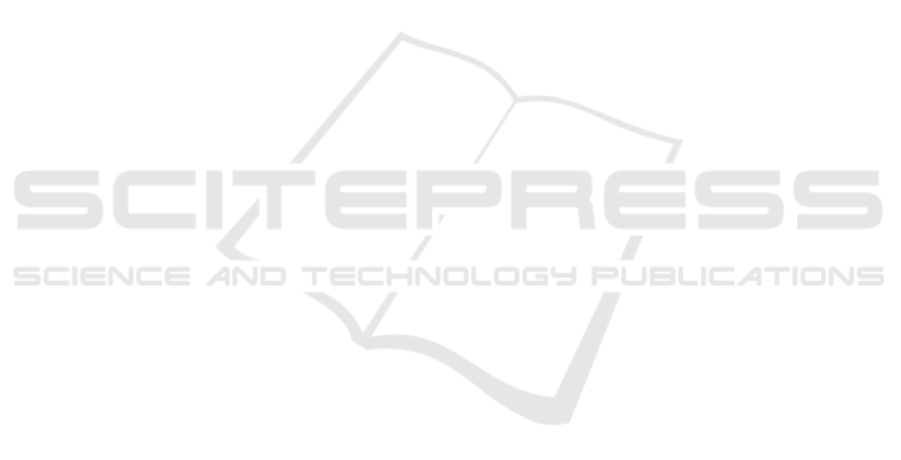
ation is barely influencing the face verification per-
formance: comparisons between good and bad qual-
ity samples output higher or lower similarity scores
alike. This behaviour is an indicator that the used face
descriptors are robust enough to the slight head pose
variation across Melania’s image samples.
Among the selected quality metrics, one could af-
firm that brightness, face luminance and occlusion by
sunglasses are the most relevant quality metrics af-
fecting the face verification performance, and to a
lesser degree with exposure, contrast and femininity.
Brightness, face luminance, exposure and contrast be-
ing all related to the illumination conditions during
the image acquisition depict the same behaviour of the
affinity matrix that could be seen in figures 3a, 3b, 3c
and 3d. The slight shift between the genuine and
imposter score distributions deduced from Melania
Trump image samples, observed in subsection 2.3, is
certainly due to the fact that the set of the ‘real’ Mela-
nia contains overall images of better quality compared
to the set of the alleged ‘body double’, and obvi-
ously not because of the existence of a Melania Trump
‘body double’.
4 CONCLUSION
This paper proposes a first attempt to explore whether
automatic face verification can help in fighting the
spread of conspiracy theories and if it could be used as
an information verification tool. A further extensive
study should be performed on a wider set of identities
and their associated body doubles. In this paper, dif-
ferent state-of-the-art descriptors of face recognition
were used to compare the ‘real’ Melania image set to
the the images of the alleged ‘body double’. Accord-
ing to the proposed study, the answer to the question
‘Does Melania Trump have a body double?’ is defi-
nitely a No. In the studied case, automatic face ver-
ification invalidates the polemical conspiracy theory
claiming the existence of a replacement of Melania
Trump during the presidency term of Donald Trump.
This disclaimer could only be reliable if we presume
that the provided face image samples were not priorly
manipulated. Recently, a new type of image manipu-
lation intended to trick the deep leaning based mod-
els into delivering an erroneous output. This type of
image manipulation, called adversarial attacks, is per-
formed by adding an adversarial perturbation that can
be imperceptible to the human eye (Bisogni et al.,
2021). It could be imagined, for instance, that a re-
placement of Melania Trump exists indeed and that
the associated image samples were manipulated in a
way that the replacement will be recognized as Mela-
nia Trump.
REFERENCES
(2019). Mobilesqueezenet: face reidentification retail 0095.
t.ly/MqxX.
Antipov, G., Berrani, S.-A., and Dugelay, J.-L. (2016). Min-
imalistic cnn-based ensemble model for gender pre-
diction from face images. Pattern recognition letters.
Bisogni, C., Cascone, L., Dugelay, J.-L., and Pero, C.
(2021). Adversarial attacks through architectures and
spectra in face recognition. Pattern Recognition Let-
ters.
Deng, J., Guo, J., Ververas, E., Kotsia, I., and Zafeiriou, S.
(2020). Retinaface: Single-shot multi-level face local-
isation in the wild. In Proceedings of the IEEE/CVF
Conference on Computer Vision and Pattern Recogni-
tion.
Deng, J., Guo, J., Xue, N., and Zafeiriou, S. (2019). Ar-
cface: Additive angular margin loss for deep face
recognition. In Proceedings of the IEEE/CVF Con-
ference on Computer Vision and Pattern Recognition,
pages 4690–4699.
Dolhansky, B., Howes, R., Pflaum, B., Baram, N., and Fer-
rer, C. C. (2020). The deepfake detection challenge
(dfdc) dataset. arXiv preprint arXiv:2006.07397.
Gao, X., Li, S. Z., Liu, R., and Zhang, P. (2007). Standard-
ization of face image sample quality. In International
Conference on Biometrics, pages 242–251. Springer.
Howard, A. G., Zhu, M., Chen, B., Kalenichenko, D.,
Wang, W., Weyand, T., Andreetto, M., and Adam,
H. (2017). Mobilenets: Efficient convolutional neu-
ral networks for mobile vision applications. arXiv
preprint arXiv:1704.04861.
Huang, G. B., Ramesh, M., Berg, T., and Learned-Miller,
E. (2007). Labeled faces in the wild: A database for
studying face recognition in unconstrained environ-
ments. Technical Report 07-49, University of Mas-
sachusetts, Amherst.
Liu, W., Wen, Y., Yu, Z., Li, M., Raj, B., and Song, L.
(2017). Sphereface: Deep hypersphere embedding for
face recognition. In Proceedings of the IEEE con-
ference on computer vision and pattern recognition,
pages 212–220.
Mahfoudi, G., Tajini, B., Retraint, F., Morain-Nicolier, F.,
Dugelay, J. L., and Marc, P. (2019). Defacto: Image
and face manipulation dataset. In 27th European Sig-
nal Processing Conference (EUSIPCO).
Mallat, K., Damer, N., Boutros, F., and Dugelay, J.-L.
(2019). Robust face authentication based on dynamic
quality-weighted comparison of visible and thermal-
to-visible images to visible enrollments. In 2019 22th
International Conference on Information Fusion (FU-
SION), pages 1–8.
Mallat, K., Galdi, C., and Dugelay, J.-L. (2017). Evaluation
of the facial makeup impact on femininity appearance
based on automatic prediction. COMET 2017, 2nd
Cosmetic Measurement & Testing Symposium,
Cergy-Pontoise, France.
M
´
endez-V
´
azquez, H., Chang, L., Rizo-Rodr
´
ıguez, D., and
Morales-Gonz
´
alez, A. (2012). Evaluaci
´
on de la cali-
dad de las im
´
agenes de rostros utilizadas para la iden-
Does Melania Trump Have a Body Double from the Perspective of Automatic Face Verification?
743