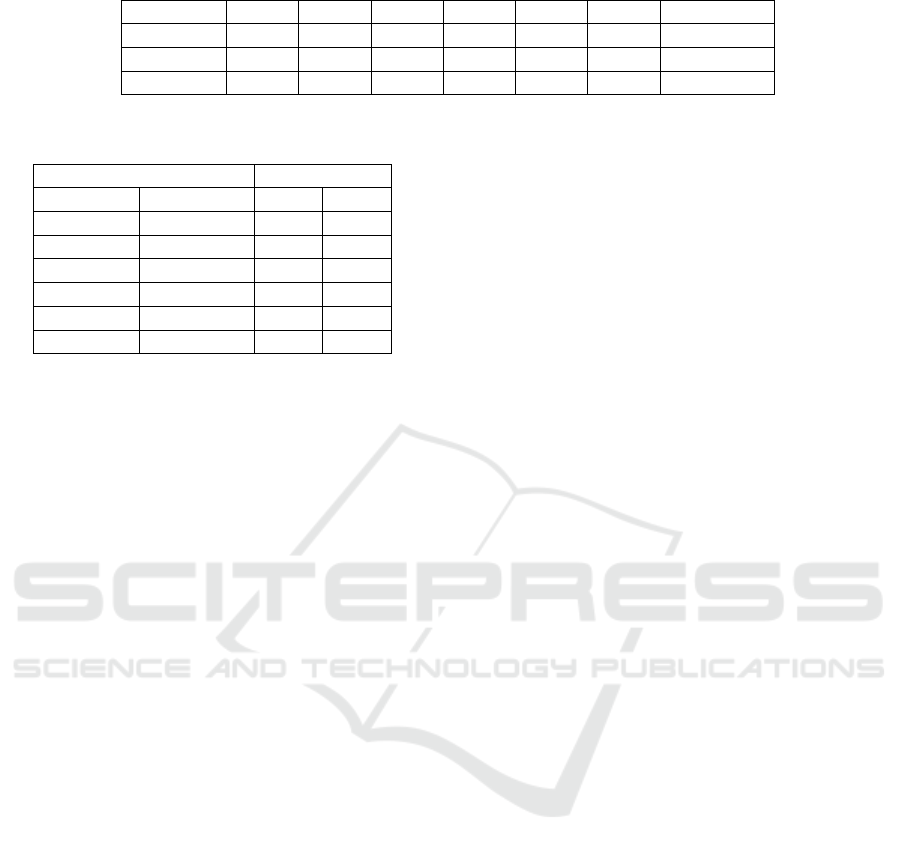
Table 2: Accuracy using different validation Folds with different color representations on the ISIC2017 dataset.
Accuracy Fold1 Fold2 Fold3 Fold4 Fold5 Mean Dimension
SCN 0.78 0.76 0.77 0.78 0.67 0.75 12
CH 0.71 0.78 0.75 0.77 0.63 0.72 255
colorSIFT 0.69 0.71 0.75 0.71 0.62 0.69 10000
Table 3: Accuracy using different representations and clas-
sifiers on the SD-198 dataset.
Accuracy
Features Dimension KNN SVM
CH 256 12.33 4.19
CN 21000 20.03 20.23
colorSIFT 21000 21.29 22.51
CN-L 21000 42.50 38.91
CCV-L 21000 42.80 40.13
SCN 12 5.58 4.73
proved utilizing a classifier with three different par-
titions. These color names are mainly extracted to
classify skin lesions for more accurate inspection of
melanomas, which are considered as the most fa-
tal skin cancer. The proposed method has shown a
notable performance for diagnosing melanomas. A
comparison of different handcrafted features is pre-
sented as well, which proves the efficiency of our
color name features against the state-of-the-art color
representations. Accordingly, using only our pro-
posed color-based features shows a promising result
compared to automatically extracted features using
deep learning. However, our proposed representation
method shows a limitation when using a benchmark
dataset that contains several skin conditions. Thus,
these color names can be further used with other
hand-crafted features and more sophisticated machine
learning models to inspect melanomas to ameliorate
the diagnosis process. Fuzzy features of color names
could be also introduced in future work.
