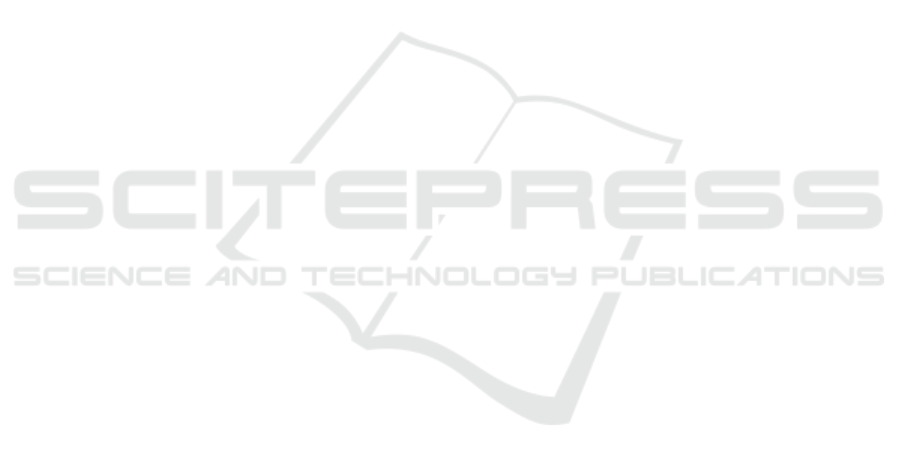
Huang, L., Fu, M., Li, F., Qu, H., Liu, Y., and Chen, W.
(2021). A deep reinforcement learning based long-
term recommender system. Knowledge-Based Systems,
213:106706.
Ikemoto, Y. and Kuwabara, K. (2019). On-the-spot knowl-
edge refinement for an interactive recommender sys-
tem. In ICAART.
Jeckmans, A. J., Beye, M., Erkin, Z., Hartel, P., Lagendijk,
R. L., and Tang, Q. (2013). Privacy in recommender
systems. In Social media retrieval, pages 263–281.
Springer.
Kim, D. and Suh, B. (2019). Enhancing vaes for collabo-
rative filtering: flexible priors & gating mechanisms.
Proceedings of the 13th ACM Conference on Recom-
mender Systems.
Koren, Y., Bell, R., and Volinsky, C. (2009). Matrix factoriza-
tion techniques for recommender systems. Computer,
42(8):30–37.
Liu, X., Deng, R. H., Raymond Choo, K.-K., and Yang,
Y. (2021). Privacy-preserving reinforcement learning
design for patient-centric dynamic treatment regimes.
IEEE Transactions on Emerging Topics in Computing,
9(1):456–470.
Mahmood, T., Ricci, F., Venturini, A., and H
¨
opken, W.
(2008). Adaptive recommender systems for travel plan-
ning. In ENTER, volume 8, pages 1–11.
Mnih, V., Kavukcuoglu, K., Silver, D., Rusu, A. A., Veness,
J., Bellemare, M. G., Graves, A., Riedmiller, M., Fidje-
land, A. K., Ostrovski, G., et al. (2015). Human-level
control through deep reinforcement learning. nature,
518(7540):529–533.
Nawrocka, A., Kot, A., and Nawrocki, M. (2018). Applica-
tion of machine learning in recommendation systems.
In 2018 19th International Carpathian Control Confer-
ence (ICCC), pages 328–331.
Park, J., Kim, D. S., and Lim, H. (2020). Privacy-preserving
reinforcement learning using homomorphic encryp-
tion in cloud computing infrastructures. IEEE Access,
8:203564–203579.
Rohde, D., Bonner, S., Dunlop, T., Vasile, F., and Karat-
zoglou, A. (2018). Recogym: A reinforcement
learning environment for the problem of product rec-
ommendation in online advertising. arXiv preprint
arXiv:1808.00720.
Rossi, S. and Cervone, F. (2016). Social utilities and per-
sonality traits for group recommendation: A pilot user
study. In Proceedings of the 8th International Confer-
ence on Agents and Artificial Intelligence - Volume 1:
ICAART,, pages 38–46. INSTICC, SciTePress.
Rossi, S., Cervone, F., and Barile, F. (2017). An off-line eval-
uation of users’ ranking metrics in group recommenda-
tion. In Proceedings of the 9th International Confer-
ence on Agents and Artificial Intelligence - Volume 1:
ICAART,, pages 252–259. INSTICC, SciTePress.
Salakhutdinov, R., Mnih, A., and Hinton, G. (2007). Re-
stricted boltzmann machines for collaborative filtering.
In Proceedings of the 24th international conference on
Machine learning, pages 791–798.
Shani, G., Heckerman, D., Brafman, R. I., and Boutilier, C.
(2005). An mdp-based recommender system. Journal
of Machine Learning Research, 6(9).
Shyong, K., Frankowski, D., Riedl, J., et al. (2006). Do
you trust your recommendations? an exploration of
security and privacy issues in recommender systems.
In International Conference on Emerging Trends in
Information and Communication Security, pages 14–
29. Springer.
Sutton, R. S. and Barto, A. G. (2011). Reinforcement learn-
ing: An introduction.
Van Hasselt, H., Guez, A., and Silver, D. (2016). Deep rein-
forcement learning with double q-learning. In Proceed-
ings of the AAAI Conference on Artificial Intelligence,
volume 30.
Walter, F. E., Battiston, S., and Schweitzer, F. (2007). A
model of a trust-based recommendation system on a
social network. Autonomous Agents and Multi-Agent
Systems, 16:57–74.
Wang, C., Zheng, Y., Jiang, J., and Ren, K. (2018). To-
ward privacy-preserving personalized recommendation
services. Engineering, 4(1):21–28.
Wang, S., Cao, L., Wang, Y., Sheng, Q. Z., Orgun, M., and
Lian, D. (2019). A survey on session-based recom-
mender systems. arXiv preprint arXiv:1902.04864.
Wang, Z., Schaul, T., Hessel, M., Hasselt, H., Lanctot, M.,
and Freitas, N. (2016). Dueling network architec-
tures for deep reinforcement learning. In International
conference on machine learning, pages 1995–2003.
PMLR.
Watkins, C. J. and Dayan, P. (1992). Q-learning. Machine
learning, 8(3-4):279–292.
Xin, X., Karatzoglou, A., Arapakis, I., and Jose, J. M.
(2020). Self-supervised reinforcement learning for
recommender systems. In Proceedings of the 43rd In-
ternational ACM SIGIR Conference on Research and
Development in Information Retrieval, pages 931–940.
Yodogawa, D. and Kuwabara, K. (2020). Reaching agree-
ment in an interactive group recommender system. In
ICAART.
Yu, Y. (2018). Towards sample efficient reinforcement learn-
ing. In IJCAI, pages 5739–5743.
Zhan, J., Hsieh, C.-L., Wang, I.-C., Hsu, T.-S., Liau, C.-J.,
and Wang, D.-W. (2010). Privacy-preserving collab-
orative recommender systems. IEEE Transactions on
Systems, Man, and Cybernetics, Part C (Applications
and Reviews), 40(4):472–476.
Zhao, X., Zhang, L., Ding, Z., Xia, L., Tang, J., and Yin, D.
(2018). Recommendations with negative feedback via
pairwise deep reinforcement learning. In Proceedings
of the 24th ACM SIGKDD International Conference
on Knowledge Discovery & Data Mining, pages 1040–
1048.
ICAART 2022 - 14th International Conference on Agents and Artificial Intelligence
596