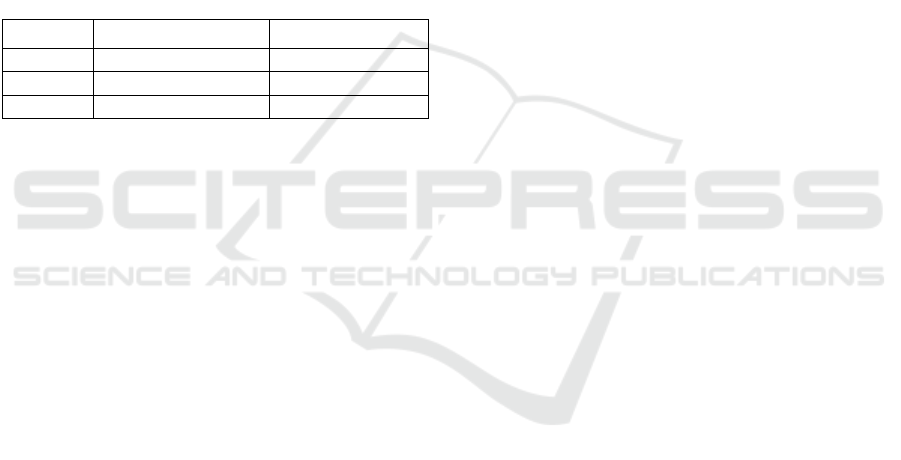
3.2 DUV Range
In the DUV, 8 features were included for each sample
into the model: the integral under the curve of each
of the 4 fluorophores and 4 ratio: TYR/NADH,
COL/NADH, TRY/COL, TYR/TRY. The model was
trained with 90797 samples (80% of the database) and
evaluated on the remaining 38914 samples (20%).
The 3 models achieve very similar score: 87% for
DT, 89% for RF and 88% for GBDT (Table 4).
We also studied the importance of each feature for
the classification. The features the more useful to
build the models are integral of collagene (COL) and
of tryptophane (TRY). Here also, training the model
with only those parameters leads to poor results.
Table 4: Classification on the test database in the DUV for
2 and 16 features using Decision Tree, Random Forest and
Gradient Boosting Decision Tree.
4 DISCUSSION AND
CONCLUSION
For the first time, we used ML methods on
spectroscopic data from brain tissue samples in order
to discriminate tumoral from non tumoral tissues
using quantitative optical parameters. This
preliminary study suggests that combining several
features into a ML model significantly improve the
classification.
We could not combine DUV and visible data in
the same model because we did not have the exact
position of the spectral records and we could not
establish the correspondence between two samples.
Such combination will be upgraded in the next
database. As the most discriminant features in DUV
and visible don’t come from the same fluorophores,
an improved result can be expected because it can be
assumed that useful information is complementary.
Indeed, though the input number of samples given
to the ML model is very high, they come from a
limited number of histological specimens and it is
necessary to confirm these results on more
specimens.
In this study, we only take into account spectral
data. Work is in progress to take advantage of other
available information such as fluorescence lifetime
and fluorescence and SHG optical images.
ACKNOWLEDGEMENTS
This work is financially supported by ITMO Cancer
AVIESAN (Alliance Nationale pour les Sciences de la Vie
et de la Santé, National Alliance for Life Sciences &
Health) within the framework of the Cancer Plan for
MEVO & IMOP projects, by CNRS with “Dfi
instrumental” grant, by ligue nationale contre le cancer
(LNCC) and the Institut National de Physique Nuclaire et
de Physique des Particules (IN2P3).
We would like to thank Synchrotron SOLEIL for the
accorded beam-time and for all staff members of DISCO
beamline for their help as well their contribution in the
scientific discussion. We would like also to thank the
Delegation for Clinical Research and Innovation (DRCI)
and the Biological Resources Center (CRB) of Sainte-Anne
hospital center for providing the samples.
We would to thank also the neurosurgeons at Sainte-
Anne hospital (M Zanello, E Dezamis, C Benevello, G Zah-
Bi, A Roux) for providing the surgical specimens.
REFERENCES
C. Buckner, et al., (2007) ‘Central Nervous System
Tumors’, Mayo Clin. Proc., vol. 82, no. 10, pp. 1271–
1286.
D. N. Louis et al., (2016), ‘The 2016 World Health
Organization Classification of Tumors of the Central
Nervous System: a summary’, Acta Neuropathol.
(Berl.), vol. 131, no. 6, pp. 803–820.
T. Wilson, M. Karajannis, and D. Harter, (2014),
‘Glioblastoma multiforme: State of the art and future
therapeutics’, Surg. Neurol. Int., vol. 5, no. 1, p. 64.
P. L. Kubben, et al., (2011), ‘Intraoperative MRI-guided
resection of glioblastoma multiforme: a systematic
review’, Lancet Oncol., vol. 12, no. 11, pp. 1062–1070,
G. Unsgaard et al., (2006), ‘Intra-operative 3D ultrasound
in neurosurgery’, Acta Neurochir. (Wien), vol. 148, no.
3, pp. 235–253
A. Ibrahim, et al., ‘Characterization of fiber ultrashort pulse
delivery for nonlinear endomicroscopy’, (2016), Opt.
Express, vol. 24, no. 12, p. 12515.
M. Sibai et al., (2018), ‘The Impact of Compressed
Femtosecond Laser Pulse Durations on Neuronal
Tissue Used for Two-Photon Excitation Through an
Endoscope’, Sci. Rep., vol. 8, no. 1, p. 11124.
F. Poulon et al., (2018), ‘Multimodal Analysis of Central
Nervous System Tumor Tissue Endogenous
Fluorescence With Multiscale Excitation’, Front.
Phys., vol. 6.
F. Poulon et al., (2018), ‘Real-time Brain Tumor imaging
with endogenous fluorophores: a diagnosis proof-of-
concept study on fresh human samples’, Sci. Rep., vol.
8, no. 1, p. 14888.
H. Mehidine et al., (2019), ‘Optical Signatures Derived
From Deep UV to NIR Excitation Discriminates
Healthy Samples From Low and High Grades Glioma’,
Sci. Rep., vol. 9, no. 1, p. 8786.
Classification of Brain Tumour Tissues in Human Patients using Machine Learning
57