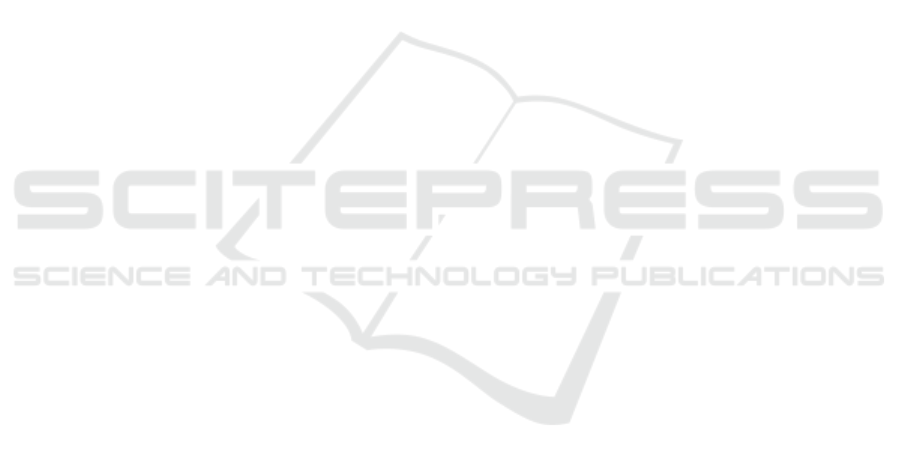
Therefore, general practitioners can be widely bene-
fited from our approach, although they did not acquire
a particular amount of obvious samples as an initial
training set. Based on our study’s proposition and
further revisits on the aforementioned avenues of im-
provement, we expect upcoming studies can provide
an effective solution to leverage ambiguous samples
on escalating the image recognition performance.
REFERENCES
Abdar, M., Pourpanah, F., Hussain, S., Rezazadegan, D.,
Liu, L., Ghavamzadeh, M., Fieguth, P., Cao, X., Khos-
ravi, A., Acharya, U. R., et al. (2021). A review of un-
certainty quantification in deep learning: Techniques,
applications and challenges. Information Fusion.
Arazo, E., Ortego, D., Albert, P., O’Connor, N. E., and
McGuinness, K. (2020). Pseudo-labeling and con-
firmation bias in deep semi-supervised learning. In
2020 International Joint Conference on Neural Net-
works (IJCNN), pages 1–8. IEEE.
Berthelot, D., Carlini, N., Goodfellow, I., Papernot, N.,
Oliver, A., and Raffel, C. (2019). Mixmatch: A
holistic approach to semi-supervised learning. arXiv
preprint arXiv:1905.02249.
Bulusu, S., Kailkhura, B., Li, B., Varshney, P. K., and Song,
D. (2020). Anomalous example detection in deep
learning: A survey. IEEE Access, 8:132330–132347.
Choi, Y., Uh, Y., Yoo, J., and Ha, J.-W. (2020). Stargan v2:
Diverse image synthesis for multiple domains. In Pro-
ceedings of the IEEE/CVF Conference on Computer
Vision and Pattern Recognition, pages 8188–8197.
Creswell, A., White, T., Dumoulin, V., Arulkumaran, K.,
Sengupta, B., and Bharath, A. A. (2018). Generative
adversarial networks: An overview. IEEE Signal Pro-
cessing Magazine, 35(1):53–65.
Deng, J., Dong, W., Socher, R., Li, L.-J., Li, K., and Fei-
Fei, L. (2009). Imagenet: A large-scale hierarchical
image database. In 2009 IEEE conference on com-
puter vision and pattern recognition, pages 248–255.
Ieee.
Denton, E., Gross, S., and Fergus, R. (2016). Semi-
supervised learning with context-conditional gen-
erative adversarial networks. arXiv preprint
arXiv:1611.06430.
Do, H. P., Guo, Y., Yoon, A. J., and Nayak, K. S. (2020).