REFERENCES
Abbasi, N. R., Shaw, H. M., Rigel, D. S., Friedman, R. J.,
McCarthy, W. H., Osman, I., Kopf, A. W., and Polsky,
D. (2004). Early diagnosis of cutaneous melanoma:
revisiting the abcd criteria. Jama, 292(22):2771–
2776.
Abdel-Hakim, A. E. and Farag, A. A. (2006). Csift: A sift
descriptor with color invariant characteristics. In 2006
IEEE computer society conference on computer vision
and pattern recognition (CVPR’06), volume 2, pages
1978–1983. Ieee.
Argenziano, G., Longo, C., Cameron, A., Cavicchini, S.,
Gourhant, J.-Y., Lallas, A., McColl, I., Rosendahl, C.,
Thomas, L., Tiodorovic-Zivkovic, D., et al. (2011).
Blue-black rule: a simple dermoscopic clue to recog-
nize pigmented nodular melanoma. British Journal of
Dermatology, 165(6):1251–1255.
Claridge, E., Cotton, S., Hall, P., and Moncrieff, M. (2003).
From colour to tissue histology: physics-based inter-
pretation of images of pigmented skin lesions. Medi-
cal Image Analysis, 7(4):489–502.
Codella, N. C., Gutman, D., Celebi, M. E., Helba, B.,
Marchetti, M. A., Dusza, S. W., Kalloo, A., Liopy-
ris, K., Mishra, N., Kittler, H., et al. (2018). Skin
lesion analysis toward melanoma detection: A chal-
lenge at the 2017 international symposium on biomed-
ical imaging (isbi), hosted by the international skin
imaging collaboration (isic). In 2018 IEEE 15th in-
ternational symposium on biomedical imaging (ISBI
2018), pages 168–172. IEEE.
Coomans, D. and Massart, D. L. (1982). Alternative k-
nearest neighbour rules in supervised pattern recogni-
tion: Part 1. k-nearest neighbour classification by us-
ing alternative voting rules. Analytica Chimica Acta,
136:15–27.
Daghrir, J., Tlig, L., Bouchouicha, M., and Sayadi, M.
(2020). Melanoma skin cancer detection using deep
learning and classical machine learning techniques: A
hybrid approach. In 2020 5th International Confer-
ence on Advanced Technologies for Signal and Image
Processing (ATSIP), pages 1–5. IEEE.
Dimitriou, F., Krattinger, R., Ramelyte, E., Barysch, M. J.,
Micaletto, S., Dummer, R., and Goldinger, S. M.
(2018). The world of melanoma: epidemiologic, ge-
netic, and anatomic differences of melanoma across
the globe. Current oncology reports, 20(11):1–9.
Gonzalez-Diaz, I. (2018). Dermaknet: Incorporating the
knowledge of dermatologists to convolutional neural
networks for skin lesion diagnosis. IEEE journal of
biomedical and health informatics, 23(2):547–559.
Grob, J. and Bonerandi, J. (1998). The ‘ugly duckling’sign:
identification of the common characteristics of nevi
in an individual as a basis for melanoma screening.
Archives of dermatology, 134(1):103–104.
Heinzerling, L. and Eigentler, T. K. (2021). Skin cancer
in childhood and adolescents: Treatment and implica-
tions for the long-term follow-up. In Late Treatment
Effects and Cancer Survivor Care in the Young, pages
349–355. Springer.
Khazaei, Z., Ghorat, F., Jarrahi, A., Adineh, H., Sohrabi-
vafa, M., and Goodarzi, E. (2019). Global incidence
and mortality of skin cancer by histological subtype
and its relationship with the human development in-
dex (hdi); an ecology study in 2018. World Cancer
Res J, 6(2):e13.
Magalhaes, C., Tavares, J. M. R., Mendes, J., and Vardasca,
R. (2021). Comparison of machine learning strategies
for infrared thermography of skin cancer. Biomedical
Signal Processing and Control, 69:102872.
A Supervised Quantification of the Color Names Characterizing the Visual Component Color in the ABCD Dermatological Criteria for a
Further Melanoma Inspection
153