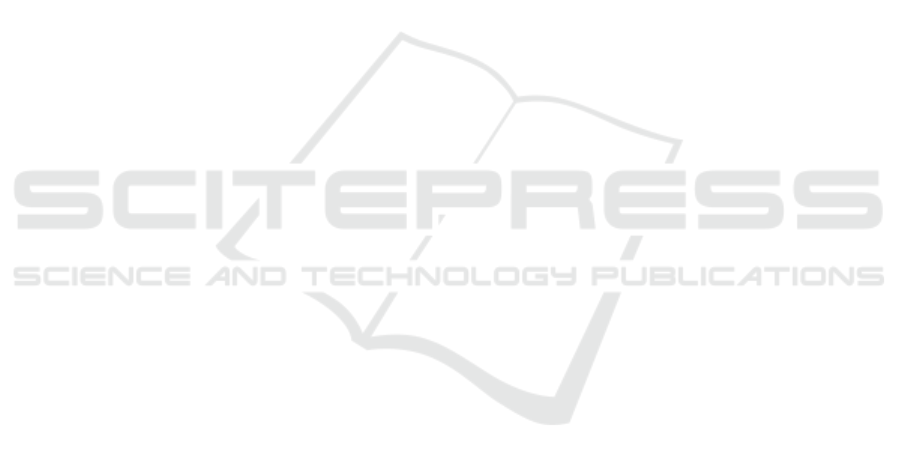
example, due to the structures of CP-nets are inter-
pretative, users can easily express their attitude to-
wards the effect of preference completion, then how
to make agents interact with users efficiently to im-
prove the accuracy of completion of preference is one
of the most important problems should be address in
the future.
ACKNOWLEDGEMENTS
The works described in this paper are supported by
the National Natural Science Foundation of China un-
der Grant Nos. 62006085, 61772210 and U1911201;
Guangdong Province Universities Pearl River Scholar
Funded Scheme (2018); Project of Science and Tech-
nology in Guangzhou in China under Grant Nos.
202007040006 and 202102020948; Natural Science
Foundation of Guangdong Province in China under
Grant No. 2018A030310529; Project of Department
of Education of Guangdong Province in China under
Grant No. 2017KQNCX048.
REFERENCES
Amor, N. B., Dubois, D., Gouider, H., and Prade, H.
(2016). Graphical models for preference representa-
tion: An overview. In Scalable Uncertainty Manage-
ment, pages 96–111.
Aydo
˘
gan, R., Baarslag, T., Hindriks, K. V., Jonker, C. M.,
and Yolum, P. (2015). Heuristics for using CP-nets
in utility-based negotiation without knowing utilities.
Knowledge and Information Systems, 45(2):357–388.
Aydo
˘
gan, R. and Yolum, P. (2010). Effective negotiation
with partial preference information. In Proceedings
of the 9th International Conference on Autonomous
Agents and Multiagent Systems, pages 1605–1606.
Baarslag, T. (2016). Exploring the strategy space of negoti-
ating agents: A framework for bidding, learning and
accepting in automated negotiation. Springer.
Baarslag, T. and Gerding, E. H. (2015). Optimal incre-
mental preference elicitation during negotiation. In
Twenty-Fourth International Joint Conference on Ar-
tificial Intelligence, page 3–9.
Baarslag, T. and Kaisers, M. (2017). The value of infor-
mation in automated negotiation: A decision model
for eliciting user preferences. In Proceedings of the
16th Conference on Autonomous Agents and MultiA-
gent Systems, pages 391–400.
Boutilier, C., Brafman, R. I., Domshlak, C., Hoos, H. H.,
and Poole, D. (2004). CP-nets: A tool for representing
and reasoning with conditional ceteris paribus prefer-
ence statements. Journal of Artificial Intelligence Re-
search, 21:135–191.
Dimopoulos, Y., Michael, L., and Athienitou, F. (2009).
Ceteris paribus preference elicitation with predic-
tive guarantees. In Proceedings of the 21st Inter-
national Joint Conference on Artificial Intelligence,
pages 1890–1895.
Emerson, P. (2013). The original borda count and partial
voting. Social Choice and Welfare, 40(2):353–358.
Faratin, P., Sierra, C., and Jennings, N. R. (1998). Ne-
gotiation decision functions for autonomous agents.
Robotics and Autonomous Systems, 24(3-4):159–182.
Goldsmith, J., Lang, J., Truszczynski, M., and Wilson, N.
(2008). The computational complexity of dominance
and consistency in CP-nets. Journal of Artificial Intel-
ligence Research, 33:403–432.
Haddawy, P., Ha, V., Restificar, A., Geisler, B., and
Miyamoto, J. (2003). Preference elicitation via the-
ory refinement. The Journal of Machine Learning Re-
search, 4:317–337.
Jennings, N. R., Faratin, P., Lomuscio, A. R., Parsons,
S., Wooldridge, M. J., and Sierra, C. (2001). Au-
tomated negotiation: Prospects, methods and chal-
lenges. Group Decision and Negotiation, 10(2):199–
215.
Kumar, S. and Mastorakis, N. E. (2009). A utility
based, multi-attribute negotiation approach for seman-
tic web services. WSEAS Transaction on Computers,
8(11):1733–1748.
Lafage, C. and Lang, J. (2000). Logical representation of
preferences for group decision making. In Proceed-
ings of the Seventh International Conference on Prin-
ciples of Knowledge Representation and Reasoning,
pages 457–468.
Liu, Z., Zhong, Z., Li, K., and Zhang, C. (2018). Struc-
ture learning of conditional preference networks based
on dependent degree of attributes from preference
database. IEEE Access, 6:27864–27872.
Morgado, A., Dodaro, C., and Marques-Silva, J. (2014).
Core-guided maxsat with soft cardinality constraints.
In International Conference on Principles and Prac-
tice of Constraint Programming, pages 564–573.
Springer.
Sanchez-Anguix, V., Tunalı, O., Aydo
˘
gan, R., and Julian, V.
(2021). Can social agents efficiently perform in auto-
mated negotiation? Applied Sciences, 11(13):6022.
S
¨
orensson, N. and Een, N. (2005). Minisat v1. 13-a
sat solver with conflict-clause minimization. SAT,
2005(53):1–2.
Towell, G. G. and Shavlik, J. W. (1994). Knowledge-based
artificial neural networks. Artificial intelligence, 70(1-
2):119–165.
Tsimpoukis, D., Baarslag, T., Kaisers, M., and Paterakis,
N. (2018). Automated negotiations under user prefer-
ence uncertainty: A linear programming approach. In
Agreement Technologies - 6th International Confer-
ence, AT 2018, Revised Selected Papers, pages 115–
129.
ICAART 2022 - 14th International Conference on Agents and Artificial Intelligence
390