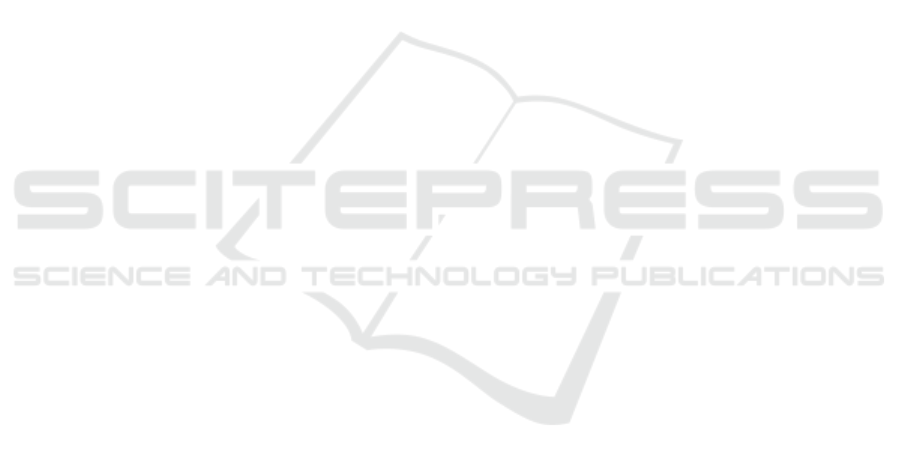
we shortlisted some papers that purely used Conta-
gio dataset and compared their results in the Table
7. Eventhough the specified research works solely re-
lied on Contagio dataset to evaluate their research, the
evaluation method was not specified. Based on the
results, it was identified that our approach achieves a
higher testing accuracy than the other two researches.
5.6 Evaluating the Proposed Model
using New Dataset
As the new dataset proved to be more reliable than
Contagio dataset based on a set of criteria, we decided
to evaluate our model on our own dataset to validate
its effectiveness further. We can observe the results
in the Table 5 and the comparison of the individual
scores of each classifier in Table 4. From these re-
sults, it can be seen that our proposed model outper-
forms each of the individual scores, which verifies the
robustness and validity of our design.
6 CONCLUSIONS AND FUTURE
WORKS
Malware PDF files are a severe Cyber risk in to-
day’s world. Analyzing the PDF complex structure
and powerful features, we can conclude that attackers
can deliver malware in multiple ways. Lack of user
awareness coupled with the ineffectiveness of com-
mon anti-viruses has increased this risk even more.
Several solutions have been proposed for PDF
malware detection, with their strengths and weak-
nesses. In this research, we proposed a stacking-
based learning model to detect malicious PDF files.
We demonstrated our solution’s effectiveness through
several experimentation by extracting a set of 28 rep-
resentative features and stacking three different al-
gorithms. Furthermore, we generated a new dataset
(Evasive-PDFMal2022) according to specific data
quality cri-teria that lead to more reliable results and
better rep-resents the real-world distribution of benign
and ma-licious PDF files.
ACKNOWLEDGEMENTS
We thank the Lockheed Martin Cybersecurity Re-
search Fund (LMCRF) to support this project.
REFERENCES
Blonce, A., Filiol, E., and Frayssignes, L. (2008). Portable
document format (pdf) security analysis and malware
threats. In Presentations of Europe BlackHat 2008
Conference.
Brandis, R. and Steller, L. (2012). Threat modelling adobe
pdf. Technical report.
Carmony, C., Hu, X., Yin, H., Bhaskar, A. V., and Zhang,
M. (2016). Extract me if you can: Abusing pdf parsers
in malware detectors. In NDSS.
Corona, I., Maiorca, D., Ariu, D., and Giacinto, G.
(2014). Lux0r: Detection of malicious pdf-embedded
javascript code through discriminant analysis of api
references. In workshop on artificial intelligent and
security workshop, pages 47–57.
Cross, J. S. and Munson, M. A. (2011). Deep pdf parsing
to extract features for detecting embedded malware.
Sandia National Labs, Albuquerque, New Mexico, Un-
limited Release SAND2011-7982.
Cuan, B., Damien, A., Delaplace, C., and Valois, M. (2018).
Malware detection in pdf files using machine learning.
Cui, Y., Sun, Y., Luo, J., Huang, Y., Zhou, Y., and Li, X.
(2020). Mmpd: A novel malicious pdf file detector
for mobile robots. IEEE Sensors Journal.
Fettaya, R. and Mansour, Y. (2020). Detecting malicious
pdf using cnn. arXiv preprint arXiv:2007.12729.
Itabashi, K. (2011). Portable document format malware.
Symantec white paper.
Jeong, Y.-S., Woo, J., and Kang, A. R. (2019). Malware
detection on byte streams of pdf files using convolu-
tional neural networks. Security and Communication
Networks, 2019.
Li, Y., Wang, Y., Wang, Y., Ke, L., and Tan, Y.-a. (2020).
A feature-vector generative adversarial network for
evading pdf malware classifiers. Information Sci-
ences, 523:pp. 38–48.
Liu, D., Wang, H., and Stavrou, A. (2014). Detecting
malicious javascript in pdf through document instru-
mentation. In 2014 44th Annual IEEE/IFIP Interna-
tional Conference on Dependable Systems and Net-
works, pages 100–111. IEEE.
Maiorca, D., Ariu, D., Corona, I., and Giacinto, G. (2015).
A structural and content-based approach for a precise
and robust detection of malicious pdf files. In 2015
international conference on information systems se-
curity and privacy (icissp), pages 27–36. IEEE.
Maiorca, D., Biggio, B., and Giacinto, G. (2019). Towards
adversarial malware detection: Lessons learned from
pdf-based attacks. ACM Computing Surveys (CSUR),
52(4):pp. 1–36.
Nissim, N., Cohen, A., Glezer, C., and Elovici, Y. (2015).
Detection of malicious pdf files and directions for en-
hancements: A state-of-the art survey. Computers &
Security, 48:246–266.
Stevens, D. (2011). Malicious pdf documents explained.
IEEE Security & Privacy, 9(1):80–82.
Torres, J. and De Los Santos, S. (2018). Malicious pdf doc-
uments detection using machine learning techniques.
PDF Malware Detection based on Stacking Learning
569