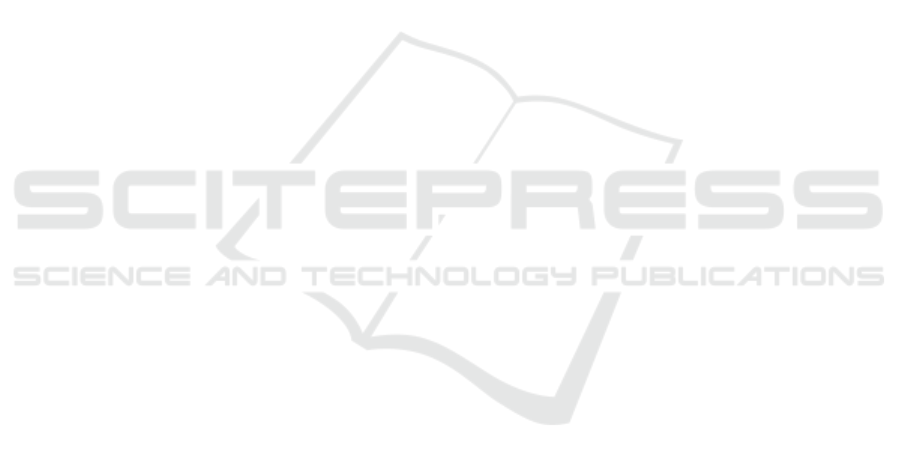
Biran, O. and Cotton, C. (2017). Explanation and justifica-
tion in machine learning: A survey. In Proceedings of
the IJCAI’17 Workshop on Explainable Artificial In-
telligence (XAI’17), pages 8–13.
Chakraborti, T., Sreedharan, S., and Kambhampati, S.
(2020). The emerging landscape of explainable auto-
mated planning & decision making. In Proceedings of
the 29th International Joint Conference on Artificial
Intelligence (IJCAI’20), pages 4803–4811.
Cheng, H.-F. et al. (2019). Explaining decision-making
algorithms through UI: Strategies to help non-expert
stakeholders. In Proceedings of the 2019 CHI Con-
ference on Human Factors in Computing Systems
(CHI’19), pages 1–12.
Domshlak, C. and Hoffmann, J. (2006). Fast probabilistic
planning through weighted model counting. In Pro-
ceedings of the 16th International Conference on Au-
tomated Planning and Scheduling (ICAPS’06), pages
243–252.
Geffner, H. and Bonet, B. (2013). A Concise Introduction to
Models and Methods for Automated Planning. Mor-
gan & Claypool Publishers.
Guo, X., Shen, Z., Zhang, Y., and Wu, T. (2019). Review
on the application of artificial intelligence in smart
homes. Smart Cities, 2(3):402–420.
Herzen, J. et al. (2021). Darts: User-friendly modern ma-
chine learning for time series. arXiv:2110.03224.
Hoffman, R. R., Mueller, S. T., Klein, G., and Litman, J.
(2018). Metrics for explainable AI: Challenges and
prospects. arXiv:1812.04608.
Hussein, A., Gaber, M. M., Elyan, E., and Jayne, C. (2017).
Imitation learning: A survey of learning methods.
ACM Computing Surveys, 50(2):21.
Hyndman, R. J. and Athanasopoulos, G. (2018). Forecast-
ing: principles and practice. OTexts, 2nd edition.
Kraus, S. et al. (2020). AI for explaining decisions in multi-
agent environments. In Proceedings of the AAAI Con-
ference on Artificial Intelligence (AAAI’20), pages
13534–13538.
Langer, M. et al. (2021). What do we want from explain-
able artificial intelligence (XAI)?–a stakeholder per-
spective on XAI and a conceptual model guiding in-
terdisciplinary XAI research. Artificial Intelligence,
296:103473.
Lipton, Z. C. (2018). The mythos of model interpretability:
In machine learning, the concept of interpretability is
both important and slippery. ACM Queue, 16(3):31–
57.
Little, I. and Thi
´
ebaux, S. (2007). Probabilistic planning vs.
replanning. In Proceedings of the ICAPS Workshop on
Planning Competitions: Past, Present, and Future.
L
¨
oning, M., Bagnall, A., Ganesh, S., Kazakov, V., Lines, J.,
and Kir
´
aly, F. J. (2019). sktime: A unified interface
for machine learning with time series. In Proceed-
ings of the NeurIPS Workshop on Systems for Machine
Learning.
Mekuria, D. N., Sernani, P., Falcionelli, N., and Dragoni,
A. F. (2019). Smart home reasoning systems: a sys-
tematic literature review. Journal of Ambient Intelli-
gence and Humanized Computing, pages 1–18.
Miller, T. (2019). Explanation in artificial intelligence: In-
sights from the social sciences. Artificial Intelligence,
267:1–38.
Pedregosa, F. et al. (2011). Scikit-learn: Machine learning
in Python. Journal of Machine Learning Research,
12:2825–2830.
Pitropakis, N., Panaousis, E., Giannetsos, T., Anastasiadis,
E., and Loukas, G. (2019). A taxonomy and survey of
attacks against machine learning. Computer Science
Review, 34:100199.
Rogers, A., Ghosh, S., Wilcock, R., and Jennings, N. R.
(2013). A scalable low-cost solution to provide per-
sonalised home heating advice to households. In
Proceedings of the 5th ACM Workshop on Embedded
Systems For Energy-Efficient Buildings (BuildSys’13),
pages 1–8.
Rogers, A., Maleki, S., Ghosh, S., and Jennings, N. R.
(2011). Adaptive home heating control through
gaussian process prediction and mathematical pro-
gramming. In Proceedings of the 2nd International
Workshop on Agent Technology for Energy Systems
(ATES’11).
Russell, S. J. and Norvig, P. (2020). Artificial Intelligence:
A Modern Approach. Prentice Hall, 4th edition.
Shann, M., Alan, A., Seuken, S., Costanza, E., and Ram-
churn, S. D. (2017). Save money or feel cozy?: A
field experiment evaluation of a smart thermostat that
learns heating preferences. In Proceedings of the 16th
Conference on Autonomous Agents and Multiagent
Systems (AAMAS’17), pages 1008–1016.
Shann, M. and Seuken, S. (2013). An active learning ap-
proach to home heating in the smart grid. In Proceed-
ings of the 23rd International Joint Conference on Ar-
tificial Intelligence (IJCAI’13), pages 2892–2899.
Shann, M. and Seuken, S. (2014). Adaptive home heat-
ing under weather and price uncertainty using gps and
mdps. In Proceedings of the 13th International Con-
ference on Autonomous Agents and Multiagent Sys-
tems (AAMAS’14), pages 821–828.
Yang, R. and Newman, M. W. (2013). Learning from a
learning thermostat: lessons for intelligent systems for
the home. In Proceedings of the 2013 ACM Interna-
tional Joint Conference on Pervasive and Ubiquitous
Computing (UbiComp’13), pages 93–102.
ICAART 2022 - 14th International Conference on Agents and Artificial Intelligence
784