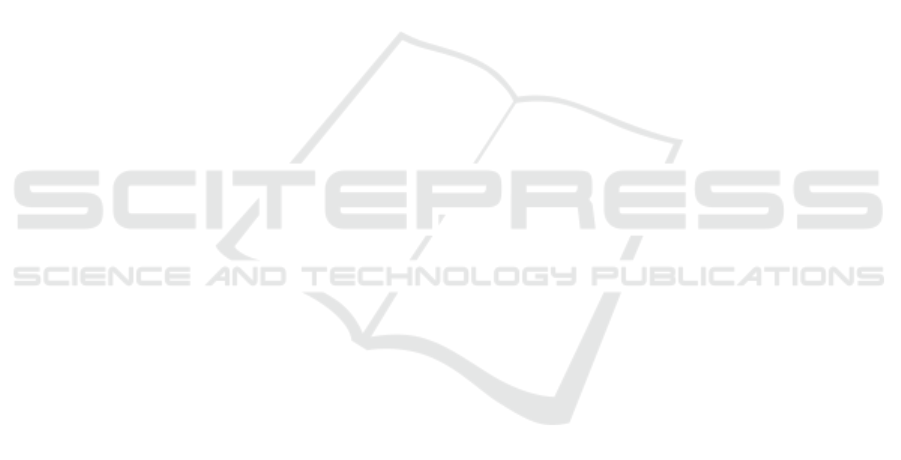
Allam, Z. and Dhunny, Z. A. (2019). On big data, artificial
intelligence and smart cities. Cities, 89:80–91.
Ancona, M., Ceolini, E.,
¨
Oztireli, C., and Gross, M. (2017).
Towards better understanding of gradient-based at-
tribution methods for deep neural networks. arXiv
preprint arXiv:1711.06104.
Ancona, M., Ceolini, E.,
¨
Oztireli, C., and Gross, M. (2019).
Gradient-based attribution methods. In Explainable
AI: Interpreting, Explaining and Visualizing Deep
Learning, pages 169–191. Springer.
Bagnall, A., Lines, J., Vickers, W., and Keogh, E. (2021).
The uea & ucr time series classification repository.
Benesty, J., Chen, J., Huang, Y., and Cohen, I. (2009).
Pearson correlation coefficient. In Noise reduction in
speech processing, pages 1–4. Springer.
Bibal, A., Lognoul, M., de Streel, A., and Fr
´
enay, B. (2020).
Impact of legal requirements on explainability in ma-
chine learning. arXiv preprint arXiv:2007.05479.
Crabb
´
e, J. and van der Schaar, M. (2021). Explaining time
series predictions with dynamic masks. In Proceed-
ings of the 38-th International Conference on Machine
Learning (ICML 2021). PMLR.
Das, A. and Rad, P. (2020). Opportunities and challenges
in explainable artificial intelligence (xai): A survey.
arXiv preprint arXiv:2006.11371.
Do
ˇ
silovi
´
c, F. K., Br
ˇ
ci
´
c, M., and Hlupi
´
c, N. (2018). Ex-
plainable artificial intelligence: A survey. In 2018 41st
International convention on information and commu-
nication technology, electronics and microelectronics
(MIPRO), pages 0210–0215. IEEE.
Fisher, A., Rudin, C., and Dominici, F. (2019). All mod-
els are wrong, but many are useful: Learning a vari-
able’s importance by studying an entire class of pre-
diction models simultaneously. J. Mach. Learn. Res.,
20(177):1–81.
Huber, T., Limmer, B., and Andr
´
e, E. (2021). Benchmark-
ing perturbation-based saliency maps for explaining
deep reinforcement learning agents. arXiv preprint
arXiv:2101.07312.
Ivanovs, M., Kadikis, R., and Ozols, K. (2021).
Perturbation-based methods for explaining deep neu-
ral networks: A survey. Pattern Recognition Letters.
