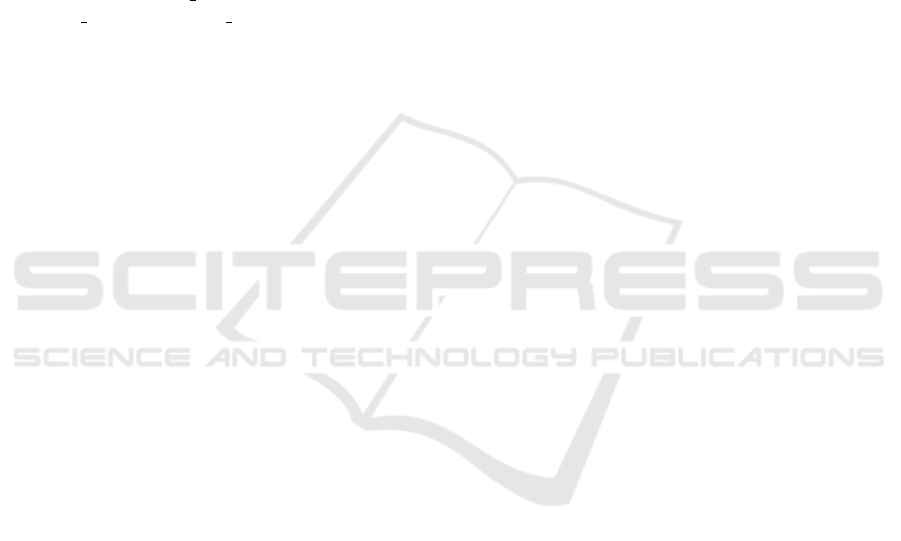
reach a more optimal outcome more rapidly without re-
ducing safety and incentive-compatibility. Regarding
the impact of adaptive coefficient
β
, we can observe
that a tiny non-null coefficient is sufficient to make the
agent safer and more incentive compatible.
7.3 Results Summary
Although our GTFT is not perfectly optimal in am-
biguous situations (with multiple optimal cycles), we
can conclude that the key point of our experiments is
the importance of a graph-processing algorithm. The
min-cost max flow is the best approach since it is
more incentive-compatible. Regarding the choice of
TFT function, the TFT beta function is clearly safer
than TFT alpha while TFT gamma is slightly more
efficient.
8 CONCLUSION
In this paper, we introduced a novel paradigm for the
N
-player Prisoner’s Dilemma where maximal coopera-
tion between agents is induced by a weighted directed
graph. This new model is particularly suited to address
the asymmetry of cooperation and in particular the cir-
cular social dilemmas: a specific situation of dilemma
where players can form a cycle of cooperation in which
no player can cooperate with its ”helper”. We showed
that classic solutions like Tit-for-Tat strategies can-
not solve properly this specific issue, we therefore
also proposed in the paper a Graph-based Tit-for-Tat
which generalizes the classic TFT with a flow network
approach. We evaluated this new algorithm in some
scenarios and compare it to some baselines. As ma-
jor conclusions, we can observe that adding a graph
processing in the TFT is relevant since our GTFT out-
performs the original TFT in most of situations. As
further works, it could be very interesting to address
the ambiguous cases with multiple equivalent optimal
cycles.
We recall our main contributions:
•
We introduced and formalized a novel Graph-
based Iterated Prisoner’s Dilemma: a formalism
able to generalize the
N
-player IPD involving
asymmetrical or circular cooperation.
•
We designed and formalized several social metrics
adapted to this GIPD.
•
We constructed a novel Graph-based Tit-for-Tat
able to cope with circular cooperation, it is based
on continuous TFT and max-flow algorithms.
We are convinced that this new GTFT paradigm
which solves circular dilemmas should offer a lot of
perspectives particularly in addition to the recent tech-
niques mixing RL and TFT. Finally, in view of the
expectations regarding the digital sobriety and the eth-
ical stakes of artificial intelligence, we reiterated the
importance of focusing urgently on non-cooperative
games, and striving to include this kind of paradigm
in the design of our future intelligent systems.
REFERENCES
Agudo, J. E. and Fyfe, C. (2011). Reinforcement learning
for the n-persons iterated prisoners’ dilemma. In 2011
Seventh International Conference on Computational
Intelligence and Security, pages 472–476. IEEE.
Ashlock, D. A. (2007). Cooperation in prisoner’s dilemma
on graphs. In 2007 IEEE Symposium on Computational
Intelligence and Games, pages 48–55. IEEE.
Axelrod, R. and Hamilton, W. D. (1981). The evolution of
cooperation. science, 211(4489):1390–1396.
Beaufils, B., Delahaye, J.-P., Mathieu, P., et al. (2001).
Adaptive behaviour in the classical iterated prisoner’s
dilemma. In Proc. Artificial Intelligence & Simul. Be-
haviour Symp. on Adaptive Agents & Multi-Agent Sys-
tems. Citeseer.
Flood, M. M. (1958). Some experimental games. Manage-
ment Science, 5(1):5–26.
Ford, L. R. and Fulkerson, D. R. (1956). Maximal flow
through a network. Canadian journal of Mathematics,
8:399–404.
Ford Jr, L. R. (1956). Network flow theory. Technical report,
Rand Corp Santa Monica Ca.
Hager, G. D., Drobnis, A., Fang, F., Ghani, R., Greenwald,
A., Lyons, T., Parkes, D. C., Schultz, J., Saria, S.,
Smith, S. F., et al. (2019). Artificial intelligence for
social good. arXiv preprint arXiv:1901.05406.
Hamburger, H. (1973). N-person prisoner’s dilemma. Jour-
nal of Mathematical Sociology, 3(1):27–48.
Izquierdo, S. S., Izquierdo, L. R., and Gotts, N. M. (2008).
Reinforcement learning dynamics in social dilemmas.
Journal of Artificial Societies and Social Simulation,
11(2):1.
Jaques, N., Lazaridou, A., Hughes, E., Gulcehre, C., Ortega,
P., Strouse, D., Leibo, J. Z., and De Freitas, N. (2019).
Social influence as intrinsic motivation for multi-agent
deep reinforcement learning. In International Confer-
ence on Machine Learning, pages 3040–3049. PMLR.
Le Gl
´
eau, T., Marjou, X., Lemlouma, T., and Radier, B.
(2020). Game theory approach in multi-agent resources
sharing. In 25th IEEE Symposium on Computers and
Communications (ISCC).
Leibo, J. Z., Zambaldi, V., Lanctot, M., et al. (2017). Multi-
agent reinforcement learning in sequential social dilem-
mas. In Proceedings of the 16th Conference on Au-
tonomous Agents and MultiAgent Systems, pages 464–
473.
Lerer, A. and Peysakhovich, A. (2017). Maintaining cooper-
ation in complex social dilemmas using deep reinforce-
ment learning. arXiv preprint arXiv:1707.01068.
Towards Circular and Asymmetric Cooperation in a Multi-player Graph-based Iterated Prisoner’s Dilemma
301