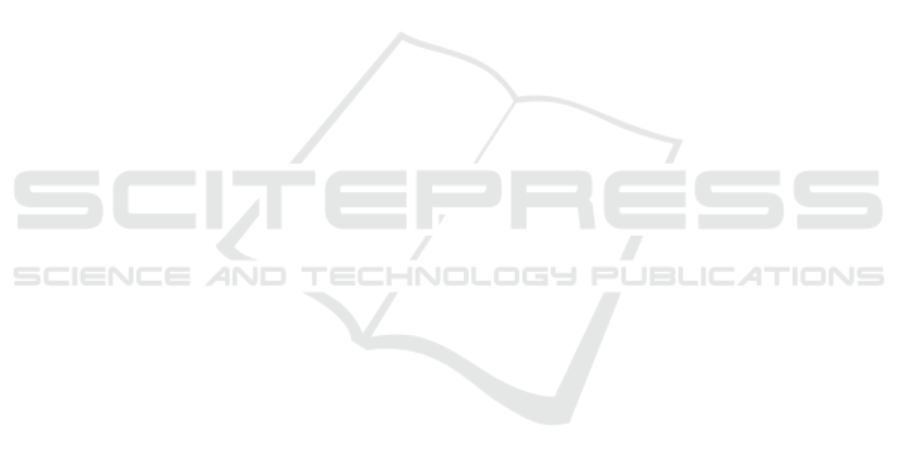
pages 252–261, Berlin, Heidelberg. Springer Berlin
Heidelberg.
Herbon, C. (2014). The hawkwood database. arXiv preprint
arXiv:1410.4393.
Herbon, C., T
¨
onnies, K., and Stock, B. (2014). Detection
and segmentation of clustered objects by using itera-
tive classification, segmentation, and gaussian mixture
models and application to wood log detection. In Ger-
man Conference on Pattern Recognition, pages 354–
364. Springer.
Janai, J., G
¨
uney, F., Behl, A., Geiger, A., et al. (2020).
Computer vision for autonomous vehicles: Prob-
lems, datasets and state of the art. Foundations and
Trends
R
in Computer Graphics and Vision, 12(1–
3):1–308.
Kakani, V., Nguyen, V. H., Kumar, B. P., Kim, H., and Pa-
supuleti, V. R. (2020). A critical review on computer
vision and artificial intelligence in food industry. Jour-
nal of Agriculture and Food Research, 2:100033.
Khalid, M., Lee, E. L. Y., Yusof, R., and Nadaraj, M.
(2008). Design of an intelligent wood species recogni-
tion system. International Journal of Simulation Sys-
tem, Science and Technology, 9(3):9–19.
Knyaz, V., Visilter, Y., and Zheltov, S. (2004). Photogram-
metric techniques for measurements in woodworking
industry. International Archives of the Photogramme-
try, Remote Sensing and Spatial Information Sciences
Proceedings, V. XXXIII. Part B5/2, pages 42–47.
Kruglov, A. V. (2016). Development of the rounded objects
automatic detection method for the log deck volume
measurement. In First International Workshop on Pat-
tern Recognition, volume 10011, page 1001104. Inter-
national Society for Optics and Photonics.
Kryl, M., Danys, L., Jaros, R., Martinek, R., Kodytek, P.,
and Bilik, P. (2020). Wood recognition and quality
imaging inspection systems. Journal of Sensors, 2020.
Mu, H., Qi, D., Zhang, M., and Yu, L. (2008). Image edge
detection of wood defects based on multi-fractal anal-
ysis. In 2008 IEEE International Conference on Au-
tomation and Logistics, pages 1232–1237. IEEE.
Nasirzadeh, M., Khazael, A. A., and bin Khalid, M. (2010).
Woods recognition system based on local binary pat-
tern. In 2010 2nd International Conference on Com-
putational Intelligence, Communication Systems and
Networks, pages 308–313. IEEE.
Paula Filho, P., Oliveira, L. S., Britto, A. d. S., and
Sabourin, R. (2010). Forest species recognition using
color-based features. In 2010 20th International Con-
ference on Pattern Recognition, pages 4178–4181.
IEEE.
Paula Filho, P. L., Oliveira, L. S., Nisgoski, S., and Britto,
A. S. (2014). Forest species recognition using macro-
scopic images. Machine Vision and Applications,
25(4):1019–1031.
Praschl, C., Auersperg-Castell, P., Brigitte, F.-H., and Zwet-
tler, G. A. (2021). Multi-resolution localization of in-
dividual logs in wooden piles utilizing yolo with tiling
on client/server architectures. Proceedings of 33rd
European Modeling & Simulation Symposium.
Ronneberger, O., Fischer, P., and Brox, T. (2015). U-net:
Convolutional networks for biomedical image seg-
mentation.
Schraml, R. and Uhl, A. (2014). Similarity based cross-
section segmentation in rough log end images. In
Iliadis, L., Maglogiannis, I., and Papadopoulos, H.,
editors, Artificial Intelligence Applications and Inno-
vations, pages 614–623, Berlin, Heidelberg. Springer
Berlin Heidelberg.
Seng, L. K. and Guniawan, T. (2018). An experimental
study on the use of visual texture for wood identifica-
tion using a novel convolutional neural network layer.
In 2018 8th IEEE International Conference on Con-
trol System, Computing and Engineering (ICCSCE),
pages 156–159. IEEE.
Shustrov, D. (2018). Species identification of wooden ma-
terial using convolutional neural networks. Master’s
thesis, Lappeenranta University of Technology School
of Engineering Science.
Tou, J. Y., Lau, P. Y., and Tay, Y. H. (2007). Computer
vision-based wood recognition system. In Proceed-
ings of International workshop on advanced image
technology, pages 197–202. Citeseer.
Tou, J. Y., Tay, Y. H., and Lau, P. Y. (2009). Ro-
tational invariant wood species recognition through
wood species verification. In 2009 First Asian Con-
ference on Intelligent Information and Database Sys-
tems, pages 115–120. IEEE.
Urbonas, A., Raudonis, V., Maskeli
¯
unas, R., and
Dama
ˇ
sevi
ˇ
cius, R. (2019). Automated identification of
wood veneer surface defects using faster region-based
convolutional neural network with data augmentation
and transfer learning. Applied Sciences, 9(22):4898.
Villalba-Diez, J., Schmidt, D., Gevers, R., Ordieres-Mer,
J., Buchwitz, M., and Wellbrock, W. (2019). Deep
learning for industrial computer vision quality control
in the printing industry 4.0. Sensors, 19(18).
Wang, C.-Y., Bochkovskiy, A., and Liao, H.-Y. M. (2020).
Scaled-yolov4: Scaling cross stage partial network.
arXiv preprint arXiv:2011.08036.
Xu, S., Wang, J., Shou, W., Ngo, T., Sadick, A.-M., and
Wang, X. (2020). Computer vision techniques in con-
struction: a critical review. Archives of Computational
Methods in Engineering, pages 1–15.
Yadav, A. R., Dewal, M., Anand, R., and Gupta, S. (2013).
Classification of hardwood species using ann clas-
sifier. In 2013 fourth national conference on com-
puter vision, pattern recognition, image processing
and graphics (NCVPRIPG), pages 1–5. IEEE.
Yusof, R., Khalid, M., and M. Khairuddin, A. S. (2013).
Application of kernel-genetic algorithm as nonlinear
feature selection in tropical wood species recognition
system. Computers and Electronics in Agriculture,
93:68–77.
ICPRAM 2022 - 11th International Conference on Pattern Recognition Applications and Methods
688