Karliuk, M. (2018). Ethical and legal issues in artificial
intelligence. International and Social Impacts of Arti-
ficial Intelligence Technologies, Working Paper, (44).
Lundberg, S. M. and Lee, S.-I. (2017). A unified approach
to interpreting model predictions. In Proceedings of
the 31st international conference on neural informa-
tion processing systems, pages 4768–4777.
Mitchell, R., Cooper, J., Frank, E., and Holmes, G. (2021).
Sampling permutations for shapley value estimation.
arXiv preprint arXiv:2104.12199.
Myers, L. and Sirois, M. J. (2004). Spearman correla-
tion coefficients, differences between. Encyclopedia
of statistical sciences, 12.
Nielsen, I. E., Rasool, G., Dera, D., Bouaynaya, N.,
and Ramachandran, R. P. (2021). Robust ex-
plainability: A tutorial on gradient-based attribution
methods for deep neural networks. arXiv preprint
arXiv:2107.11400.
Niwattanakul, S., Singthongchai, J., Naenudorn, E., and
Wanapu, S. (2013). Using of jaccard coefficient for
keywords similarity. In Proceedings of the interna-
tional multiconference of engineers and computer sci-
entists, volume 1, pages 380–384.
Perc, M., Ozer, M., and Hojnik, J. (2019). Social and juristic
challenges of artificial intelligence. Palgrave Commu-
nications, 5(1):1–7.
Peres, R. S., Jia, X., Lee, J., Sun, K., Colombo, A. W.,
and Barata, J. (2020). Industrial artificial intelligence
in industry 4.0-systematic review, challenges and out-
look. IEEE Access, 8:220121–220139.
Ribeiro, M. T., Singh, S., and Guestrin, C. (2016). ”why
should I trust you?”: Explaining the predictions of any
classifier. In Proceedings of the 22nd ACM SIGKDD
International Conference on Knowledge Discovery
and Data Mining, San Francisco, CA, USA, August
13-17, 2016, pages 1135–1144.
Shrikumar, A., Greenside, P., Shcherbina, A., and Kun-
daje, A. (2016). Not just a black box: Learning im-
portant features through propagating activation differ-
ences. arXiv preprint arXiv:1605.01713.
Siddiqui, S. A., Mercier, D., Munir, M., Dengel, A., and
Ahmed, S. (2019). Tsviz: Demystification of deep
learning models for time-series analysis. IEEE Ac-
cess, 7:67027–67040.
Simonyan, K., Vedaldi, A., and Zisserman, A. (2013).
Deep inside convolutional networks: Visualising im-
age classification models and saliency maps. arXiv
preprint arXiv:1312.6034.
Springenberg, J. T., Dosovitskiy, A., Brox, T., and Ried-
miller, M. (2014). Striving for simplicity: The all con-
volutional net. arXiv preprint arXiv:1412.6806.
Sundararajan, M., Taly, A., and Yan, Q. (2017). Axiomatic
attribution for deep networks. In International Confer-
ence on Machine Learning, pages 3319–3328. PMLR.
Vermeire, T., Laugel, T., Renard, X., Martens, D., and De-
tyniecki, M. (2021). How to choose an explainability
method? towards a methodical implementation of xai
in practice. arXiv preprint arXiv:2107.04427.
Yeh, C.-K., Hsieh, C.-Y., Suggala, A., Inouye, D. I., and
Ravikumar, P. K. (2019). On the (in) fidelity and sen-
sitivity of explanations. Advances in Neural Informa-
tion Processing Systems, 32:10967–10978.
Zeiler, M. D. and Fergus, R. (2014). Visualizing and under-
standing convolutional networks. In European confer-
ence on computer vision, pages 818–833. Springer.
Zhang, Q. and Zhu, S.-C. (2018). Visual interpretabil-
ity for deep learning: a survey. arXiv preprint
arXiv:1802.00614.
Time to Focus: A Comprehensive Benchmark using Time Series Attribution Methods
573