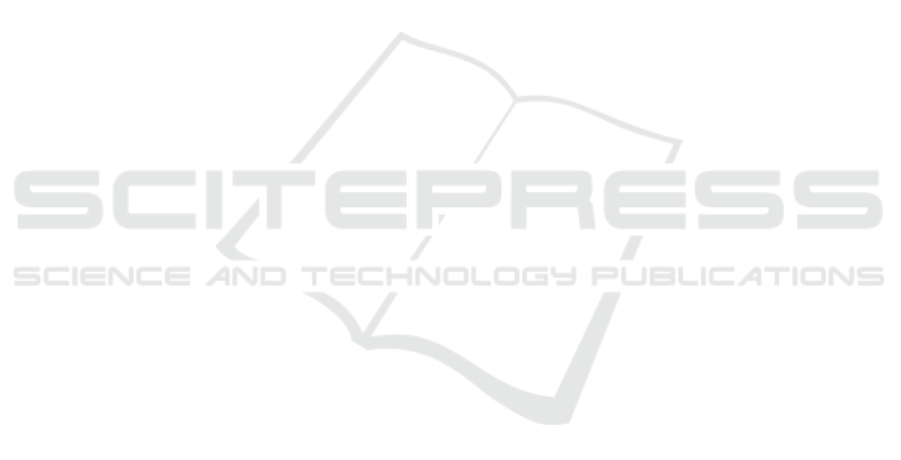
tection from chest x-ray images: A high resolution
network (hrnet) approach.
Albahli, S. (2020). Efficient gan-based chest radiographs
(cxr) augmentation to diagnose coronavirus disease
pneumonia. International journal of medical sciences,
17(10):1439.
Apostolopoulos, I. D. and Mpesiana, T. A. (2020). Covid-
19: automatic detection from x-ray images utilizing
transfer learning with convolutional neural networks.
Physical and Engineering Sciences in Medicine,
43(2):635–640.
Bhagat, V. and Bhaumik, S. (2019). Data augmentation
using generative adversarial networks for pneumonia
classification in chest xrays. In 2019 Fifth Interna-
tional Conference on Image Information Processing
(ICIIP), pages 574–579. IEEE.
Chollet, F. (2017). Xception: Deep learning with depthwise
separable convolutions. In Proceedings of the IEEE
conference on computer vision and pattern recogni-
tion, pages 1251–1258.
Chowdhury, M. E., Rahman, T., Khandakar, A., Mazhar,
R., Kadir, M. A., Mahbub, Z. B., Islam, K. R., Khan,
M. S., Iqbal, A., Al Emadi, N., et al. (2020). Can
ai help in screening viral and covid-19 pneumonia?
IEEE Access, 8:132665–132676.
Das, A. K., Ghosh, S., Thunder, S., Dutta, R., Agarwal,
S., and Chakrabarti, A. (2021). Automatic covid-19
detection from x-ray images using ensemble learning
with convolutional neural network. Pattern Analysis
and Applications, pages 1–14.
El Asnaoui, K. and Chawki, Y. (2020). Using x-ray images
and deep learning for automated detection of coron-
avirus disease. Journal of Biomolecular Structure and
Dynamics, pages 1–12.
Gordienko, Y., Gang, P., Hui, J., Zeng, W., Kochura, Y.,
Alienin, O., Rokovyi, O., and Stirenko, S. (2018).
Deep learning with lung segmentation and bone
shadow exclusion techniques for chest x-ray analy-
sis of lung cancer. In International Conference on
Computer Science, Engineering and Education Appli-
cations, pages 638–647. Springer.
Hira, S., Bai, A., and Hira, S. (2021). An automatic ap-
proach based on cnn architecture to detect covid-19
disease from chest x-ray images. Applied Intelligence,
51(5):2864–2889.
Huang, G., Liu, Z., Van Der Maaten, L., and Weinberger,
K. Q. (2017). Densely connected convolutional net-
works. In Proceedings of the IEEE conference on
computer vision and pattern recognition, pages 4700–
4708.