Accuracy, uncertainty, and adaptability of automatic
myocardial asl segmentation using deep cnn. Mag-
netic resonance in medicine, 83(5):1863–1874.
Gal, Y. and Ghahramani, Z. (2016). Dropout as a bayesian
approximation: Representing model uncertainty in
deep learning. In international conference on machine
learning, pages 1050–1059. PMLR.
Gao, B.-B., Xing, C., Xie, C.-W., Wu, J., and Geng, X.
(2017). Deep label distribution learning with label
ambiguity. IEEE Transactions on Image Processing,
26(6):2825–2838.
Geng, X. (2016). Label distribution learning. IEEE
Transactions on Knowledge and Data Engineering,
28(7):1734–1748.
Goodfellow, I., Pouget-Abadie, J., Mirza, M., Xu, B.,
Warde-Farley, D., Ozair, S., Courville, A., and Ben-
gio, Y. (2014). Generative adversarial nets. Advances
in neural information processing systems, 27.
Harang, R. and Rudd, E. M. (2018). Towards principled un-
certainty estimation for deep neural networks. arXiv
preprint arXiv:1810.12278.
He, K., Zhang, X., Ren, S., and Sun, J. (2016). Deep resid-
ual learning for image recognition. In Proceedings of
the IEEE conference on computer vision and pattern
recognition, pages 770–778.
Hendrycks, D. and Gimpel, K. (2016). A baseline for de-
tecting misclassified and out-of-distribution examples
in neural networks. arXiv preprint arXiv:1610.02136.
Hendrycks, D., Mazeika, M., and Dietterich, T. (2018).
Deep anomaly detection with outlier exposure. arXiv
preprint arXiv:1812.04606.
H
¨
ullermeier, E. and Beringer, J. (2006). Learning from am-
biguously labeled examples. Intelligent Data Analy-
sis, 10(5):419–439.
Iscen, A., Tolias, G., Avrithis, Y., and Chum, O. (2019).
Label propagation for deep semi-supervised learning.
In Proceedings of the IEEE/CVF Conference on Com-
puter Vision and Pattern Recognition, pages 5070–
5079.
Jiang, B., Zhang, Z., Lin, D., Tang, J., and Luo,
B. (2019). Semi-supervised learning with graph
learning-convolutional networks. In Proceedings of
the IEEE/CVF Conference on Computer Vision and
Pattern Recognition, pages 11313–11320.
Kabir, H. D., Khosravi, A., Hosen, M. A., and Nahavandi,
S. (2018). Neural network-based uncertainty quantifi-
cation: A survey of methodologies and applications.
IEEE access, 6:36218–36234.
Karras, T., Aila, T., Laine, S., and Lehtinen, J. (2017). Pro-
gressive growing of gans for improved quality, stabil-
ity, and variation. arXiv preprint arXiv:1710.10196.
Karras, T., Laine, S., and Aila, T. (2019). A style-based
generator architecture for generative adversarial net-
works. In Proceedings of the IEEE/CVF Conference
on Computer Vision and Pattern Recognition, pages
4401–4410.
Kim, H., Choi, Y., Kim, J., Yoo, S., and Uh, Y. (2021).
Stylemapgan: Exploiting spatial dimensions of latent
in gan for real-time image editing. arXiv preprint
arXiv:2104.14754.
Lakshminarayanan, B., Pritzel, A., and Blundell, C.
(2016). Simple and scalable predictive uncertainty
estimation using deep ensembles. arXiv preprint
arXiv:1612.01474.
Liu, B., Wu, Z., Hu, H., and Lin, S. (2019). Deep metric
transfer for label propagation with limited annotated
data. In Proceedings of the IEEE/CVF International
Conference on Computer Vision Workshops, pages 0–
0.
Nair, T., Precup, D., Arnold, D. L., and Arbel, T. (2020).
Exploring uncertainty measures in deep networks for
ICAART 2022 - 14th International Conference on Agents and Artificial Intelligence
550