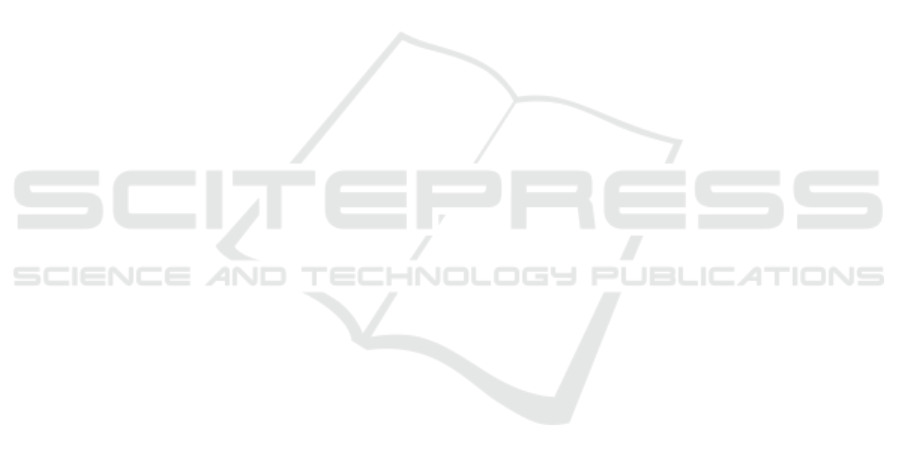
time-series analysis. Mathematical Problems in Engi-
neering, 2015:1–9.
Li, W., Zhang, Z., and Liu, Z. (2010). Action recognition
based on a bag of 3d points. In 2010 IEEE Computer
Society Conference on Computer Vision and Pattern
Recognition - Workshops, pages 9–14.
Lin, C.-H., Yumer, E., Wang, O., Shechtman, E., and Lucey,
S. (2018). St-gan: Spatial transformer generative
adversarial networks for image compositing. 2018
IEEE/CVF Conference on Computer Vision and Pat-
tern Recognition, pages 9455–9464.
Liu, J., Shahroudy, A., Xu, D., Kot, A. C., and Wang,
G. (2018). Skeleton-based action recognition using
spatio-temporal lstm network with trust gates. IEEE
Transactions on Pattern Analysis and Machine Intel-
ligence, 40:3007–3021.
Liu, J., Shahroudy, A., Xu, D., and Wang, G. (2016).
Spatio-temporal lstm with trust gates for 3d human ac-
tion recognition. ArXiv, abs/1607.07043.
Liu, J., Wang, G., Hu, P., Duan, L.-Y., and Kot, A. C.
(2017). Global context-aware attention lstm networks
for 3d action recognition. In Proceedings of the IEEE
Conference on Computer Vision and Pattern Recogni-
tion (CVPR).
Lv, F. and Nevatia, R. (2006). Recognition and segmenta-
tion of 3-d human action using hmm and multi-class
adaboost. In Leonardis, A., Bischof, H., and Pinz, A.,
editors, Computer Vision – ECCV 2006, pages 359–
372, Berlin, Heidelberg. Springer Berlin Heidelberg.
Noori, F. M., Wallace, B., Uddin, M. Z., and Tørresen, J.
(2019). A robust human activity recognition approach
using openpose, motion features, and deep recurrent
neural network. In SCIA.
Padilla-L
´
opez, J. R., Chaaraoui, A. A., and Fl
´
orez-Revuelta,
F. (2014). A discussion on the validation tests em-
ployed to compare human action recognition methods
using the msr action3d dataset. ArXiv, abs/1407.7390.
Paoletti, G., Cavazza, J., C¸ i
˘
gdem Beyan, and Bue, A. D.
(2021). Subspace clustering for action recognition
with covariance representations and temporal prun-
ing. 2020 25th International Conference on Pattern
Recognition (ICPR), pages 6035–6042.
Peng, W., Shi, J., Varanka, T., and Zhao, G. (2021). Re-
thinking the st-gcns for 3d skeleton-based human ac-
tion recognition. Neurocomputing, 454:45–53.