Irvin, J., Rajpurkar, P., Ko, M., Yu, Y., Ciurea-Ilcus, S.,
Chute, C., Marklund, H., Haghgoo, B., Ball, R., Sh-
panskaya, K., et al. (2019). Chexpert: A large chest
radiograph dataset with uncertainty labels and expert
comparison. In Proceedings of the AAAI Conference
on Artificial Intelligence, volume 33, pages 590–597.
Joseph, M. (2020). Does imagenet pretraining work for
chest radiography images(covid-19)?
Kesim, E., Dokur, Z., and Olmez, T. (2019). X-ray chest im-
age classification by a small-sized convolutional neu-
ral network. In 2019 scientific meeting on electrical-
electronics & biomedical engineering and computer
science (EBBT), pages 1–5. IEEE.
Khan, A. I., Shah, J. L., and Bhat, M. M. (2020). Coronet:
A deep neural network for detection and diagnosis of
covid-19 from chest x-ray images. Computer Methods
and Programs in Biomedicine, 196:105581.
Oh, Y., Park, S., and Ye, J. C. (2020). Deep learning covid-
19 features on cxr using limited training data sets.
IEEE Transactions on Medical Imaging, 39(8):2688–
2700.
Pardamean, B., Cenggoro, T. W., Rahutomo, R., Budiarto,
A., and Karuppiah, E. K. (2018). Transfer learning
from chest x-ray pre-trained convolutional neural net-
work for learning mammogram data. Procedia Com-
puter Science, 135:400–407.
Rahman, T., Khandakar, A., Qiblawey, Y., Tahir, A.,
Kiranyaz, S., Kashem, S. B. A., Islam, M. T.,
Al Maadeed, S., Zughaier, S. M., Khan, M. S.,
et al. (2021). Exploring the effect of image enhance-
ment techniques on covid-19 detection using chest
x-ray images. Computers in biology and medicine,
132:104319.
Santa Cruz, J. F. H. (2021). An ensemble approach
for multi-stage transfer learning models for covid-
19 detection from chest ct scans. Intelligence-Based
Medicine, 5:100027.
Sethi, R., Mehrotra, M., and Sethi, D. (2020). Deep learn-
ing based diagnosis recommendation for covid-19 us-
ing chest x-rays images. In 2020 Second International
Conference on Inventive Research in Computing Ap-
plications (ICIRCA), pages 1–4.
Stirenko, S., Kochura, Y., Alienin, O., Rokovyi, O., Gor-
dienko, Y., Gang, P., and Zeng, W. (2018). Chest x-
ray analysis of tuberculosis by deep learning with seg-
mentation and augmentation. In 2018 IEEE 38th In-
ternational Conference on Electronics and Nanotech-
nology (ELNANO), pages 422–428. IEEE.
Tataru, C., Yi, D., Shenoyas, A., and Ma, A. (2017). Deep
learning for abnormality detection in chest x-ray im-
ages. In IEEE Conference on Deep Learning.
Wang, B., Wu, Z., Khan, Z. U., Liu, C., and Zhu, M.
(2019). Deep convolutional neural network with seg-
mentation techniques for chest x-ray analysis. In 2019
14th IEEE Conference on Industrial Electronics and
Applications (ICIEA), pages 1212–1216. IEEE.
Wang, X., Peng, Y., Lu, L., Lu, Z., Bagheri, M., and Sum-
mers, R. M. (2017). Chestx-ray8: Hospital-scale chest
x-ray database and benchmarks on weakly-supervised
classification and localization of common thorax dis-
eases. In Proceedings of the IEEE conference on
computer vision and pattern recognition, pages 2097–
2106.
Wiysobunri, B. N., Erden, H. S., and Toreyin, B. U. (2020).
An ensemble deep learning system for the automatic
detection of covid-19 in x-ray images.
ICAART 2022 - 14th International Conference on Agents and Artificial Intelligence
386