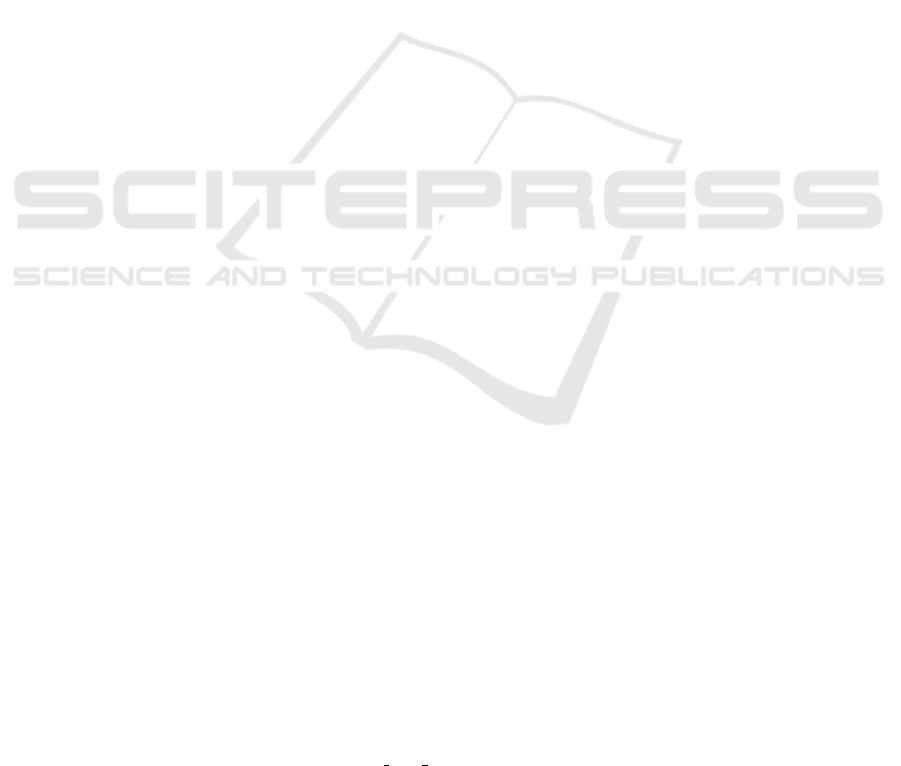
Both, the methodology and the gathered data were
enabled by closer collaboration with several players
and coaches from local table tennis associations. The
best result, with the overall dataset D5, was clearly
obtained by the ConvLSTM model, with a maximum
performance of 97.33% F1 score, among the best re-
sults published in the literature.
6.1 Future Work
Currently, the trained ConvLSTM model, together
with the SDA algorithm, is in the process of being in-
corporated into a WatchOS app to be used in real-time
classification experiments involving a limited number
of volunteer table tennis players. In the medium term
commercial use and widespread acceptance among
table tennis players, tested classifiers should be sub-
ject to a broad number of sensor datasets, preferably
from players with different levels of competition.
ACKNOWLEDGEMENTS
This work was partially funded by: AppGenera-
tion Software Technologies Lda; by Base Funding -
UIDB/00027/2020 of the Artificial Intelligence and
Computer Science Laboratory - LIACC - funded by
national funds through the FCT/MCTES (PIDDAC);
and by Fundac¸
˜
ao Ensino e Cultura Fernando Pessoa.
REFERENCES
Barshan, B. and Y
¨
uksek, M. C. (2014). Recognizing
Daily and Sports Activities in Two Open Source Ma-
chine Learning Environments Using Body-Worn Sen-
sor Units. The Computer Journal, 57(11):1649–1667.
Blank, P., Hoßbach, J., Schuldhaus, D., and Eskofier, B. M.
(2015). Sensor-based stroke detection and stroke type
classification in table tennis. ISWC 2015 - Proceed-
ings of the 2015 ACM International Symposium on
Wearable Computers, 1(September):93–100.
Connaghan, D., Kelly, P., O’Connor, N. E., Gaffney, M.,
Walsh, M., and O’Mathuna, C. (2011). Multi-sensor
classification of tennis strokes. In Proceedings of
IEEE Sensors, pages 1437–1440.
