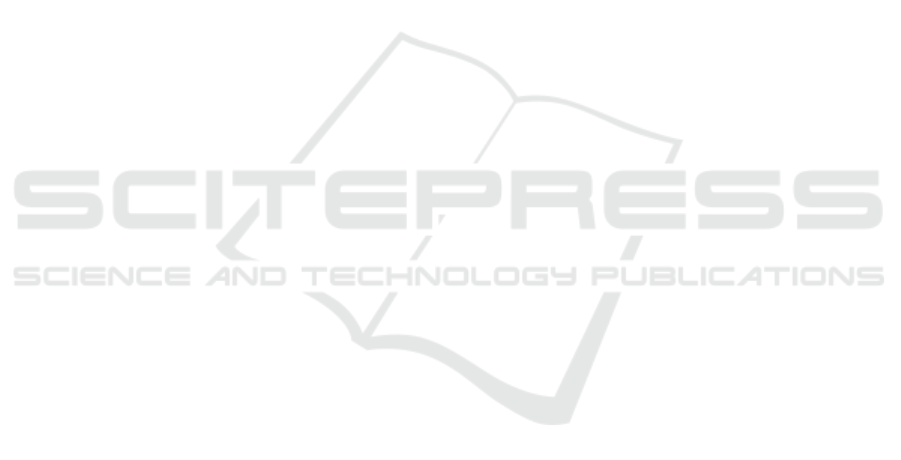
5 CONCLUSIONS AND FUTURE
WORK
This work introduces a methodology for strategi-
cally identifying and selecting the most informative
2D slices using a percentile-based-position-analysis
method. The impact of the proposed methodology on
the overall performance of CNN-based classification
models is explored experimentally. The slice subsets
contribution to the model performance varies accord-
ing to the position; the 35th percentile reaches the
higher accuracy. Based on the best average results,
the proposed methodology establishes the resize by
cropping technique, the image sizes of (224 × 224)
and (192 × 192) and the axial plane, as suitable to
get the highest model performance for Alzheimer’s
disease classification. The number of slices per sub-
ject greatly influences the model performance, sub-
sets with 32 slices presenting the best results.
The use of 2D slices produces an increased num-
ber of instances and the possibility of using existing
2D CNNs to train a model with transfer learning or
from scratch. The classifications obtained at the slice
level must be fused to obtain a classification at the
subject level. Finally, data leakage can be avoided by
using a subject dataset early split and creating an inde-
pendent test set as proposed in the instance selection
process.
For future work, image metrics will be used to
select the most informative instances. Also, custom
CNNs and model ensembles using the different planes
and cropping regions should be considered to improve
the classification model performance and reliability.
ACKNOWLEDGMENTS
This work is supported by the Smart Data Analy-
sis Systems Group - SDAS Research Group (http:
//sdas-group.com)
REFERENCES
Backstrom, K., Nazari, M., Gu, I. Y. H., and Jakola, A. S.
(2018). An efficient 3D deep convolutional network
for Alzheimer’s disease diagnosis using MR images.
Proceedings - International Symposium on Biomedi-
cal Imaging, 2018-April(Isbi):149–153.
Bae, J. B., Lee, S., Jung, W., Park, S., Kim, W., Oh, H., Han,
J. W., Kim, G. E., Kim, J. S., Kim, J. H., and Kim,
K. W. (2020). Identification of Alzheimer’s disease
using a convolutional neural network model based on
T1-weighted magnetic resonance imaging. Scientific
Reports, 10(1):1–10.
Duc, N. T., Ryu, S., Qureshi, M. N. I., Choi, M., Lee, K. H.,
and Lee, B. (2020). 3D-Deep Learning Based Au-
tomatic Diagnosis of Alzheimer’s Disease with Joint
MMSE Prediction Using Resting-State fMRI. Neu-
roinformatics, 18(1):71–86.
Farooq, A., Anwar, S., Awais, M., and Alnowami, M.
(2017a). Artificial intelligence based smart diagno-
sis of Alzheimer’s disease and mild cognitive impair-
ment. 2017 International Smart Cities Conference,
ISC2 2017, pages 0–3.
Farooq, A., Anwar, S. M., Awais, M., and Rehman, S.
(2017b). A deep CNN based multi-class classifica-
tion of Alzheimer’s disease using MRI. 2017 IEEE In-
ternational Conference on Imaging Systems and Tech-
niques (IST), pages 3–8.
Goodfellow, I., Bengio, Y., and Courville, A. (2016). Deep
Learning. MIT Press.
Guan, Z. (2019). A Comprehensive Study of Alzheimer ’ s
Disease. pages 1–16.
Hon, M. and Khan, N. M. (2017). Towards Alzheimer’s
disease classification through transfer learning. Pro-
ceedings - 2017 IEEE International Conference on
Bioinformatics and Biomedicine, BIBM 2017, 2017-
Janua:1166–1169.
Huang, G., Liu, Z., Van Der Maaten, L., and Weinberger,
K. Q. (2017). Densely connected convolutional net-
works. In Proceedings of the IEEE conference on
computer vision and pattern recognition, pages 4700–
4708.
Hussain, E., Hasan, M., Hassan, S. Z., Hassan Azmi, T.,
Rahman, M. A., and Zavid Parvez, M. (2020). Deep
Learning Based Binary Classification for Alzheimer’s
Disease Detection using Brain MRI Images. Proceed-
ings of the 15th IEEE Conference on Industrial Elec-
tronics and Applications, ICIEA 2020, pages 1115–
1120.
Jabason, E., Ahmad, M. O., and Swamy, M. N. (2019a).
Classification of Alzheimer’s Disease from MRI Data
Using an Ensemble of Hybrid Deep Convolutional
Neural Networks. Midwest Symposium on Circuits
and Systems, 2019-Augus(Mci):481–484.
Jabason, E., Omair Ahmad, M., and Swamy, M. N.
(2019b). Hybrid Feature Fusion Using RNN and Pre-
trained CNN for Classification of Alzheimer’s Disease
(Poster). FUSION 2019 - 22nd International Confer-
ence on Information Fusion, pages 2019–2022.
Khan, N. M., Abraham, N., and Hon, M. (2019). Trans-
fer Learning with Intelligent Training Data Selection
for Prediction of Alzheimer’s Disease. IEEE Access,
7:72726–72735.
Khvostikov, A., Aderghal, K., Krylov, A., Catheline,
G., and Benois-Pineau, J. (2018). 3D inception-
based CNN with sMRI and MD-DTI data fusion for
Alzheimer’s disease diagnostics. arXiv.
Lin, W., Tong, T., Gao, Q., Guo, D., Du, X., Yang, Y.,
Guo, G., Xiao, M., Du, M., and Qu, X. (2018). Con-
volutional neural networks-based MRI image analy-
sis for the Alzheimer’s disease prediction from mild
ICPRAM 2022 - 11th International Conference on Pattern Recognition Applications and Methods
336