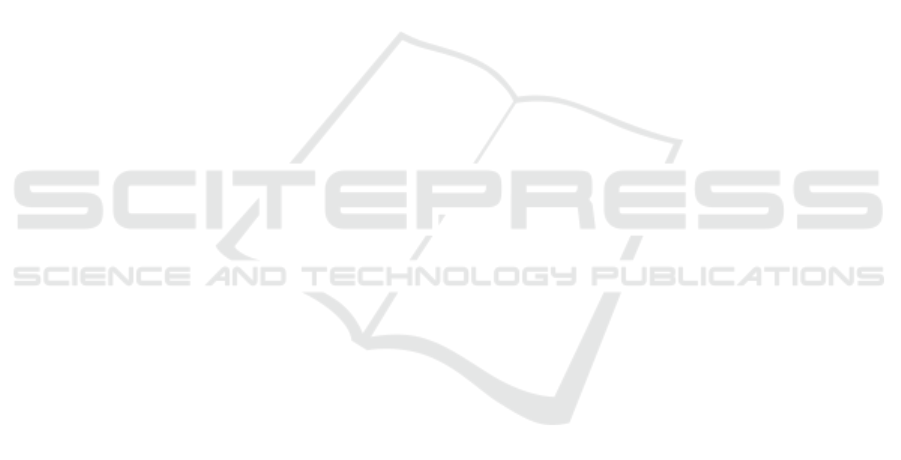
Kurze, A., Bischof, A., Totzauer, S., Storz, M., Eibl, M.,
Brereton, M., and Berger, A. (2020). Guess the data:
Data work to understand how people make sense of
and use simple sensor data from homes. In Proceed-
ings of the 2020 CHI Conference on Human Factors
in Computing Systems, pages 1–12.
Lake, B. M., Ullman, T. D., Tenenbaum, J. B., and Gersh-
man, S. J. (2017). Building machines that learn and
think like people. Behavioral and brain sciences, 40.
Laput, G., Zhang, Y., and Harrison, C. (2017). Synthetic
sensors: Towards general-purpose sensing. In Pro-
ceedings of the 2017 CHI Conference on Human Fac-
tors in Computing Systems, pages 3986–3999.
Le-Phuoc, D., Eiter, T., and Le-Tuan, A. (2021). A scalable
reasoning and learning approach for neural-symbolic
stream fusion. In Proceedings of the AAAI Conference
on Artificial Intelligence, volume 35, pages 4996–
5005.
Lin, J., Keogh, E., Lonardi, S., and Chiu, B. (2003). A sym-
bolic representation of time series, with implications
for streaming algorithms. In Proceedings of the 8th
ACM SIGMOD workshop on Research issues in data
mining and knowledge discovery, pages 2–11.
Llinas, J. (2014). A survey of automated methods for sense-
making support. In Next-Generation Analyst II, vol-
ume 9122, page 912206. International Society for Op-
tics and Photonics.
Marcus, G. and Davis, E. (2019). Rebooting AI: Building
artificial intelligence we can trust. Vintage.
Martin, D., Kühl, N., and Satzger, G. (2021). Virtual sen-
sors. Business & Information Systems Engineering,
63(3):315–323.
McCarthy, J. (2006). Challenges to machine learning: Rela-
tions between reality and appearance. In International
Conference on Inductive Logic Programming, pages
2–9. Springer.
Mueller, E. T. (2014). Commonsense reasoning: an event
calculus based approach. Morgan Kaufmann.
Neisser, U. (2014). Cognitive psychology: Classic edition.
Psychology Press.
Olszewski, R. T. (2001). Generalized feature extraction
for structural pattern recognition in time-series data.
Carnegie Mellon University.
Richardson, M. and Domingos, P. (2006). Markov logic
networks. Machine learning, 62(1-2):107–136.
Saker, M. K., Zhou, L., Eberhart, A., and Hitzler, P. (2021).
Neuro-symbolic artificial intelligence. AI Communi-
cations, pages 1–13.
Schoenfisch, J., Meilicke, C., von Stülpnagel, J., Ortmann,
J., and Stuckenschmidt, H. (2018). Root cause anal-
ysis in it infrastructures using ontologies and abduc-
tion in markov logic networks. Information Systems,
74:103–116.
Schurz, G. (2016). Common cause abduction: The for-
mation of theoretical concepts and models in science.
Logic Journal of the IGPL, 24(4):494–509.
Siddiqui, T., Luh, P., Wang, Z., Karahalios, K., and
Parameswaran, A. (2020). Shapesearch: A flexible
and efficient system for shape-based exploration of
trendlines. In Proceedings of the 2020 ACM SIG-
MOD International Conference on Management of
Data, pages 51–65.
Skarlatidis, A. and Michelioudakis, E. (2014). Logical
Markov Random Fields (LoMRF): an open-source
implementation of Markov Logic Networks.
Skarlatidis, A., Paliouras, G., Artikis, A., and Vouros, G. A.
(2015). Probabilistic event calculus for event recog-
nition. ACM Transactions on Computational Logic
(TOCL), 16(2):1–37.
Spelke, E. S. and Kinzler, K. D. (2007). Core knowledge.
Developmental science, 10(1):89–96.
Suchan, J., Bhatt, M., and Varadarajan, S. (2019). Out of
sight but not out of mind: An answer set programming
based online abduction framework for visual sense-
making in autonomous driving. In Proceedings of the
Twenty-Eighth International Joint Conference on Arti-
ficial Intelligence, IJCAI 2019, Macao, China, August
10-16, 2019, pages 1879–1885. ijcai.org.
Teijeiro, T. and Félix, P. (2018). On the adoption of abduc-
tive reasoning for time series interpretation. Artificial
Intelligence, 262:163–188.
Tran, S. D. and Davis, L. S. (2008). Event modeling and
recognition using markov logic networks. In Euro-
pean Conference on Computer Vision, pages 610–623.
Springer.
Tsitsipas, A., Eisenhart, G., Seybold, D., and Wesner, S.
(2022). Scalable shapeoid recognition on multivariate
data streams with apache beam. In Proceedings of the
Computing Conference 2022, forthcoming. Springer.
Tsitsipas, A., Schiessle, P., and Schubert, L. (2021). Scotty:
Fast a priori structure-based extraction from time se-
ries. In 2021 IEEE International Conference on Big
Data (BigData 2021), forthcoming.
Tsitsipas, A. and Schubert, L. (2021a). Modelling and
reasoning for indirect sensing over discrete-time via
markov logic networks. In Proceedings of the Twelfth
International Workshop Modelling and Reasoning in
Context (MRC 2021). CEUR-WS.org.
Tsitsipas, A. and Schubert, L. (2021b). On group theory and
interpretable time series primitives. In Proceedings
of the Seventeenth International Conference on Ad-
vanced Data Mining and Applications (ADMA 2021),
forthcoming. Springer.
Wasserkrug, S., Gal, A., Etzion, O., and Turchin, Y. (2010).
Efficient processing of uncertain events in rule-based
systems. IEEE Transactions on Knowledge and Data
Engineering, 24(1):45–58.
Towards an Ensemble Approach for Sensor Data Sensemaking
329