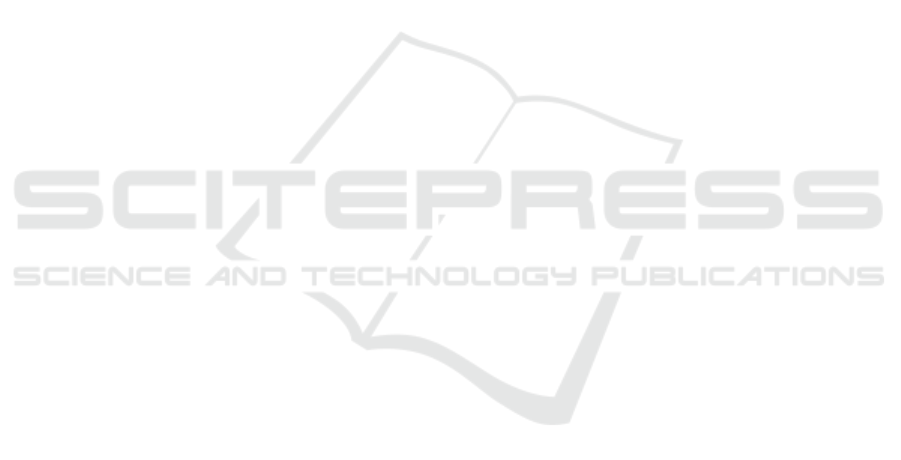
the Greyscale image is able to outperform the RGB
ones, regardless of the size used by the latter. As
future work, we are currently investigating whether
the same considering emerging from the experimen-
tal analysis we presented can be demonstrated on mal-
ware working on different operating systems i.e., iOS
and Microsoft Windows.
REFERENCES
(last visit 09 April 2015). Virustotal. https://www.virustotal.
com/.
(last visit 25 November 2014). Google play un-
official python api. https://github.com/egirault/
googleplay-api.
Arp, D., Spreitzenbarth, M., Huebner, M., Gascon, H., and
Rieck, K. (2014). Drebin: Efficient and explainable
detection of android malware in your pocket. In Pro-
ceedings of 21th Annual Network and Distributed Sys-
tem Security Symposium (NDSS).
Arzt, S., Rasthofer, S., Fritz, C., Bodden, E., Bartel, A.,
Klein, J., Le Traon, Y., Octeau, D., and McDaniel, P.
(2014). Flowdroid: Precise context, flow, field, object-
sensitive and lifecycle-aware taint analysis for android
apps. ACM SIGPLAN Notices, 49(6):259–269.
Bhodia, N., Prajapati, P., Di Troia, F., and Stamp, M.
(2019). Transfer learning for image-based malware
classification. arXiv preprint arXiv:1903.11551.
Blasing, T., Schmidt, A.-D., Batyuk, L., Camtepe, S. A.,
and Albayrak, S. (2010). An android application
sandbox system for suspicious software detection. In
Proceedings of 5th International Conference on Mali-
cious and Unwanted Software.
Canfora, G., Di Sorbo, A., Mercaldo, F., and Visag-
gio, C. A. (2015). Obfuscation techniques against
signature-based detection: a case study. In 2015
Mobile Systems Technologies Workshop (MST), pages
21–26. IEEE.
Canfora, G., Mercaldo, F., and Visaggio, C. A. (2013). A
classifier of malicious android applications. In Pro-
ceedings of the 2nd International Workshop on Secu-
rity of Mobile Applications, in conjunction with the In-
ternational Conference on Availability, Reliability and
Security.
Cimitile, A., Mercaldo, F., Nardone, V., Santone, A., and
Visaggio, C. A. (2017). Talos: no more ransomware
victims with formal methods. International Journal of
Information Security, pages 1–20.
Ciobanu, M. G., Fasano, F., Martinelli, F., Mercaldo, F.,
and Santone, A. (2019). A data life cycle modeling
proposal by means of formal methods. In Proceedings
of the 2019 ACM Asia Conference on Computer and
Communications Security, pages 670–672. ACM.
Dixon, B., Jiang, Y., Jaiantilal, A., and Mishra, S. (2011).
Location based power analysis to detect malicious
code in smartphones. In Proceedings of the 1st ACM
workshop on Security and privacy in smartphones and
mobile devices.
Faiella, M., La Marra, A., Martinelli, F., Mercaldo, F.,
Saracino, A., and Sheikhalishahi, M. (2017). A
distributed framework for collaborative and dynamic
analysis of android malware. In 2017 25th Euromi-
cro International Conference on Parallel, Distributed
and Network-Based Processing (PDP), pages 321–
328. IEEE.
Fasano, F., Martinelli, F., Mercaldo, F., and Santone, A.
(2019). Energy consumption metrics for mobile de-
vice dynamic malware detection. Procedia Computer
Science, 159:1045–1052.
Feizollah, A., Anuar, N. B., Salleh, R., Suarez-Tangil, G.,
and Furnell, S. (2017). Androdialysis: analysis of an-
droid intent effectiveness in malware detection. com-
puters & security, 65:121–134.
Ferrante, A., Medvet, E., Mercaldo, F., Milosevic, J., and
Visaggio, C. A. (2016). Spotting the malicious mo-
ment: Characterizing malware behavior using dy-
namic features. In Availability, Reliability and Secu-
rity (ARES), 2016 11th International Conference on,
pages 372–381. IEEE.
Gandotra, E., Bansal, D., and Sofat, S. (2014). Malware
analysis and classification: A survey. Journal of In-
formation Security, 5(02):56.
Google (2015). Play. https://play.google.com/store.
Kim, H., Smith, J., and Shin, K. G. (2008). Detecting
energy-greedy anomalies and mobile malware vari-
ants. In Proceedings of the 6th international confer-
ence on Mobile systems, applications, and services.
Lindorfer, M., Neugschwandtner, M., and Platzer, C.
(2015). Marvin: Efficient and comprehensive mobile
app classification through static and dynamic analy-
sis. In Computer Software and Applications Confer-
ence (COMPSAC), 2015 IEEE 39th Annual, volume 2,
pages 422–433. IEEE.
Martinelli, F., Mercaldo, F., Nardone, V., Santone, A., San-
gaiah, A. K., and Cimitile, A. (2018). Evaluating
model checking for cyber threats code obfuscation
identification. Journal of Parallel and Distributed
Computing, 119:203–218.
Martinelli, F., Mercaldo, F., and Santone, A. (2019). So-
cial network polluting contents detection through deep
learning techniques. In 2019 International Joint Con-
ference on Neural Networks (IJCNN), pages 1–10.
IEEE.
Martinelli, F., Mercaldo, F., and Saracino, A. (2017). Bride-
maid: An hybrid tool for accurate detection of android
malware. In Proceedings of the 2017 ACM on Asia
Conference on Computer and Communications Secu-
rity, pages 899–901. ACM.
Mercaldo, F., Nardone, V., and Santone, A. (2016a). Ran-
somware inside out. In Availability, Reliability and
Security (ARES), 2016 11th International Conference
on, pages 628–637. IEEE.
Mercaldo, F., Nardone, V., Santone, A., and Visaggio, C. A.
(2016b). Hey malware, i can find you! In Enabling
Technologies: Infrastructure for Collaborative Enter-
prises (WETICE), 2016 IEEE 25th International Con-
ference on, pages 261–262. IEEE.
On the Influence of Image Settings in Deep Learning-based Malware Detection
675