Cust, E. E., Sweeting, A. J., Ball, K., and Robertson, S.
(2019). Machine and deep learning for sport-specific
movement recognition: a systematic review of model
development and performance. Journal of Sports Sci-
ences, 37(5):568–600.
Ferreira, N. (2021). Table Tennis Data Sets and Mod-
els. URL: https://github.com/MikaPower/ai ping
pong (accessed: 2021-10-28).
Kulkarni, K. M. and Shenoy, S. (2021). Table Tennis Stroke
Recognition Using Two-Dimensional Human Pose
Estimation. CVPR Sports Workshop 2021 (7th Inter-
national Workshop on Computer Vision in Sports).
Lim, S. M., Oh, H. C., Kim, J., Lee, J., and Park, J. (2018).
LSTM-Guided Coaching Assistant for Table Tennis
Practice. Sensors (Basel, Switzerland), 18(12):1–14.
Liu, R., Wang, Z., Shi, X., Zhao, H., Qiu, S., Li, J.,
and Yang, N. (2019). Table tennis stroke recognition
based on body sensor network, volume 11874 LNCS.
Springer International Publishing.
Neville, J., Wixted, A., Rowlands, D., and James, D. (2010).
Accelerometers: An underutilized resource in sports
monitoring. In Proceedings of the 2010 6th Inter-
national Conference on Intelligent Sensors, Sensor
Networks and Information Processing, ISSNIP 2010,
pages 287–290.
Ord
´
o
˜
nez, F. J. and Roggen, D. (2016). Deep Convo-
lutional and LSTM Recurrent Neural Networks for
Multimodal Wearable Activity Recognition. Sensors
2016, Vol. 16, Page 115, 16(1):115.
P
¨
arkk
¨
a, J., Ermes, M., Korpip
¨
a
¨
a, P., M
¨
antyj
¨
arvi, J., Peltola,
J., and Korhonen, I. (2006). Activity classification us-
ing realistic data from wearable sensors. IEEE Trans-
actions on Information Technology in Biomedicine,
10(1):119–128.
Roeder, L. (2021). Netron: viewer for neural network, deep
learning and machine learning models. URL: https://
github.com/lutzroeder/netron (accessed: 2021-10-28).
Sainath, T. N., Vinyals, O., Senior, A., and Sak, H. (2015).
Convolutional, long short-term memory, fully con-
nected deep neural networks. ICASSP, IEEE Inter-
national Conference on Acoustics, Speech and Signal
Processing - Proceedings, 2015-August:4580–4584.
Sensorsoscar, S., Lara, D., and Labrador, M. A. (2018). A
Survey on Human Activity Recognition using Wear-
able Sensors Oscar. IEEE COMMUNICATIONS SUR-
VEYS AND TUTORIALS, 15(3).
Shi, X., Chen, Z., Wang, H., Yeung, D.-Y., Wong, W.-k.,
and Woo, W.-c. (2015). Convolutional LSTM Net-
work: A Machine Learning Approach for Precipita-
tion Nowcasting. arXiv.
Thomas, B. (2021). Sensorlog, log and stream sensor
data. URL: https://apps.apple.com/us/app/sensorlog/
id388014573 (accessed: 2021-10-28).
Wu, Y., Lan, J., Shu, X., Ji, C., Zhao, K., Wang, J., and
Zhang, H. (2018). ITTVis: Interactive Visualization
of Table Tennis Data. IEEE Transactions on Visual-
ization and Computer Graphics, 24(1):709–718.
Xia, K., Huang, J., and Wang, H. (2020). LSTM-CNN Ar-
chitecture for Human Activity Recognition. IEEE Ac-
cess, 8:56855–56866.
Zeng, M., Nguyen, L. T., Yu, B., Mengshoel, O. J., Zhu, J.,
Wu, P., and Zhang, J. (2015). Convolutional Neural
Networks for human activity recognition using mobile
sensors. In Proceedings of the 2014 6th International
Conference on Mobile Computing, Applications and
Services, MobiCASE 2014, pages 197–205. Institute
of Electrical and Electronics Engineers Inc.
ICAART 2022 - 14th International Conference on Agents and Artificial Intelligence
636