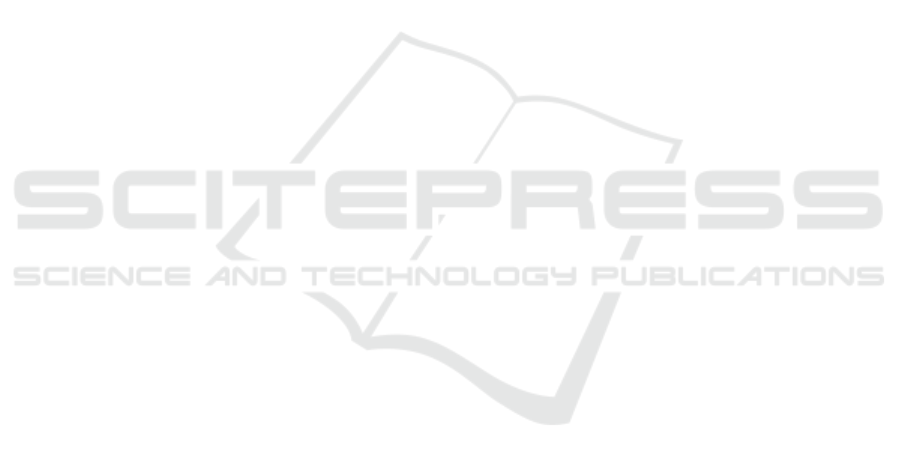
was observed to have influenced the learner more than
the tutor. The findings from the results and compar-
isons across both user studies shows the effectiveness
of the proposed learning task and the multi-agent in-
teraction in promoting self-regulation in the learner.
Altogether, the insights on perceptions and influence
of agent roles and related regulation behaviours in the
proposed interaction would act as the basis of our fur-
ther studies towards understanding distinct regulation
strategies and their relevance in various phases of self-
regulated learning.
REFERENCES
Bartneck, C., Kuli
´
c, D., Croft, E., and Zoghbi, S. (2009).
Measurement instruments for the anthropomorphism,
animacy, likeability, perceived intelligence, and per-
ceived safety of robots. International journal of social
robotics, 1(1):71–81.
Baylor, A. L. and Kim, Y. (2005). Simulating instructional
roles through pedagogical agents. International Jour-
nal of Artificial Intelligence in Education, 15(1):95.
Breazeal, C., Harris, P. L., DeSteno, D., Kory Westlund,
J. M., Dickens, L., and Jeong, S. (2016). Young chil-
dren treat robots as informants. Topics in cognitive
science, 8(2):481–491.
Coppola, B. P. and Pontrello, J. K. (2014). Using errors to
teach through a two-staged, structured review: Peer-
reviewed quizzes and “what’s wrong with me?”. Jour-
nal of Chemical Education, 91(12):2148–2154.
Deci, E. L., Hodges, R., Pierson, L., and Tomassone, J.
(1992). Autonomy and competence as motivational
factors in students with learning disabilities and emo-
tional handicaps. Journal of learning disabilities,
25(7):457–471.
Geiskkovitch, D. Y., Thiessen, R., Young, J. E., and Glen-
wright, M. R. (2019). What? that’s not a chair!: How
robot informational errors affect children’s trust to-
wards robots. In 2019 14th ACM/IEEE International
Conference on Human-Robot Interaction (HRI), pages
48–56. IEEE.
J
¨
arvel
¨
a, S., J
¨
arvenoja, H., Malmberg, J., and Hadwin, A. F.
(2013). Exploring socially shared regulation in the
context of collaboration. Journal of Cognitive Educa-
tion and Psychology, 12(3):267–286.
Kim, Y., Baylor, A. L., Group, P., et al. (2006). Pedagogi-
cal agents as learning companions: The role of agent
competency and type of interaction. Educational tech-
nology research and development, 54(3):223–243.
Kory-Westlund, J. M. and Breazeal, C. (2019). Assess-
ing children’s perceptions and acceptance of a so-
cial robot. In Proceedings of the 18th ACM Inter-
national Conference on Interaction Design and Chil-
dren, pages 38–50.
Nomura, T., Kanda, T., and Suzuki, T. (2006). Experimen-
tal investigation into influence of negative attitudes to-
ward robots on human–robot interaction. Ai & Soci-
ety, 20(2):138–150.
Ogan, A., Finkelstein, S., Mayfield, E., D’Adamo, C., Mat-
suda, N., and Cassell, J. (2012). ” oh dear stacy!” so-
cial interaction, elaboration, and learning with teach-
able agents. In Proceedings of the SIGCHI conference
on human factors in computing systems, pages 39–48.
Okita, S. Y. (2014). Learning from the folly of others:
Learning to self-correct by monitoring the reasoning
of virtual characters in a computer-supported mathe-
matics learning environment. Computers & Educa-
tion, 71:257–278.
Panadero, E. (2017). A review of self-regulated learning:
Six models and four directions for research. Frontiers
in psychology, 8:422.
Pelachaud, C. (2015). Greta: an interactive expressive
embodied conversational agent. In Proceedings of
the 2015 International Conference on Autonomous
Agents and Multiagent Systems, pages 5–5.
Ryan, R. M. and Connell, J. P. (1989). Perceived locus of
causality and internalization: examining reasons for
acting in two domains. Journal of personality and so-
cial psychology, 57(5):749.
Ryan, R. M. and Deci, E. L. (2000). Self-determination the-
ory and the facilitation of intrinsic motivation, social
development, and well-being. American psychologist,
55(1):68.
Stone, B. A. and Lester, J. C. (1996). Dynamically sequenc-
ing an animated pedagogical agent. In AAAI/IAAI, Vol.
1, pages 424–431.
Weiss, A., Scherndl, T., Buchner, R., and Tscheligi, M.
(2010). A robot as persuasive social actor a field
trial on child–robot interaction. In Proceedings of
the 2nd international symposium on new frontiers in
human–robot interaction—a symposium at the AISB
2010 convention, pages 136–142.
Yadollahi, E., Johal, W., Paiva, A., and Dillenbourg, P.
(2018). When deictic gestures in a robot can harm
child-robot collaboration. In Proceedings of the 17th
ACM Conference on Interaction Design and Children,
pages 195–206.
ICAART 2022 - 14th International Conference on Agents and Artificial Intelligence
344