022 - 11th International Conference on Operations Research and Enterprise Systems
66