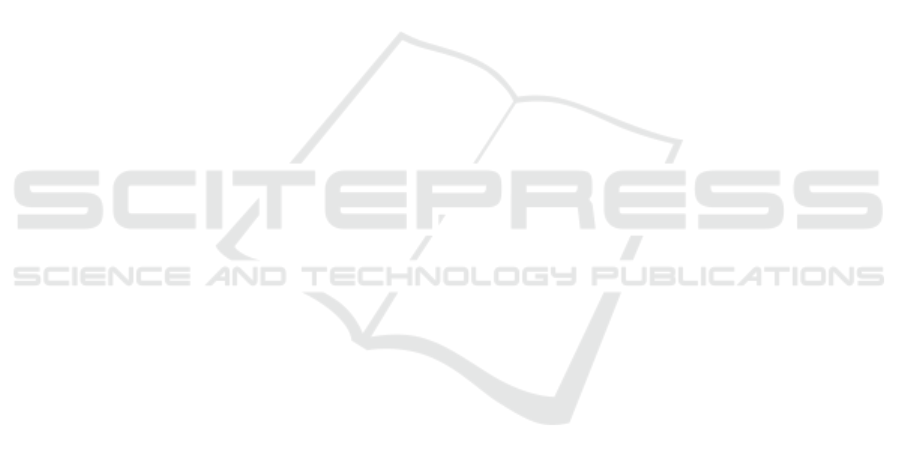
(2019). A short note on the kinetics-700 human ac-
tion dataset. arXiv preprint arXiv:1907.06987.
Carreira, J. and Zisserman, A. (2017). Quo vadis, action
recognition? a new model and the kinetics dataset.
In proceedings of the IEEE Conference on Computer
Vision and Pattern Recognition, pages 6299–6308.
Charfi, I., Miteran, J., Dubois, J., Atri, M., and Tourki, R.
(2012). Definition and performance evaluation of a ro-
bust svm based fall detection solution. In 2012 Eighth
International Conference on Signal Image Technology
and Internet Based Systems, pages 218–224. IEEE.
He, K., Zhang, X., Ren, S., and Sun, J. (2016). Deep resid-
ual learning for image recognition. In Proceedings of
the IEEE conference on computer vision and pattern
recognition, pages 770–778.
Johnson, J. M. and Khoshgoftaar, T. M. (2019). Survey on
deep learning with class imbalance. Journal of Big
Data, 6(1):1–54.
Khan, S. S. and Hoey, J. (2017). Review of fall detection
techniques: A data availability perspective. Medical
engineering & physics, 39:12–22.
Li, D., Zhang, J., Yang, Y., Liu, C., Song, Y.-Z., and
Hospedales, T. M. (2019). Episodic training for do-
main generalization. In Proceedings of the IEEE/CVF
International Conference on Computer Vision, pages
1446–1455.
Li, H., Pan, S. J., Wang, S., and Kot, A. C. (2018). Do-
main generalization with adversarial feature learning.
In Proceedings of the IEEE Conference on Computer
Vision and Pattern Recognition, pages 5400–5409.
Liu, Y., Lu, Z., Li, J., Yao, C., and Deng, Y. (2018). Trans-
ferable feature representation for visible-to-infrared
cross-dataset human action recognition. Complexity,
2018.
Mart
´
ınez-Villase
˜
nor, L., Ponce, H., Brieva, J., Moya-Albor,
E., N
´
u
˜
nez-Mart
´
ınez, J., and Pe
˜
nafort-Asturiano, C.
(2019). Up-fall detection dataset: A multimodal ap-
proach. Sensors, 19(9):1988.
Pe
˜
nafort-Asturiano, C. J., Santiago, N., N
´
u
˜
nez-Mart
´
ınez,
J. P., Ponce, H., and Mart
´
ınez-Villase
˜
nor, L. (2018).
Challenges in data acquisition systems: Lessons
learned from fall detection to nanosensors. In 2018
Nanotechnology for Instrumentation and Measure-
ment (NANOfIM), pages 1–8. IEEE.
Piergiovanni, A., Angelova, A., Toshev, A., and Ryoo, M. S.
(2019). Evolving space-time neural architectures for
videos. In Proceedings of the IEEE international con-
ference on computer vision, pages 1793–1802.
Piergiovanni, A. and Ryoo, M. (2019). Temporal gaussian
mixture layer for videos. In International Conference
on Machine Learning, pages 5152–5161.
Qiao, F., Zhao, L., and Peng, X. (2020). Learning to learn
single domain generalization. In Proceedings of the
IEEE/CVF Conference on Computer Vision and Pat-
tern Recognition, pages 12556–12565.
Ryoo, M. S., Piergiovanni, A., Tan, M., and Angelova,
A. (2019). Assemblenet: Searching for multi-stream
neural connectivity in video architectures. arXiv
preprint arXiv:1905.13209.
Szegedy, C., Vanhoucke, V., Ioffe, S., Shlens, J., and Wo-
jna, Z. (2016). Rethinking the inception architecture
for computer vision. In Proceedings of the IEEE con-
ference on computer vision and pattern recognition,
pages 2818–2826.
Tran, D., Wang, H., Torresani, L., Ray, J., LeCun, Y., and
Paluri, M. (2018a). A closer look at spatiotemporal
convolutions for action recognition. In Proceedings of
the IEEE conference on Computer Vision and Pattern
Recognition, pages 6450–6459.
Tran, T.-H., Le, T.-L., Pham, D.-T., Hoang, V.-N., Khong,
V.-M., Tran, Q.-T., Nguyen, T.-S., and Pham, C.
(2018b). A multi-modal multi-view dataset for human
fall analysis and preliminary investigation on modal-
ity. In 2018 24th International Conference on Pattern
Recognition (ICPR), pages 1947–1952. IEEE.
Vadivelu, S., Ganesan, S., Murthy, O. R., and Dhall, A.
(2016). Thermal imaging based elderly fall detection.
In Asian Conference on Computer Vision, pages 541–
553. Springer.
Wang, H. and Schmid, C. (2013). Action recognition with
improved trajectories. In Proceedings of the IEEE
international conference on computer vision, pages
3551–3558.
Wang, Y., Long, M., Wang, J., and Yu, P. S. (2017).
Spatiotemporal pyramid network for video action
recognition. In Proceedings of the IEEE conference
on Computer Vision and Pattern Recognition, pages
1529–1538.
Weinland, D., Ronfard, R., and Boyer, E. (2006). Free
viewpoint action recognition using motion history vol-
umes. Computer vision and image understanding,
104(2-3):249–257.
Zoetgnande, Y. W. K., Cormier, G., Fougeres, A.-J., and
Dillenseger, J.-L. (2020). Sub-pixel matching method
for low-resolution thermal stereo images. Infrared
Physics & Technology, 105:103161.
Zoetgnand
´
e, Y. W. K., Foug
`
eres, A.-J., Cormier, G., and
Dillenseger, J.-L. (2019). Robust low resolution ther-
mal stereo camera calibration. In Eleventh Interna-
tional Conference on Machine Vision (ICMV 2018),
volume 11041, page 110411D. International Society
for Optics and Photonics.
Domain Generalization for Activity Recognition: Learn from Visible, Infer with Thermal
729