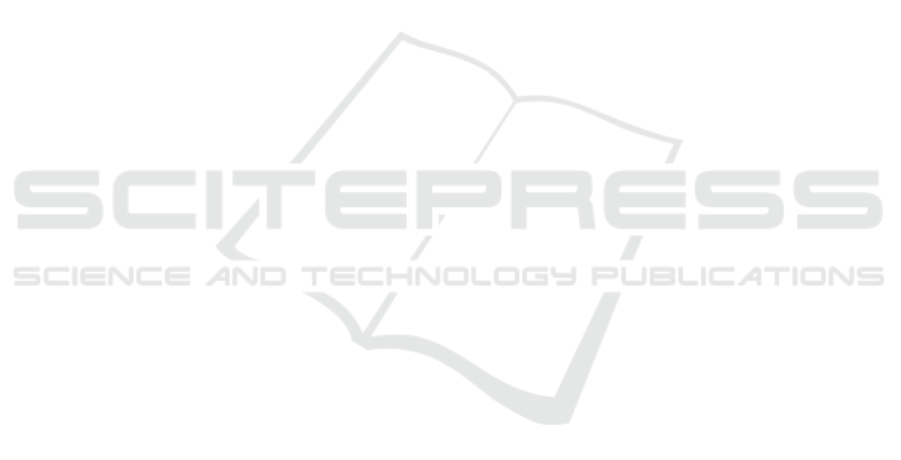
helps optimize event marketing strategies made by
companies after an event and during it.
A next step would be to implement this framework
in a real-world event and compare its performance to
that of a traditional framework. As described earlier,
the approach used in the framework is time-efficient
and can be easily implemented, making it a promising
event managing and marketing tool.
Like any other AI-based framework, it is impor-
tant to address the ethical concerns which accompany
the proposed framework (Liu et al., 2021; Zhu et al.,
2020; Oseni et al., 2021). While such events are gen-
erally public and it is common to have cameras in-
stalled for security purposes, it is still important to
get the approval of attendees when it comes to us-
ing their photos and analyzing them to get insights.
Hence, depending of the region where the event is
taking place, the proposed engagement measurement
framework will be accompanied with a number of
procedures, policies, and guidelines.
We have demonstrated in this study the role of AI
in leveraging event marketing performance, focusing
on attendee engagement as an indicator of the quality
of presentations/events. Our proposed platform ana-
lyzes the feedback of attendees by observing the at-
tendees’ facial expressions. The role of the platform
becomes even more vital in the case of presentations
in which there is no human presenter. In this case, the
system is expected to make an automatic intervention
as a reaction to the feedback gathered. To achieve
this, the system is fed with a number of presenting
formats such as image, video, or text. As a reaction
to the attendees’ feelings, the system would automat-
ically shift between these formats to produce the best
results.
REFERENCES
Campbell, C., Sands, S., Ferraro, C., Tsao, H.-Y. J.,
and Mavrommatis, A. (2020). From data to ac-
tion: How marketers can leverage ai. Business
Horizons, 63(2):227–243. ARTIFICIAL INTELLI-
GENCE AND MACHINE LEARNING.
De Bruyn, A., Viswanathan, V., Beh, Y. S., Brock, J. K.-
U., and von Wangenheim, F. (2020). Artificial intelli-
gence and marketing: Pitfalls and opportunities. Jour-
nal of Interactive Marketing, 51:91–105.
Ekman, P. (1993). Facial expression and emotion. American
psychologist, 48(4):384.
Findface. Face detection, verification and recognition tech-
nology. Available at https://findface.pro/en/.
Gupta, S. (2003). Event marketing: Issues and challenges.
IIMB Management Review, 15(2):87–96.
Hoyle, L. H. (2016). Event marketing. John Wiley & Sons,
Inc.
Huang, M.-H. and Rust, R. T. (2021). A strategic frame-
work for artificial intelligence in marketing. Journal
of the Academy of Marketing Science, 49(1):30–50.
Kietzmann, J., Paschen, J., and Treen, E. (2018). Artificial
intelligence in advertising: How marketers can lever-
age artificial intelligence along the consumer journey.
Journal of Advertising Research, 58:263–267.
Liu, B., Ding, M., Shaham, S., Rahayu, W., Farokhi, F., and
Lin, Z. (2021). When machine learning meets privacy:
A survey and outlook. ACM Comput. Surv., 54(2).
Neuhofer, B., Magnus, B., and Celuch, K. (2020). The
impact of artificial intelligence on event experiences:
a scenario technique approach. Electronic Markets,
pages 1–17.
Niedenthal, P. M., Halberstadt, J. B., Margolin, J., and
Innes-Ker,
˚
A. H. (2000). Emotional state and the de-
tection of change in facial expression of emotion. Eu-
ropean journal of social psychology, 30(2):211–222.
Oseni, A., Moustafa, N., Janicke, H., Liu, P., Tari, Z., and
Vasilakos, A. (2021). Security and privacy for artifi-
cial intelligence: Opportunities and challenges. arXiv
preprint arXiv:2102.04661.
Richhariya, B. and Gupta, D. (2019). Facial expression
recognition using iterative universum twin support
vector machine. Applied Soft Computing, 76:53–67.
Services, H. B. R. A. (2018). The event marketing evolu-
tion. Technical report.
Sterne, J. (2017). Artificial intelligence for marketing:
practical applications. John Wiley & Sons.
Whitehill, J., Serpell, Z., Lin, Y.-C., Foster, A., and Movel-
lan, J. (2014). The faces of engagement: Automatic
recognition of student engagement from facial expres-
sions.
Woodward Jr, J. D., Horn, C., Gatune, J., and Thomas,
A. (2003). Biometrics: A look at facial recognition.
Technical report, RAND CORP SANTA MONICA
CA.
Zhu, T., Ye, D., Wang, W., Zhou, W., and Yu, P. S. (2020).
More than privacy: Applying differential privacy in
key areas of artificial intelligence. arXiv preprint
arXiv:2008.01916.
Leveraging Event Marketing Performance using AI in Facial Recognition
573