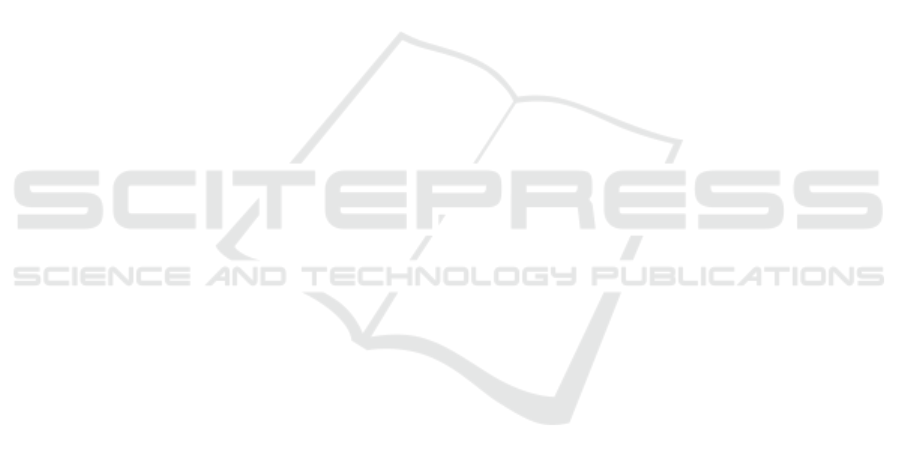
epistemic actions, nested epistemic statements etc.),
we also need to update the formal proofs regard-
ing the equivalence with possible worlds-based the-
ories. We also identified numerous ways of extend-
ing the expressive power of DE C K T , to account for
more complex cases, such as revision of beliefs (re-
call that DE C K T only supports knowledge, i.e., in
the presence of contradicting statements, the theory
collapses), potential action occurrences, beliefs of di-
verse types, among others.
From the practical standpoint, our main goal is
to evaluate how ToM can improve typical prediction
tasks that are of interest in the field of Computer Vi-
sion. Already recent studies, as by (Ji et al., 2021), try
to take advantage of past human-object interactions,
including where the user looked at, in order to pre-
dict future actions in videos. Datasets, such as Action
Genome, that provide annotations about attentional
relationships (whether a person is looking at some-
thing), in addition to spatial and contact relationships,
can help build cognitive models about the mental state
of users. In addition to such experiments, we also plan
to evaluate the proposed formalism in terms of scala-
bility and to further explore efficient means of imple-
menting HCDs, the main component that introduces
exponential complexity to the epistemic reasoner.
ACKNOWLEDGEMENTS
This project has received funding from the Hellenic
Foundation for Research and Innovation (HFRI) and
the General Secretariat for Research and Technology
(GSRT), under grant agreement No 188.
REFERENCES
Apperly, I. A. (2012). What is “theory of mind”? concepts,
cognitive processes and individual differences. Quar-
terly Journal of Experimental Psychology, 65(5):825–
839.
Chen, B., Vondrick, C., and Lipson, H. (2021). Visual be-
havior modelling for robotic theory of mind. Scientific
Reports, 11(1):424.
D’Asaro, F. A., Bikakis, A., Dickens, L., and Miller, R.
(2020). Probabilistic reasoning about epistemic action
narratives. Artificial Intelligence, 287:103352.
Davis, E. and Morgenstern, L. (2005). A First-order Theory
of Communication and Multi-agent Plans. Journal of
Logic and Computation, 15(5):701–749.
Ditmarsch, H. v., van der Hoek, W., and Kooi, B. (2007).
Dynamic Epistemic Logic. Springer Publishing Com-
pany, Incorporated, 1st edition.
Gelfond, M. and Lifschitz, V. (1988). The stable model
semantics for logic programming. In Proc. 5th Inter-
national Joint Conference and Symposium on Logic
Programming, IJCSLP 1988, pages 1070–1080.
Ji, J., Desai, R., and Niebles, J. C. (2021). Detecting human-
object relationships in videos. In Proceedings of the
IEEE/CVF International Conference on Computer Vi-
sion (ICCV), pages 8106–8116.
Kowalski, R. and Sergot, M. (1986). A logic-based calculus
of events. newgeneration computing 4.
Liu, Y. and Levesque, H. (2005). Tractable reasoning with
incomplete first-order knowledge in dynamic systems
with context-dependent actions. In IJCAI-05, pages
522–527.
Ma, J., Miller, R., Morgenstern, L., and Patkos, T. (2013).
An epistemic event calculus for asp-based reasoning
about knowledge of the past, present and future. In
LPAR 2013, 19th International Conference on Logic
for Programming, volume 26, pages 75–87.
Marcus, G. and Davis, E. (2019). Rebooting AI: Building
Artificial Intelligence We Can Trust. Pantheon Books,
USA.
Marek, V. W. and Truszczynski, M. (1999). Stable models
and an alternative logic programming paradigm. In
The Logic Programming Paradigm: A 25-Year Per-
spective, pages 375–398. Springer Berlin Heidelberg.
Miller, R. and Shanahan, M. (2002). Some alternative for-
mulations of the event calculus. In Computational
logic: logic programming and beyond, pages 452–
490. Springer.
Mueller, E. (2015). Commonsense Reasoning: An Event
Calculus Based Approach. Morgan Kaufmann Pub-
lishers Inc., San Francisco, CA, USA, 2
nd
edition.
Patkos, T. and Plexousakis, D. (2009). Reasoning with
knowledge, action and time in dynamic and uncertain
domains. In IJCAI-09.
Patkos, T., Plexousakis, D., Chibani, A., and Amirat, Y.
(2016). An event calculus production rule system for
reasoning in dynamic and uncertain domains. Theory
Pract. Log. Program., 16(3):325–352.
Scherl, R. (2003). Reasoning about the interaction of
knowlege, time and concurrent actions in the situation
calculus. In IJCAI-03, pages 1091–1096.
Schwering, C., Lakemeyer, G., and Pagnucco, M. (2015).
Belief revision and progression of knowledge bases in
the epistemic situation calculus. In IJCAI-15.
Shapiro, S., Pagnucco, M., Lesp
´
erance, Y., and Levesque,
H. (2011). Iterated belief change in the situation cal-
culus. Artificial Intelligence, 175(1):165–192.
Suchan, J., Bhatt, M., Wałega, P., and Schultz, C.
(2018). Visual explanation by high-level abduction:
On answer-set programming driven reasoning about
moving objects. In AAAI Conference on Artificial In-
telligence, pages 1965–1972.
Tsampanaki, N., Patkos, T., Flouris, G., and Plexousakis,
D. (2021). Revising event calculus theories to recover
from unexpected observations. Annals of Mathematics
and Artificial Intelligence, 89(1-2):209–236.
ICAART 2022 - 14th International Conference on Agents and Artificial Intelligence
696