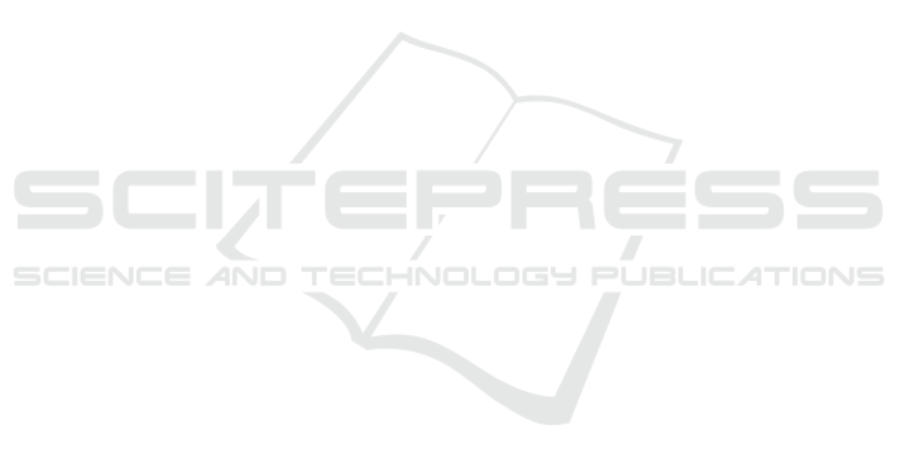
Edwards III, G. A., Gamez, N., Escobedo Jr, G., Calderon,
O., and Moreno-Gonzalez, I. (2019). Modifiable risk
factors for alzheimer’s disease. Frontiers in aging neu-
roscience, 11(164).
El-Sappagh, S., Abuhmed, T., Islam, S. R., and Kwak, K. S.
(2020). Multimodal multitask deep learning model
for alzheimer’s disease progression detection based on
time series data. Neurocomputing, 412:197–215.
Farouk, Y. and Rady, S. (2020). Early diagnosis of
alzheimer’s disease using unsupervised clustering. In-
ternational Journal of Intelligent Computing and In-
formation Sciences, 20(2):112–124.
Feng, J., Zhang, S.-W., Chen, L., and Xia, J.
(2021). Alzheimer’s disease classification using
features extracted from nonsubsampled contourlet
subband-based individual networks. Neurocomputing,
421:260–272.
Grassi, M., Rouleaux, N., Caldirola, D., Loewenstein, D.,
Schruers, K., Perna, G., Dumontier, M., Initiative,
A. D. N., et al. (2019). A novel ensemble-based
machine learning algorithm to predict the conversion
from mild cognitive impairment to alzheimer’s disease
using socio-demographic characteristics, clinical in-
formation, and neuropsychological measures. Front
Neurology, 10(756).
Haaksma, M. L., Calder
´
on-Larra
˜
naga, A., Rikkert, M.
G. O., Melis, R. J., and Leoutsakos, J. S. (2018). Cog-
nitive and functional progression in alzheimer disease:
A prediction model of latent classes. International
journal of geriatric psychiatry, 33(8).
Hameed, B., Elfetouh, A., and Elkheir, M. A. (2015).
Data cleaningtool: Usageoffuzzyroughsettheory as
machine learningpre-processing. International Jour-
nal of Intelligent Computing and Information Sci-
ences, 15:41–54.
Hancer, E., Xue, B., and Zhang, M. (2020). A survey on
feature selection approaches for clustering. Artificial
Intelligence Review volume, 53:4519–4545.
Helal, M., Elmogy, M., and Al-Awady, R. (2015). Using
rough set and boosting ensemble techniques to en-
hance classification performance of hepatitis c virus.
International Journal of Intelligent Computing and
Information Sciences, 15:45–59.
Hochreiter, S. and Schmidhuber, J. (1997). Long Short-
Term Memory. Neural Computation, 9(8):1735–1780.
Hong, X., Lin, R., Yang, C., Zeng, N., Cai, C., Gou, J., and
Yang, J. (2019). Predicting alzheimer’s disease using
lstm. IEEE Access, 7:80893–80901.
Janghel, R. R. (2020). Deep-Learning-Based Classification
and Diagnosis of Alzheimer’s Disease, chapter 76,
page 25. IGI Global.
Khaire, U. M. and Dhanalakshmi, R. (2019). Stability of
feature selection algorithm: A review. Journal of
King Saud University – Computer and Information
Sciences.
Liu, L., Zhao, S., Chen, H., and Wang, A. (2020). A new
machine learning method for identifying alzheimer’s
disease. Simulation Modelling Practice and Theory,
99.
Mart
´
ı-Juan, G., Sanroma-Guell, G., and Piellaa, G. (2020).
A survey on machine and statistical learning for lon-
gitudinal analysis of neuroimaging data in alzheimer’s
disease. Computer Methods and Programs in
Biomedicine.
Perneczky, R., editor (2018). Biomarkers for Preclinical
Alzheimer’s Disease, volume 137 of Neuromethods.
Humana Press, New York, NY.
Piccialli, F., Giampaolo, F., Prezioso, E., Camacho, D.,
and Acampora, G. (2021). Artificial intelligence and
healthcare: Forecasting of medical bookings through
multi-source time-series fusion. Information Fusion,
74:1–16.
Qiu, S., Chang, G. H., Panagia, M., Gopal, D. M., Au,
R., and Kolachalama, V. B. (2018). Fusion of deep
learning models of mri scans, mini-mental state ex-
amination, and logical memory test enhances diagno-
sis of mild cognitive impairment. Alzheimers Dement
(Amst)., 28(10):737–749.
Remeseiro, B. and Bolon-Canedo, V. (2019). A review
of feature selection methods in medical applications.
Computers in Biology and Medicine, 112.
Shikalgar, A. and Sonavane, S. (2020). Hybrid deep learn-
ing approach for classifying alzheimer disease based
on multimodal data. In Iyer, B., Deshpande, P. S.,
Sharma, S. C., and Shiurkar, U., editors, Computing
in Engineering and Technology, pages 511–520, Sin-
gapore. Springer Singapore.
Soliman, S. A., El-Dahshan, E.-S. A., and Salem, A.-B. M.
(2021). Diagnosis of alzheimer’s disease by three
dimensional convolutional neural network using un-
supervised feature learning method. International
Journal of Intelligent Computing and Information Sci-
ences.
Tanveer, M., Richhariya, B., Khan, R. U., Rashid, A. H.,
Khanna, P., Prasad, M., and Lin, C. T. (2020).
Machine learning techniques for the diagnosis of
alzheimer’s disease: A review. ACM Transactions on
Multimedia Computing, Communications, and Appli-
cations, 16(15).
Vemuri, P., Lesnick, T. G., Przybelski, S. A., Knopman,
D. S., Lowe, V. J., Graff-Radford, J., Roberts, R. O.,
Mielke, M. M., Machulda, M. M., Petersen, R. C.,
et al. (2017). Age, vascular health, and alzheimerdis-
ease biomarkers in an elderly sample. Annals of neu-
rology, 82:706–718.
Yang, C., Delcher, C., Shenkman, E., and Ranka, S. (2018).
Machine learning approaches for predicting high cost
high need patient expenditures in health care. BioMed-
ical Engineering OnLine, 17(113).
Zhong, Y., Yang, H., Zhang, Y., Li, P., and Ren, C. (2021).
Long short-term memory self-adapting online random
forests for evolving data stream regression. Neuro-
computing, 457:265–276.
ICPRAM 2022 - 11th International Conference on Pattern Recognition Applications and Methods
696