Seidenari, L., Varano, V., Berretti, S., Bimbo, A., and Pala,
P. (2013). Recognizing actions from depth cameras as
weakly aligned multi-part bag-of-poses. 2013 IEEE
Conference on Computer Vision and Pattern Recogni-
tion Workshops, pages 479–485.
Shotton, J., Sharp, T., Kipman, A., Fitzgibbon, A., Finoc-
chio, M., Blake, A., Cook, M., and Moore, R. (2013).
Real-time human pose recognition in parts from sin-
gle depth images. Communications of the ACM,
56(1):116–124.
Snoun, A., Jlidi, N., Bouchrika, T., Jemai, O., and Zaied,
M. (2021). Towards a deep human activity recogni-
tion approach based on video to image transformation
with skeleton data. Multimedia Tools and Applica-
tions, 80:29675–29698.
Taha, A., Zayed, H. H., Khalifa, M. E., and El-Horbaty, E.-
S. M. (2015). Human activity recognition for surveil-
lance applications. In ICIT 2015.
Theodorakopoulos, I., Kastaniotis, D., Economou, G., and
Fotopoulos, S. (2013). Pose-based human action
recognition via sparse representation in dissimilarity
space. Journal of Visual Communication and Image
Representation, 25:12–23.
Vaswani, A., Shazeer, N. M., Parmar, N., Uszkoreit, J.,
Jones, L., Gomez, A. N., Kaiser, L., and Polo-
sukhin, I. (2017). Attention is all you need. ArXiv,
abs/1706.03762.
Vemulapalli, R., Arrate, F., and Chellappa, R. (2014). Hu-
man action recognition by representing 3d skeletons
as points in a lie group. In 2014 IEEE Conference
on Computer Vision and Pattern Recognition, pages
588–595.
Wang, J., Liu, Z., Wu, Y., and Yuan, J. (2012). Mining
actionlet ensemble for action recognition with depth
cameras. 2012 IEEE Conference on Computer Vision
and Pattern Recognition, pages 1290–1297.
Wang, P., Li, W., Ogunbona, P., Gao, Z., and ling Zhang, H.
(2014). Mining mid-level features for action recogni-
tion based on effective skeleton representation. 2014
International Conference on Digital Image Comput-
ing: Techniques and Applications (DICTA), pages 1–
8.
Wang, P., Yuan, C., Hu, W., Li, B., and Zhang, Y. (2016).
Graph based skeleton motion representation and sim-
ilarity measurement for action recognition. In Leibe,
B., Matas, J., Sebe, N., and Welling, M., editors, Com-
puter Vision – ECCV 2016, pages 370–385, Cham.
Springer International Publishing.
Wu, D. and Shao, L. (2014). Leveraging hierarchical para-
metric networks for skeletal joints based action seg-
mentation and recognition. In Proceedings of the
IEEE Conference on Computer Vision and Pattern
Recognition (CVPR).
Xia, L., Chen, C.-C., and Aggarwal, J. K. (2012). View in-
variant human action recognition using histograms of
3d joints. 2012 IEEE Computer Society Conference on
Computer Vision and Pattern Recognition Workshops,
pages 20–27.
Yang, X. and Tian, Y. (2014). Effective 3d action recog-
nition using eigenjoints. Journal of Visual Communi-
cation and Image Representation, 25(1):2–11. Visual
Understanding and Applications with RGB-D Cam-
eras.
Zhao, R., Xu, W., Su, H., and Ji, Q. (2019). Bayesian hier-
archical dynamic model for human action recognition.
2019 IEEE/CVF Conference on Computer Vision and
Pattern Recognition (CVPR), pages 7725–7734.
Zhu, W., Lan, C., Xing, J., Li, Y., Shen, L., Zeng, W.,
and Xie, X. (2016). Co-occurrence feature learning
for skeleton based action recognition using regular-
ized deep lstm networks. page 8.
Zhu, Y., Chen, W., and Guo, G. (2013). Fusing spatiotem-
poral features and joints for 3d action recognition.
2013 IEEE Conference on Computer Vision and Pat-
tern Recognition Workshops, pages 486–491.
View-invariant 3D Skeleton-based Human Activity Recognition based on Transformer and Spatio-temporal Features
715