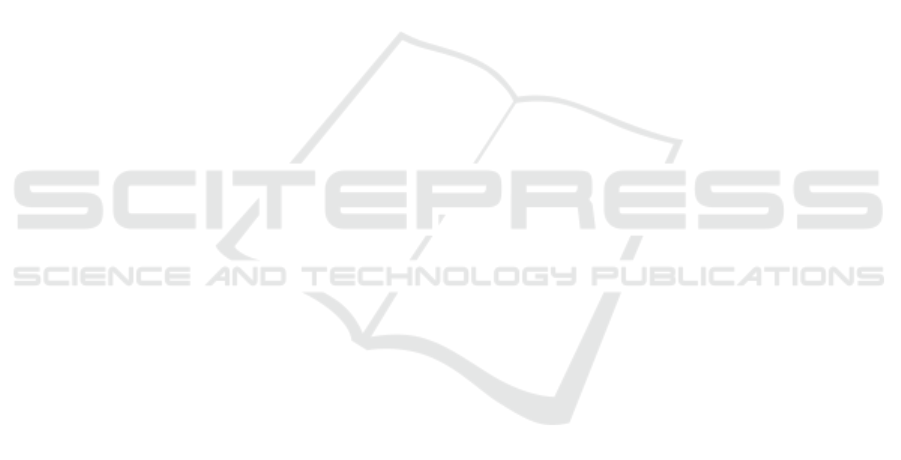
1. For Clear Weather: Lidar-ONLY
2. For Cloudy Weather: Lidar-ONLY
3. For Rainy Weather: Camera-Radar
4. For Snowy Weather: Camera-ONLY
However, given the fact that rain and snow pro-
duce similar types of noise, an assumption can be
made that camera-radar may be the best for snowy
conditions, as well as rain.
5 CONCLUSION
In this paper, various datasets containing various
weather conditions were used and tested on different
modalities for the goal of finding the best sensor con-
figuration for each weather condition. From the eval-
uations of the different sensor modalities, insight as
to which sensors should be used for which weather
conditions has been gained. Now, preparations can be
made for the next step which is to develop a frame-
work based on this knowledge, then an efficient and
safe system for computation on edge devices may be
developed.
Future work opportunities may include the addi-
tion of a model for classifying weather conditions, so
that the decisions can be made based on the model’s
output. A variety of configurations utilizing different
modalities was tested in this paper. However, there
may still be some novel sensors that can be tested
such as, thermal cameras and night-vision cameras.
Also, camera-radar fusion was only tested on clear
and rainy conditions. An opportunity would be to
test this fusion on other conditions. Moreover, this
work resorted to the evaluation of earlier fusion ap-
proaches between sensors(early and middle). Testing
of late-fusion architectures may add more insight as
to which sensor configuration is best-suited to each
weather condition. Finally, it may be beneficial to test
on a large amount of samples.
REFERENCES
Bijelic, M., Gruber, T., and Ritter, W. (2018). Benchmark-
ing image sensors under adverse weather conditions
for autonomous driving. 2018 IEEE Intelligent Vehi-
cles Symposium (IV), pages 1773–1779.
Caesar, H., Bankiti, V., Lang, A. H., Vora, S., Liong, V. E.,
Xu, Q., Krishnan, A., Pan, Y., Baldan, G., and Bei-
jbom, O. (2020). nuscenes: A multimodal dataset
for autonomous driving. 2020 IEEE/CVF Conference
on Computer Vision and Pattern Recognition (CVPR),
pages 11618–11628.
Chen, K., Wang, J., Pang, J., Cao, Y., Xiong, Y., Li, X.,
Sun, S., Feng, W., Liu, Z., Xu, J., Zhang, Z., Cheng,
D., Zhu, C., Cheng, T., Zhao, Q., Li, B., Lu, X., Zhu,
R., Wu, Y., Dai, J., Wang, J., Shi, J., Ouyang, W., Loy,
C. C., and Lin, D. (2019). MMDetection: Open mm-
lab detection toolbox and benchmark. arXiv preprint
arXiv:1906.07155.
Contributors, M. (2020). MMDetection3D: OpenMMLab
next-generation platform for general 3D object detec-
tion. https://github.com/open-mmlab/mmdetection3d.
Everingham, M., Gool, L., Williams, C. K., Winn, J.,
and Zisserman, A. (2010). The pascal visual ob-
ject classes (voc) challenge. Int. J. Comput. Vision,
88(2):303–338.
Geiger, A., Lenz, P., and Urtasun, R. (2012). Are we ready
for autonomous driving? the kitti vision benchmark
suite. In 2012 IEEE Conference on Computer Vision
and Pattern Recognition, pages 3354–3361.
Heinzler, R., Schindler, P., Seekircher, J., Ritter, W., and
Stork, W. (2019). Weather influence and classification
with automotive lidar sensors. In 2019 IEEE Intelli-
gent Vehicles Symposium (IV), pages 1527–1534.
Hendrycks, D. and Dietterich, T. G. (2019). Benchmarking
neural network robustness to common corruptions and
perturbations. ArXiv, abs/1903.12261.
Lee, U., Jung, J., Jung, S., and Shim, D. (2018). Develop-
ment of a self-driving car that can handle the adverse
weather. International Journal of Automotive Tech-
nology, 19:191–197.
Michaelis, C., Mitzkus, B., Geirhos, R., Rusak, E., Bring-
mann, O., Ecker, A. S., Bethge, M., and Brendel, W.
(2019). Benchmarking robustness in object detection:
Autonomous driving when winter is coming. ArXiv,
abs/1907.07484.
Nabati, R. and Qi, H. (2021). Centerfusion: Center-based
radar and camera fusion for 3d object detection. 2021
IEEE Winter Conference on Applications of Computer
Vision (WACV), pages 1526–1535.
Peynot, T., Underwood, J., and Scheding, S. (2009). To-
wards reliable perception for unmanned ground vehi-
cles in challenging conditions. In 2009 IEEE/RSJ In-
ternational Conference on Intelligent Robots and Sys-
tems, pages 1170–1176.
Pitropov, M., Garcia, D., Rebello, J., Smart, M., Wang, C.,
Czarnecki, K., and Waslander, S. L. (2021). Canadian
adverse driving conditions dataset. The International
Journal of Robotics Research, 40:681 – 690.
Ren, S., He, K., Girshick, R. B., and Sun, J. (2015). Faster
r-cnn: Towards real-time object detection with region
proposal networks. IEEE Transactions on Pattern
Analysis and Machine Intelligence, 39:1137–1149.
Sindagi, V. A., Zhou, Y., and Tuzel, O. (2019). Mvx-net:
Multimodal voxelnet for 3d object detection. 2019
International Conference on Robotics and Automation
(ICRA), pages 7276–7282.
Tang, J., Liu, S., Yu, B., and Shi, W. (2019). Pi-edge: A
low-power edge computing system for real-time au-
tonomous driving services. ArXiv, abs/1901.04978.
Yan, Y., Mao, Y., and Li, B. (2018). Second: Sparsely
embedded convolutional detection. Sensors (Basel,
Switzerland), 18.
ICAART 2022 - 14th International Conference on Agents and Artificial Intelligence
792