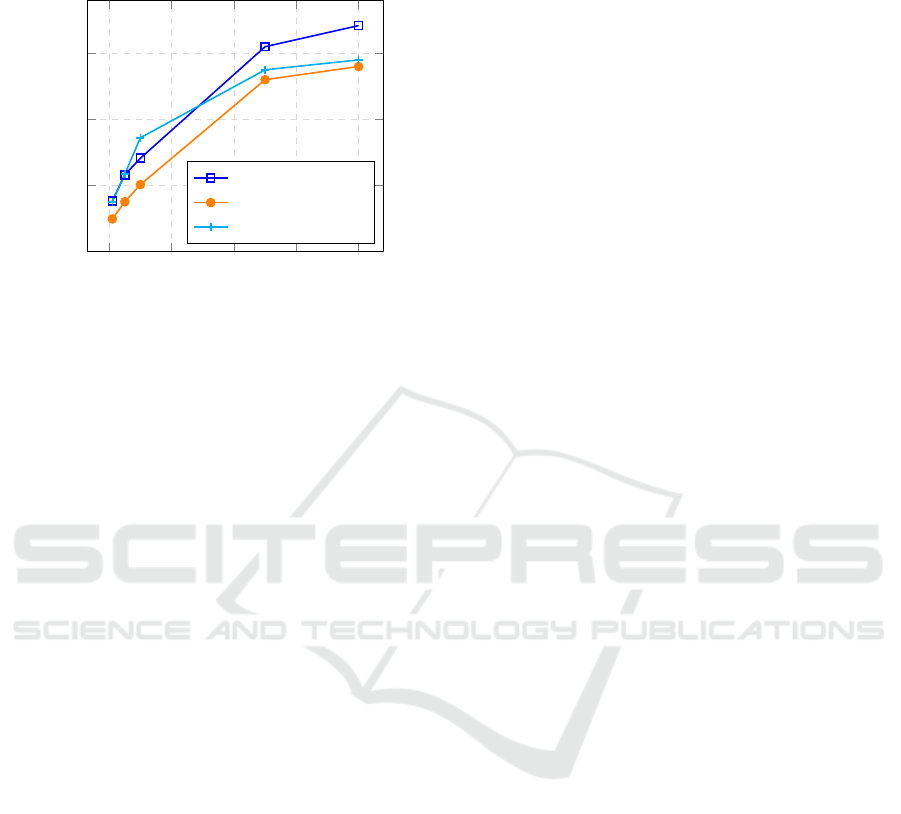
respectively about ∼19% and ∼16% faster than the
only use of HC.
0 2 4
6
8
·10
4
0
10
20
30
Sample size
Expected Loss
HC
HC, sc=1, k=0.2
HC, sc=2, k=0.2
Figure 6: Runtime w.r.t. the data size.
6 CONCLUSION AND FUTURE
WORKS
In this paper, we proposed a new generic method for
the BN learning structure by exploiting the knowl-
edge in the ontology. Our method is divided into sev-
eral steps: (i) constructing a weighted graph from the
ontology (ii) deriving dependencies and constructing
the BN skeleton (iii) orienting edges using the seman-
tic of relations in the ontology (iv) refining the BN via
a scored based algorithm. By integrating knowledge
from the ontology, our approach leads to a significant
improvement in terms of runtime computations and
expected loss results. The proposed approach can be
easily applied to other domains. As future works, our
current approach can be extended by integrating addi-
tional knowledge from ontologies and also by refining
these latter through the use of BN results (adding re-
lationships, uncertainty, etc.). Additional experimen-
tations on more complex real-world datasets will be
also explored.
REFERENCES
Bucci, G., Sandrucci, V., and Vicario, E. (2011). Ontologies
and bayesian networks in medical diagnosis. In 2011
44th Hawaii International Conference on System Sci-
ences, pages 1–8. IEEE.
Carvalho, R. N., Laskey, K. B., and Costa, P. C. (2010).
Pr-owl 2.0–bridging the gap to owl semantics. Uncer-
tainty reasoning for the semantic web II, pages 1–18.
Devitt, A., Danev, B., and Matusikova, K. (2006). Con-
structing bayesian networks automatically using on-
tologies. Applied Ontology, 1.
Dijkstra, E. W. et al. (1959). A note on two problems
in connexion with graphs. Numerische mathematik,
1(1):269–271.
Fenz, S. (2012). An ontology-based approach for construct-
ing bayesian networks. Data & Knowledge Engineer-
ing, 73:73–88.
Genesereth, M. R. and Nilsson, N. J. (1987). Logical Foun-
dations of Artificial Intelligence. San Francisco, CA,
USA.
Gruber, T. R. (1993). A translation approach to portable
ontology specifications. Knowledge Acquisition,
5:199–220.
Guarino, N. (1995). Formal ontology, conceptual analysis
and knowledge representation. International Journal
Human Computer Study, 43(5-6):625–640.
Helsper, E. M. and Van Der Gaag, L. C. (2002). Building
bayesian networks through ontologies. In ECAI, vol-
ume 2002, page 15th.
Ishak, M. B., Leray, P., and Amor, N. B. (2011a). Ontology-
based generation of object oriented bayesian net-
works. In BMAW 2011, pages 9–17.
Ishak, M. B., Leray, P., and Amor, N. B. (2011b). A
two-way approach for probabilistic graphical models
structure learning and ontology enrichment. In KEOD
2011, pages 189–194.
Jeon, B.-J. and Ko, I.-Y. (2007). Ontology-based semi-
automatic construction of bayesian network models
for diagnosing diseases in e-health applications. In
2007 Frontiers in the Convergence of Bioscience and
Information Technologies, pages 595–602. IEEE.
Messaoud, M. B., Leray, P., and Amor, N. B. (2013). Ac-
tive learning of causal bayesian networks using on-
tologies: a case study. In The 2013 International Joint
Conference on Neural Networks (IJCNN), pages 1–8.
IEEE.
Mohammed, A.-W., Xu, Y., and Liu, M. (2016).
Knowledge-oriented semantics modelling towards un-
certainty reasoning. SpringerPlus, 5(1):1–27.
Pearl, J. (1988). Probabilistic reasoning in intelligent sys-
tems : networks of plausible inference / Judea Pearl.
Morgan Kaufmann Publishers San Mateo, Calif.
Pearl, J. (2014). Probabilistic reasoning in intelligent sys-
tems: networks of plausible inference. Elsevier.
Scutari, M. (2009). Learning bayesian networks with the
bnlearn r package. arXiv preprint arXiv:0908.3817.
Setiawan, F. A., Budiardjo, E. K., and Wibowo, W. C.
(2019). Bynowlife: a novel framework for owl and
bayesian network integration. Information, 10(3):95.
Wang, Y. (2007). Integrating uncertainty into ontology
mapping. In The Semantic Web, pages 961–965.
Springer.
Yang, Y. and Calmet, J. (2005). Ontobayes: An ontology-
driven uncertainty model. In International Conference
on Computational Intelligence for Modelling, Control
and Automation and International Conference on In-
telligent Agents, Web Technologies and Internet Com-
merce (CIMCA-IAWTIC’06), volume 1, pages 457–
463. IEEE.
Zhang, S., Sun, Y., Peng, Y., Wang, X., et al. (2009).
Bayesowl: A prototype system for uncertainty in se-
mantic web. In Proceedings of the International Con-
ference on Artificial Intelligence.
Exploiting Ontology to Build Bayesian Network
585