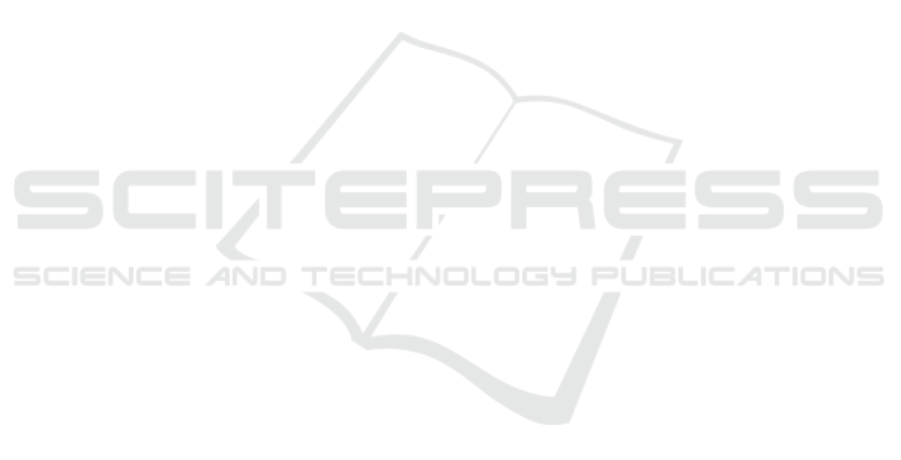
shot is represented with the center frame, and base-
line methods (ResNet50, VGG16) are established.
The dataset has a size of 1885 samples and six dif-
ferent shot types. Additionally, a user study has
been conducted to compare the results with human
classification. Compared to state-of-the-art datasets
(e.g., Cinescale, MovieGraphs), the published Hist-
Shot dataset focuses on historical documentaries and
original digitized film reels. In a follow-up investi-
gation, the dataset will be extended with additional
cinematographic annotations such as shot boundaries,
shot-based shot types, and camera movements. More-
over, the dataset will include exclusive original digi-
tized footage related to the Second World War (about
100 films).
ACKNOWLEDGEMENTS
Visual History of the Holocaust: Rethinking Cura-
tion in the Digital Age (Zechner and Loebenstein,
2019). This project has received funding from the Eu-
ropean Union’s Horizon 2020 research and innovation
program under the Grant Agreement 822670. Spe-
cial thanks to all participants (film experts and non-
experts) of the survey.
REFERENCES
Awad, G., Butt, A. A., Curtis, K., Fiscus, J. G., Godil,
A., Lee, Y., Delgado, A., Zhang, J., Godard, E.,
Chocot, B., Diduch, L. L., Liu, J., Smeaton, A. F.,
Graham, Y., Jones, G. J. F., Kraaij, W., and Qu
´
enot, G.
(2021). TRECVID 2020: A comprehensive campaign
for evaluating video retrieval tasks across multiple ap-
plication domains. CoRR, abs/2104.13473.
Benini, S., Savardi, M., B
´
alint, K., Kov
´
acs, A. B., and Sig-
noroni, A. (2019). On the influence of shot scale on
film mood and narrative engagement in film viewers.
IEEE Transactions on Affective Computing, pages 1–
1.
Benini, S., Svanera, M., Adami, N., Leonardi, R., and
Kov
´
acs, A. B. (2016). Shot scale distribution
in art films. Multimedia Tools and Applications,
75(23):16499–16527.
Carreira, J. and Zisserman, A. (2017). Quo vadis, action
recognition? a new model and the kinetics dataset.
In 2017 IEEE Conference on Computer Vision and
Pattern Recognition (CVPR), pages 4724–4733, Los
Alamitos, CA, USA. IEEE Computer Society.
Cherif, I., Solachidis, V., and Pitas, I. (2007). Shot type
identification of movie content. In 2007 9th Interna-
tional Symposium on Signal Processing and Its Ap-
plications, pages 1–4, Sharjah, United Arab Emirates.
IEEE.
Deng, Z., Hu, X., Zhu, L., Xu, X., Qin, J., Han, G., and
Heng, P.-A. (2018). R³net: Recurrent residual refine-
ment network for saliency detection. In Proceedings
of the Twenty-Seventh International Joint Conference
on Artificial Intelligence, IJCAI-18, pages 684–690.
International Joint Conferences on Artificial Intelli-
gence Organization.
Fl
¨
uckiger, B., Pfluger, D., Trumpy, G., Aydin, T., and
Smolic, A. (2018). Film material-scanner interaction.
Technical report, University of Zurich, Zurich.
Fossati, G. (2018). From Grain to Pixel - The Archival Life
of Film in Transition. Amsterdam University Press,
Amsterdam.
Fossati, G. and van den Oever, A. (2016). Exposing the Film
Apparatus. Amsterdam University Press, Amsterdam.
Government, U. S. (1934). The U.S. National Archives and
Records Administration. https://www.archives.gov/.
[Online; last accessed 31.05.2021].
Government, U. S. (1993). United States Holocaust Memo-
rial Museum. https://www.ushmm.org/. [Online; last
accessed 31.05.2021].
Gu, C., Sun, C., Ross, D. A., Vondrick, C., Pantofaru, C., Li,
Y., Vijayanarasimhan, S., Toderici, G., Ricco, S., Suk-
thankar, R., Schmid, C., and Malik, J. (2018). Ava: A
video dataset of spatio-temporally localized atomic vi-
sual actions. In Proceedings of the IEEE Conference
on Computer Vision and Pattern Recognition (CVPR).
Helm, D. and Kampel, M. (2019a). Shot boundary detec-
tion for automatic video analysis of historical films.
In Cristani, M., Prati, A., Lanz, O., Messelodi, S.,
and Sebe, N., editors, New Trends in Image Analysis
and Processing – ICIAP 2019, pages 137–147, Cham.
Springer International Publishing.
Helm, D. and Kampel, M. (2019b). Video Shot Analy-
sis for Digital Curation and Preservation of Histori-
cal Films. In Rizvic, S. and Rodriguez Echavarria,
K., editors, Eurographics Workshop on Graphics and
Cultural Heritage. The Eurographics Association.
Huang, Q., Xiong, Y., Rao, A., Wang, J., and Lin, D. (2020).
Movienet: A holistic dataset for movie understand-
ing. Lecture Notes in Computer Science (including
subseries Lecture Notes in Artificial Intelligence and
Lecture Notes in Bioinformatics), 12349 LNCS:709–
727.
Kahle, B. (1996). Internet archive. https://archive.org/. [On-
line; last accessed 2020/11/09].
Luca, C., Sergio, B., and Riccardo, L. (2013). Classify-
ing cinematographic shot types. Multimedia Tools and
Applications, 62(1):51–73.
Marszalek, M., Laptev, I., and Schmid, C. (2009). Actions
in context. In 2009 IEEE Conference on Computer
Vision and Pattern Recognition, pages 2929–2936.
Miech, A., Zhukov, D., Alayrac, J.-B., Tapaswi, M., Laptev,
I., and Sivic, J. (2019). Howto100m: Learning a text-
video embedding by watching hundred million nar-
rated video clips. In Proceedings of the IEEE/CVF
International Conference on Computer Vision (ICCV).
Rao, A., Wang, J., Xu, L., Jiang, X., Huang, Q., Zhou, B.,
and Lin, D. (2020). A unified framework for shot type
ICPRAM 2022 - 11th International Conference on Pattern Recognition Applications and Methods
642