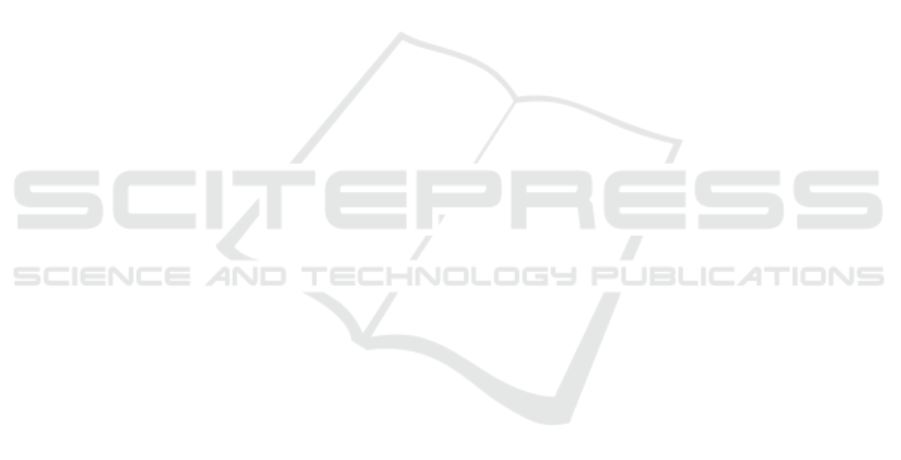
course, there exist many CRFs much more sophisti-
cated that the linear-chain CRF considered in the pa-
per. Let us cite some recent papers (Siddiqi, 2021;
Song et al., 2019; Quattoni et al., 2007; Kumar et al.,
2003; Saa and C¸ etin, 2012), among others. Compar-
ing different sophisticated CRFs to different PMCs
and TMCs will undoubtedly be an interesting second
perspective.
REFERENCES
Azeraf, E., Monfrini, E., and Pieczynski, W. (2021a). Us-
ing the Naive Bayes as a Discriminative Model. In
Proceedings of the 13th International Conference on
Machine Learning and Computing, pages 106–110.
Azeraf, E., Monfrini, E., Vignon, E., and Pieczynski, W.
(2020a). Hidden Markov Chains, Entropic Forward-
Backward, and Part-Of-Speech Tagging. arXiv
preprint arXiv:2005.10629.
Azeraf, E., Monfrini, E., Vignon, E., and Pieczynski, W.
(2020b). Highly Fast Text Segmentation With Pair-
wise Markov Chains. In Proceedings of the 6th IEEE
Congress on Information Science and Technology,
pages 361–366.
Azeraf, E., Monfrini, E., Vignon, E., and Pieczynski, W.
(2021b). Introducing the Hidden Neural Markov
Chain Framework. In Proceedings of the 13th Inter-
national Conference on Agents and Artificial Intelli-
gence - Volume 2, pages 1013–1020.
Baum, L. E., Petrie, T., Soules, G., and Weiss, N. (1970). A
Maximization Technique Occurring in the Statistical
Analysis of Probabilistic Functions of Markov Chains.
The Annals of Mathematical Statistics, 41(1):164–
171.
Boudaren, M. E. Y., Monfrini, E., Pieczynski, W., and Ais-
sani, A. (2014). Phasic Triplet Markov Chains. IEEE
Transactions on Pattern Analysis and Machine Intel-
ligence, 36(11):2310–2316.
Brants, T. (2000). TnT: a Statistical Part-of-Speech Tag-
ger. In Proceedings of the 6th Conference on Applied
Natural Language Processing, pages 224–231.
Capp
´
e, O., Moulines, E., and Ryden, T. (2005). Inference in
Hidden Markov Models. Springer Series in Statistics,
Berlin, Heidelberg.
Fang, M., Kodamana, H., and Huang, B. (2019). Real-Time
Mode Diagnosis for Processes with Multiple Operat-
ing Conditions using Switching Conditional Random
Fields. IEEE Transactions on Industrial Electronics,
67(6):5060–5070.
Fang, M., Kodamana, H., Huang, B., and Sammaknejad,
N. (2018). A Novel Approach to Process Operating
Mode Diagnosis using Conditional Random Fields in
the Presence of Missing Data. Computers & Chemical
Engineering, 111:149–163.
Gorynin, I., Gangloff, H., Monfrini, E., and Pieczynski, W.
(2018). Assessing the Segmentation Performance of
Pairwise and Triplet Markov Models. Signal Process-
ing, 145:183–192.
Jurafsky, D. and Martin, J. H. (2009). Speech and Lan-
guage Processing: An Introduction to Natural Lan-
guage Processing, Speech Recognition, and Compu-
tational Linguistics, 2nd Edition. Prentice-Hall.
Jurafsky, D. and Martin, J. H. (2021). Speech and Lan-
guage Processing, 3rd Edition Draft of December
2021. Copyright ©. All rights reserved.
Koski, T. (2001). Hidden Markov Models for Bioinformat-
ics. Springer Netherlands.
Kumar, S. et al. (2003). Discriminative Random Fields:
A Discriminative Framework for Contextual Interac-
tion in Classification. In Proceedings of the 9th IEEE
International Conference on Computer Vision, pages
1150–1157.
Lafferty, J. D., McCallum, A., and Pereira, F. C. (2001).
Conditional Random Fields: Probabilistic Models for
Segmenting and Labeling Sequence Data. In Proceed-
ings of the 18th International Conference on Machine
Learning, pages 282–289.
Lanchantin, P., Lapuyade-Lahorgue, J., and Pieczynski, W.
(2011). Unsupervised Segmentation of Randomly
Switching Data Hidden with non-Gaussian Correlated
Noise. Signal Processing, 91(2):163–175.
McCallum, A., Freitag, D., and Pereira, F. C. N. (2000).
Maximum Entropy Markov Models for Information
Extraction and Segmentation. In Proceedings of the
17th International Conference on Machine Learning,
page 591–598.
Pieczynski, W. (2003). Pairwise Markov Chains. IEEE
Transactions on Pattern Analysis and Machine Intel-
ligence, 25(5):634–639.
Quattoni, A., Wang, S., Morency, L.-P., Collins, M., and
Darrell, T. (2007). Hidden Conditional Random
Fields. IEEE Transactions on Pattern Analysis and
Machine Intelligence, 29(10):1848–1852.
Rabiner, L. R. (1989). A Tutorial on Hidden Markov Mod-
els and Selected Applications in Speech Recognition.
Proceedings of the IEEE, 77(2):257–286.
Saa, J. F. D. and C¸ etin, M. (2012). A Latent Discriminative
Model-Based Approach for Classification of Imagi-
nary Motor tasks from EEG Data. Journal of Neural
Engineering, 9(2).
Siddiqi, M. H. (2021). An Improved Gaussian Mixture
Hidden Conditional Random Fields Model for Audio-
Based Emotions Classification. Egyptian Informatics
Journal, 22(1):45–51.
Song, S., Zhang, N., and Huang, H. (2019). Named Entity
Recognition Based on Conditional Random Fields.
Cluster Computing, 22(3):5195–5206.
Sutton, C. and McCallum, A. (2006). An Introduction to
Conditional Random Fields for Relational Learning.
Introduction to Statistical Relational Learning, 2:93–
128.
ICAART 2022 - 14th International Conference on Agents and Artificial Intelligence
728