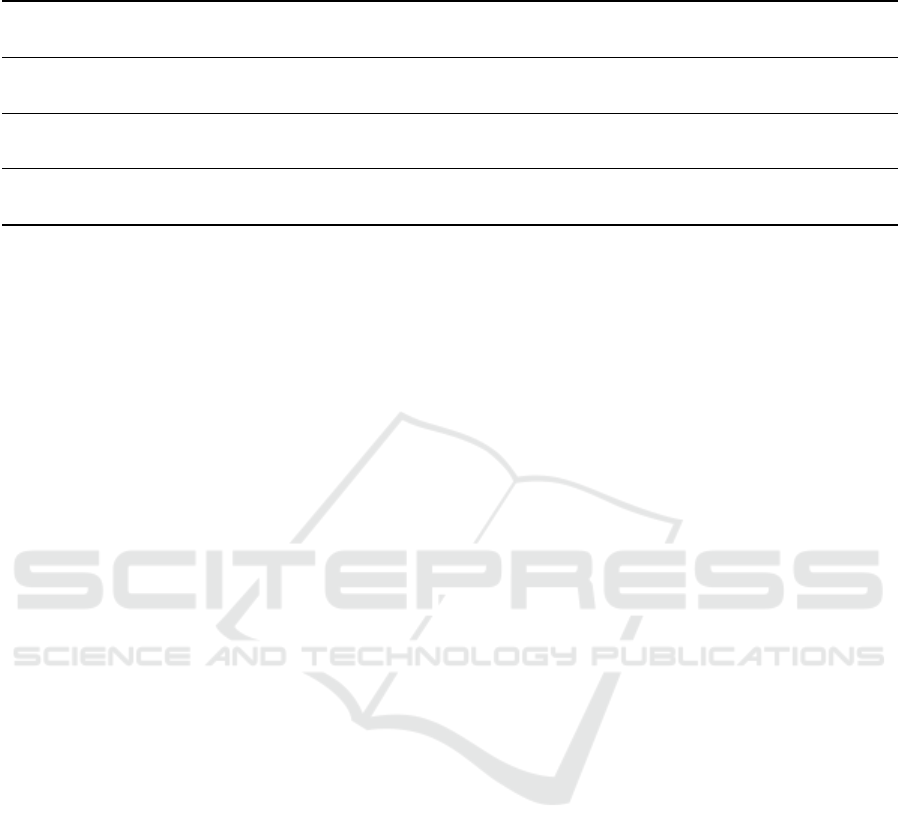
Table 2: Overview on examples for typical scenarios using e-assessment data along the two dimensions of data usage.
Type of data Cases with one-time use Cases with frequent use Cases with continuous
use
Anonymous or ag-
gregated data
research studies quality assurance feedback
Individual, identifi-
able data
— plagiarism check feedback, adaptivity,
competency measurement
Data merged with
external sources
research studies plagiarism check, predic-
tion
authentication
performed for the papers included in the search results
to get even more insights into the dimensions of data
usage as well as possible interconnections within and
between the different contexts of data usage. Second,
the results can be used as a starting point to make con-
nections to the usage of other data than e-assessment
data in similar context. For example, authentication,
privacy and plagiarism may also be relevant topics
in other areas of educational technology and beyond,
even if academic dishonesty may indeed only be a ma-
jor problem in the context of assessments. Third, the
results can be used to identify research gaps that re-
quire further attention. The results so far are surely
not yet detailed enough for that purpose, but the fact
that e. g. papers on data handling appear relatively
rarely in the search results may hint towards the fact
that the technical aspects on how to handle data within
e-assessment systems could possibly need further at-
tention in future research.
REFERENCES
Aldowah, H., Al-Samarraie, H., and Fauzy, W. M. (2019).
Educational data mining and learning analytics for
21st century higher education: A review and syn-
thesis. Telematics and informatics, (37):13–49.
Amigud, A., Arnedo-Moreno, J., Daradoumis, T., and
Guerrero-Roldan, A.-E. (2018). Open proctor: An
academic integrity tool for the open learning envir-
onment. In Lecture Notes on Data Engineering and
Communications Technologies, volume 8, pages 262–
273.
Azevedo, J., Oliveira, E., and Beites, P. (2019). Using learn-
ing analytics to evaluate the quality of multiple-choice
questions: A perspective with classical test theory and
item response theory. International Journal of Inform-
ation and Learning Technology, 36(4):322–341.
Bañeres, D., Noguera, I., Elena Rodríguez, M., and
Guerrero-Roldán, A. (2019). Using an intelligent tu-
toring system with plagiarism detection to enhance e-
assessment. In Lecture Notes on Data Engineering
and Communications Technologies, volume 23, pages
363–372.
Baró, X., Muñoz Bernaus, R., Baneres, D., and Guerrero-
Roldán, A. (2020). Biometric tools for learner identity
in e-assessment. In Lecture Notes on Data Engineer-
ing and Communications Technologies, volume 34,
pages 41–65.
Birjali, M., Beni-Hssane, A., and Erritali, M. (2018). A
novel adaptive e-learning model based on big data by
using competence-based knowledge and social learner
activities. Applied Soft Computing Journal, 69:14–32.
Bull, S., Wasson, B., Kickmeier-Rust, M., Johnson, M.,
Moe, E., Hansen, C., Meissl-Egghart, G., and Ham-
mermueller, K. (2012). Assessing english as a second
language: From classroom data to a competence-
based open learner model. In Proceedings of the 20th
International Conference on Computers in Education,
ICCE 2012, pages 618–622.
Carneiro, D., Novais, P., Durães, D., Pego, J., and Sousa, N.
(2019). Predicting completion time in high-stakes ex-
ams. Future Generation Computer Systems, 92:549–
559.
Derr, K., Hübl, R., and Ahmed, M. (2015). Using test data
for successive refinement of an online pre-course in
mathematics. In Proceedings of the European Confer-
ence on e-Learning, ECEL, pages 173–180.
Ellis, C. (2013). Broadening the scope and increasing the
usefulness of learning analytics: The case for assess-
ment analytics. British Journal of Educational Tech-
nology, 44(4):662–664.
Florián, B. E., Baldiris, S. M., and Fabregat, R. (2010).
A new competency-based e-assessment data model:
Implementing the aeea proposal. In IEEE Education
Engineering Conference, EDUCON 2010, pages 473–
480.
Gamulin, J., Gamulin, O., and Kermek, D. (2015). The ap-
plication of formative e-assessment data in final exam
results modeling using neural networks. In 2015 38th
International Convention on Information and Com-
munication Technology, Electronics and Microelec-
tronics, MIPRO 2015 - Proceedings, pages 726–730.
Geetha, V., Chandrakala, D., Nadarajan, R., and Dass, C.
(2013). A bayesian classification approach for hand-
ling uncertainty in adaptive e-assessment. Interna-
tional Review on Computers and Software, 8(4):1045–
1052.
Ihantola, P., Vihavainen, A., Ahadi, A., Butler, M., Börst-
ler, J., Edwards, S. H., Isohanni, E., Korhonen, A.,
Where Does All the Data Go? A Review of Research on E-Assessment Data
163