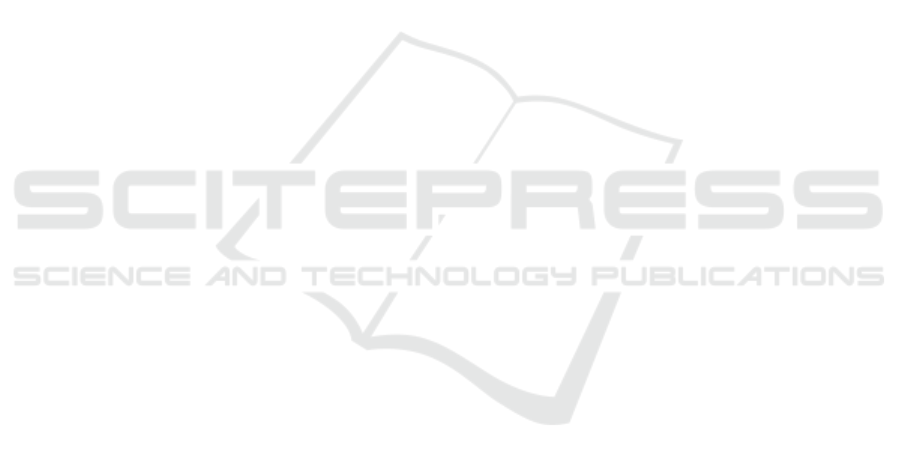
that the false alarm rate would decrease and overall
Dice score would increase further. In the future, we
would like to adapt our work in order to handle dif-
ferent image types, e.g. infrared images as well as
the combination of images and depth information. In
addition, we would also like to validate this approach
for the automated detection and segmentation of foam
in a chemical production installation.
ACKNOWLEDGEMENTS
We would like to thank VLAIO and BASF Antwerpen
for funding the project (HBC.2020.2876).
REFERENCES
Ackerman, S., Holz, R., Frey, R., Eloranta, E., Maddux,
B., and McGill, M. (2008). Cloud detection with
modis. part ii: validation. Journal of Atmospheric and
Oceanic Technology, 25(7):1073–1086.
Akcay, S., Atapour-Abarghouei, A., and Breckon, T. P.
(2018). Ganomaly: Semi-supervised anomaly detec-
tion via adversarial training. In Asian conference on
computer vision, pages 622–637. Springer.
Akc¸ay, S., Atapour-Abarghouei, A., and Breckon, T. P.
(2019). Skip-ganomaly: Skip connected and adversar-
ially trained encoder-decoder anomaly detection. In
2019 International Joint Conference on Neural Net-
works (IJCNN), pages 1–8. IEEE.
Br
´
eon, F.-M. and Colzy, S. (1999). Cloud detection from the
spaceborne polder instrument and validation against
surface synoptic observations. Journal of Applied Me-
teorology, 38(6):777–785.
Chan, T. F. and Vese, L. A. (2001). Active contours with-
out edges. IEEE Transactions on image processing,
10(2):266–277.
Dosovitskiy, A. and Brox, T. (2016). Generating images
with perceptual similarity metrics based on deep net-
works. Advances in neural information processing
systems, 29:658–666.
Ferecatu, M. and Boujemaa, N. (2007). Interactive remote-
sensing image retrieval using active relevance feed-
back. IEEE Transactions on Geoscience and Remote
Sensing, 45(4):818–826.
Frey, R. A., Ackerman, S. A., Liu, Y., Strabala, K. I., Zhang,
H., Key, J. R., and Wang, X. (2008). Cloud detection
with modis. part i: Improvements in the modis cloud
mask for collection 5. Journal of atmospheric and
oceanic technology, 25(7):1057–1072.
King, M. D., Platnick, S., Menzel, W. P., Ackerman, S. A.,
and Hubanks, P. A. (2013). Spatial and temporal dis-
tribution of clouds observed by modis onboard the
terra and aqua satellites. IEEE transactions on geo-
science and remote sensing, 51(7):3826–3852.
Kiran, B. R., Thomas, D. M., and Parakkal, R. (2018).
An overview of deep learning based methods for un-
supervised and semi-supervised anomaly detection in
videos. Journal of Imaging, 4(2):36.
Li, L., Li, X., Jiang, L., Su, X., and Chen, F. (2021). A
review on deep learning techniques for cloud detec-
tion methodologies and challenges. Signal, Image and
Video Processing, pages 1–9.
Melgani, F. and Bruzzone, L. (2004). Classification of hy-
perspectral remote sensing images with support vector
machines. IEEE Transactions on geoscience and re-
mote sensing, 42(8):1778–1790.
Movia, A., Beinat, A., and Crosilla, F. (2016). Shadow de-
tection and removal in rgb vhr images for land use
unsupervised classification. ISPRS Journal of Pho-
togrammetry and Remote Sensing, 119:485–495.
Nimmegeers, P., Vallerio, M., Telen, D., Van Impe, J., and
Logist, F. (2019). Interactive multi-objective dynamic
optimization of bioreactors under parametric uncer-
tainty. Chemie Ingenieur Technik, 91(3):349–362.
Ozkan, S., Efendioglu, M., and Demirpolat, C. (2018).
Cloud detection from rgb color remote sensing images
with deep pyramid networks. In IGARSS 2018-2018
IEEE International Geoscience and Remote Sensing
Symposium, pages 6939–6942. IEEE.
Saunders, R. (1986). An automated scheme for the re-
moval of cloud contamination from avhrr radiances
over western europe. International Journal of Remote
Sensing, 7(7):867–886.
Saunders, R. W. and Kriebel, K. T. (1988). An improved
method for detecting clear sky and cloudy radiances
from avhrr data. International Journal of Remote
Sensing, 9(1):123–150.
Schlegl, T., Seeb
¨
ock, P., Waldstein, S. M., Schmidt-Erfurth,
U., and Langs, G. (2017). Unsupervised anomaly de-
tection with generative adversarial networks to guide
marker discovery. In International conference on in-
formation processing in medical imaging, pages 146–
157. Springer.
Srinivas, A. (2020). Cloud and non-cloud images(anomaly
detection).
Tao, L., Yuan, L., and Sun, J. (2009). Skyfinder: attribute-
based sky image search. ACM transactions on graph-
ics (TOG), 28(3):1–5.
Wang, Z., Bovik, A. C., Sheikh, H. R., and Simoncelli, E. P.
(2004). Image quality assessment: from error visi-
bility to structural similarity. IEEE transactions on
image processing, 13(4):600–612.
Xie, F., Shi, M., Shi, Z., Yin, J., and Zhao, D. (2017). Multi-
level cloud detection in remote sensing images based
on deep learning. IEEE Journal of Selected Topics
in Applied Earth Observations and Remote Sensing,
10(8):3631–3640.
Yang, Y., Chen, J., Wang, R., Ma, T., Wang, L., Chen, J.,
Zheng, W.-S., and Zhang, T. (2021). Towards unbi-
ased covid-19 lesion localisation and segmentation via
weakly supervised learning. In 2021 IEEE 18th Inter-
national Symposium on Biomedical Imaging (ISBI),
pages 1966–1970. IEEE.
Zhang, Q. and Xiao, C. (2014). Cloud detection of rgb color
aerial photographs by progressive refinement scheme.
IEEE Transactions on Geoscience and Remote Sens-
ing, 52(11):7264–7275.
Semi-Supervised Cloud Detection with Weakly Labeled RGB Aerial Images using Generative Adversarial Networks
635