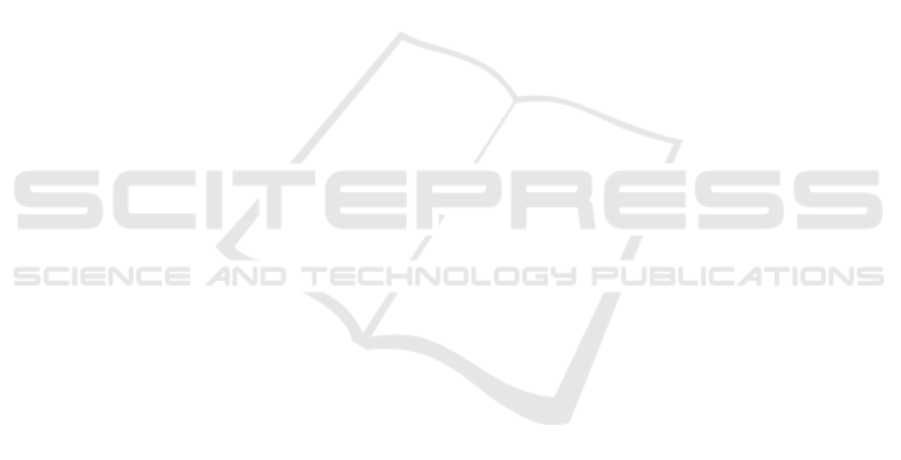
to the PDDL+ language, that does not allow to de-
scribe for instance the characteristics of valid states,
there is also a lack of work in the area from the plan-
ning community, as highlighted by a recent analysis
(Chrpa et al., 2017; Vallati and McCluskey, 2021).
We see several avenues for future work. First, we
are interested in designing approaches to verify com-
plex initial states expressed in PDDL+; this can be
done either by leveraging on additional knowledge
provided as an attachment to a planning model, or
by analysing the characteristics of the domain model
to identify suspicious trajectories. Second, we plan
to extend the capabilities of existing validation ap-
proaches, to provide additional support when plans
are analysed. Finally, we are working on a language
for supporting the goal specification, that allows do-
main experts to express goals in a way that can then
be translated into actual PDDL+ code.
ACKNOWLEDGEMENTS
Mauro Vallati was supported by a UKRI Future Lead-
ers Fellowship [grant number MR/T041196/1].
REFERENCES
Bart
´
ak, R., Fratini, S., and McCluskey, L. (2010). The third
competition on knowledge engineering for planning
and scheduling. AI Mag., 31(1):95–98.
Capitanelli, A., Maratea, M., Mastrogiovanni, F., and Val-
lati, M. (2018). On the manipulation of articulated ob-
jects in human-robot cooperation scenarios. Robotics
and Autonomous Systems, 109:139–155.
Cardellini, M., Maratea, M., Vallati, M., Boleto, G., and
Oneto, L. (2021). In-station train dispatching: A
PDDL+ planning approach. In Proceedings of the
Thirty-First International Conference on Automated
Planning and Scheduling,, pages 450–458. AAAI
Press.
Chrpa, L., McCluskey, T. L., Vallati, M., and Vaquero, T.
(2017). The fifth international competition on knowl-
edge engineering for planning and scheduling: Sum-
mary and trends. AI Mag., 38(1):104–106.
Fox, M. and Long, D. (2003). PDDL2.1: an exten-
sion to PDDL for expressing temporal planning do-
mains. Journal of Artificial Intelligence Research
(JAIR), 20:61–124.
Fox, M. and Long, D. (2006). Modelling mixed discrete-
continuous domains for planning. J. Artif. Intell. Res.,
27:235–297.
Fox, M., Long, D., and Magazzeni, D. (2012). Plan-based
policies for efficient multiple battery load manage-
ment. J. Artif. Intell. Res., 44:335–382.
Ghallab, M., Nau, D., and Traverso, P. (2004). Automated
Planning: theory and practice. Elsevier.
Gregory, P. (2020). PDDL Templating and Custom Report-
ing: Generating Problems and Processing Plans. In
Proc. of KEPS.
Guli
´
c, M., Olivares, R., and Borrajo, D. (2016). Using auto-
mated planning for traffic signals control. PROMET-
Traffic&Transportation, 28(4):383–391.
Hoffmann, J. (2015). Simulated penetration testing: From
”dijkstra” to ”turing test++”. In Proceedings of the
Twenty-Fifth International Conference on Automated
Planning and Scheduling, ICAPS, pages 364–372.
Hounsell, N. and McDonald, M. (1990). Astrid: Automatic
scoot traffic information database. Project report.
Howey, R., Long, D., and Fox, M. (2004). VAL: automatic
plan validation, continuous effects and mixed initia-
tive planning using PDDL. In Proceedings of the 16th
IEEE International Conference on Tools with Artifi-
cial Intelligence (ICTAI), pages 294–301.
Hu, H. and Smith, S. F. (2019). Using bi-directional infor-
mation exchange to improve decentralized schedule-
driven traffic control. In Proceedings of the Twenty-
Ninth International Conference on Automated Plan-
ning and Scheduling, ICAPS, pages 200–208. AAAI
Press.
Kvarnstr
¨
om, J. and Doherty, P. (2010). Automated planning
for collaborative uav systems. In Control Automation
Robotics & Vision (ICARCV), 2010 11th International
Conference on, pages 1078–1085.
Long, D., Dolejsi, J., and Fox, M. (2018). Building Support
for PDDL as a Modelling Tool. In Proc. of KEPS.
Lopez, P. A., Behrisch, M., Bieker-Walz, L., Erdmann, J.,
Fl
¨
otter
¨
od, Y.-P., Hilbrich, R., L
¨
ucken, L., Rummel, J.,
Wagner, P., and Wießner, E. (2018). Microscopic traf-
fic simulation using sumo. In Proceedings of ITSC.
McCluskey, T. L. and Porteous, J. M. (1997). Engineering
and compiling planning domain models to promote
validity and efficiency. Artif. Intell., 95(1):1–65.
McCluskey, T. L. and Vallati, M. (2017). Embedding auto-
mated planning within urban traffic management op-
erations. In Proceedings of the Twenty-Seventh In-
ternational Conference on Automated Planning and
Scheduling, ICAPS, pages 391–399.
McCluskey, T. L., Vaquero, T. S., and Vallati, M. (2017).
Engineering knowledge for automated planning: To-
wards a notion of quality. In Proceedings of the
Knowledge Capture Conference, K-CAP, pages 14:1–
14:8.
Pozanco, A., Fern
´
andez, S., and Borrajo, D. (2021). On-
line modelling and planning for urban traffic control.
Expert Systems.
Scala, E., Haslum, P., Thi
´
ebaux, S., and Ram
´
ırez, M.
(2020). Subgoaling techniques for satisficing and op-
timal numeric planning. J. Artif. Intell. Res., 68:691–
752.
Smith, S. F. (2020). Smart infrastructure for future urban
mobility. AI Mag., 41(1):5–18.
Taale, H., Fransen, W., and Dibbits, J. (1998). The second
assessment of the SCOOT system in Nijmegen. In
IEEE Road Transport Information and Control, num-
ber 21-23.
ICAART 2022 - 14th International Conference on Agents and Artificial Intelligence
396