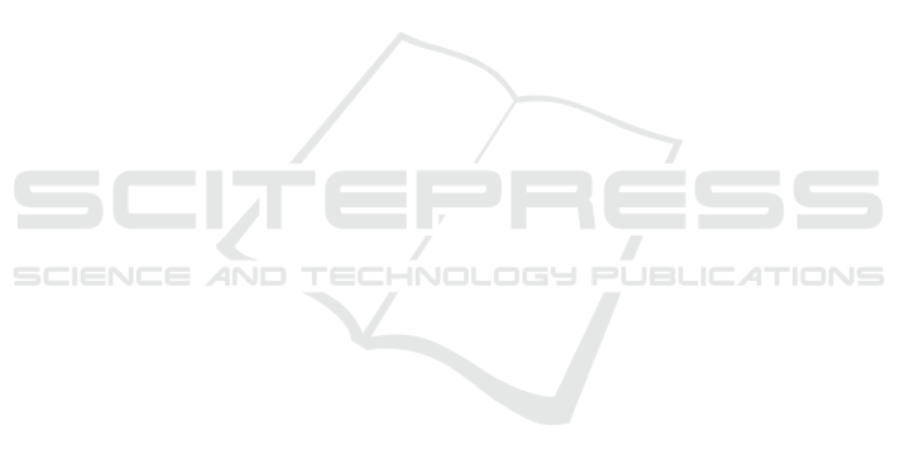
ployed in the production plan given by the solution
of (BP). As
˜
A
F
= A
F
for ω = 0, we neglect the corre-
sponding column in Table 2. The ’Overall’ row shows
the aggregate values on the average across instance
groups, except for column ’#lim’ that counts the total
number of time limits occurred.
Let us first comment ω = 2.5%. With respect to
ω = 0, the expected yield loss has a consistent de-
crease:
˜
Λ
%
= 19.7%, ranging from 13.4% to 23.0%.
This reduction is obtained by paying an average
+2.1% raw material to meet item demand, a value
that slightly increases with the amounts of small sizes
to cut. For ω = 5.0%, increasing raw material by
4.4% brings a mean yield loss reduction of 31.3%,
from a minimum 21.5% to a maximum 35.8%. The
trend continues up to ω = 10%, halving yield loss on
average (from 40.3% to 54.7%) while
˜
S
%
= 9.4%.
These result shows that, in the worst-case per-
spective described and as far as decision makers con-
sider the use of additional material, the solutions of
(BP) provide the fabrication process with increasing
resilience to defects. Indeed, with a mere count of
raw material, the reduction of defective parts does
not compensate the float glass increment: with ω =
2.5%, in front of an average +2.1% raw glass us-
age, only 0.4% is recovered in terms of faultless semi-
finite glass, so leaving a 1.7% material surplus unset-
tled. Similarly, ω = 5%,7.5%,10% respectively give
+3.7%,+6.0%,+8.4% net raw glass usage. This per-
formance is not surprising, and can generically be at-
tributed to the discrepancy between a continuous pa-
rameter (the amount of raw material supplied) and
discrete actions (sheet cuts). Instead, one must ob-
serve that raw material (siliceous sand, glass cullet,
additives) is only one component of semi-finite cost
(which includes among others energy and workforce),
and an algebraic sum of volumes is thus unfair. In
fact, the reduction of product defectiveness is defi-
nitely worth the cost of the additional material, not
only considering the added value of semi-finite items,
but also looking at the whole manufacturing process,
which is improved by (i) less item inspection and han-
dling, and (ii) downstream operation schedules less
vulnerable to delays.
On the computational side, finding optimal solu-
tions through (BP) requires more CPU time as the
size of |S| grows and constraint (8) is relaxed, taking
from 2.4 seconds to 123.2 seconds on average (last
row of the tables). In detail, T evidently increases
on instance groups G
5
-G
8
in all cases, that is, those
with the largest amount of small sizes. The time limit
is sporadically reached up to ω = 2.5%, and occurs
16 times for ω = 10%, that is, only in 8% of the in-
stances. In all the 47 cases where an optimum could
not be certified, we observed very large gaps: they
were under 30% in 4 cases only (with a minimum of
9.7%) and above 90% in 38 cases. Tests highlighted
that CPLEX struggled to tighten the dual bound and in
21 instances computation halted with a lower bound
equal to zero. Nevertheless, good primal bounds were
found in relatively few iterations and usually corre-
sponded to actual optima, when certified. All in all,
though the time response is not a critical aspect (be-
ing a mid-term planning problem), the picture so ob-
tained motivates us to investigate valid inequalities to
strengthen model (BP) or to identify combinatorial
dual bounds.
7 CONCLUSIONS AND FUTURE
RESEARCH
In this paper we discussed an assortment-and-cut
problem derived from a glass manufacturing process
where raw material is affected by imperfections that
can compromise the efficiency of the production. De-
fects are modeled as statistically independent points
uniformly-distributed on material, and we limit their
occurrence to at most one defect per large glass sheet.
Due to the simple form of cutting patterns, a critical
area can be identified, where a defect causes a small
item loss whatever the pattern layout. Based on this
observation, the probability that a defect induces a
yield loss is easily computed.
Following a worst-case perspective, we developed
an original bilevel approach where two decision mak-
ers operate with contrasting goals: while the leader
optimizes assortment and cuts to fulfill as much de-
mand as possible with a given amount of material,
the follower tries to impair it by distributing a given
amount of defects in a way that maximizes faulty
parts. We then rearranged the bilevel program into
an equivalent one-level 0-1 LP, whose optimal solu-
tions give an expectation of the yield reduction and a
measure of robustness against defectiveness.
We tested the formulation on a set of random in-
stances, limited in size but generated with a parameter
setting that reflects real-world production. When fed
with the minimum amount of raw material required
to fulfill demand in absence of defects, our model re-
turns a worst-case estimation of losses identical to that
achieved with defect-free optimal solutions obtained
as in (Arbib and Marinelli, 2007). Employing some
amount of extra glass leads to solution that are much
more robust against defect occurrence: for instance,
just supplying the system with 2.5% extra glass, one
can reduce mean yield loss by about 20%.
Although computational evidence on the largest
ICORES 2022 - 11th International Conference on Operations Research and Enterprise Systems
300