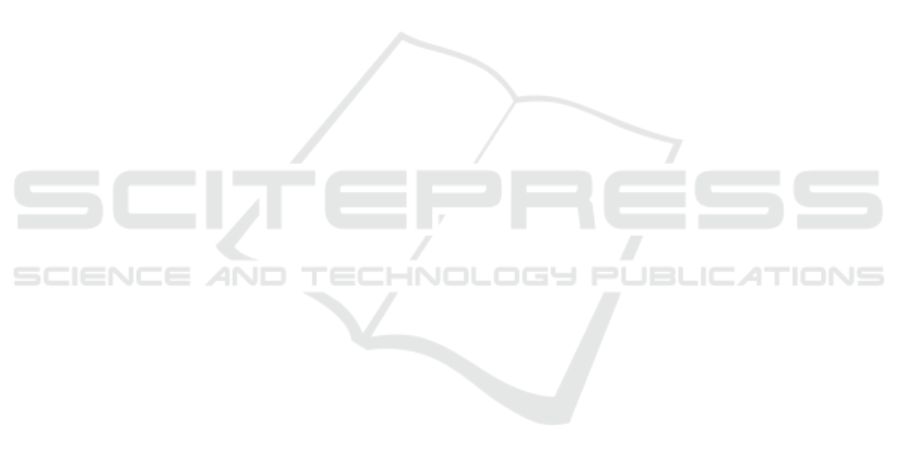
ACKNOWLEDGMENT
This work was supported by the DEFACTO (Auto-
mated detection of digital images falsifications) con-
sortium (UTT, Eurecom and Surys), which partici-
pated in the French challenge DEFALS (DEtection of
FALSifications in images and videos).
REFERENCES
Bayar, B. and Stamm, M. C. (2017a). Augmented con-
volutional feature maps for robust cnn-based cam-
era model identification. In 2017 IEEE International
Conference on Image Processing (ICIP), pages 4098–
4102.
Bayar, B. and Stamm, M. C. (2017b). Design principles of
convolutional neural networks for multimedia foren-
sics. In Media Watermarking, Security, and Forensics.
Bayar, B. and Stamm, M. C. (2018). Towards open set cam-
era model identification using a deep learning frame-
work. In 2018 IEEE International Conference on
Acoustics, Speech and Signal Processing (ICASSP),
pages 2007–2011.
Berthet, A. and Dugelay, J.-L. (2020). A review of data pre-
processing modules in digital image forensics meth-
ods using deep learning. In 2020 IEEE Interna-
tional Conference on Visual Communications and Im-
age Processing (VCIP), pages 281–284.
Berthet, A., Tescari, F., Galdi, C., and Dugelay, J.-L.
(2021). Two-stream convolutional neural network
for image source social network identification. In
2021 International Conference on Cyberworlds (CW),
pages 229–237.
Celiktutan, Avcibas, Sankur, Ayerden, and Capar (2006).
Source cell-phone identification. In 2006 IEEE 14th
Signal Processing and Communications Applications,
pages 1–3.
Chen, M., Fridrich, J., Goljan, M., and Lukas, J. (2008).
Determining image origin and integrity using sensor
noise. IEEE Transactions on Information Forensics
and Security, 3(1):74–90.
Chen, Y., Huang, Y., and Ding, X. (2017). Camera model
identification with residual neural network. In 2017
IEEE International Conference on Image Processing
(ICIP), pages 4337–4341.
Choi, K., Lam, E., and Wong, K. (2006). Source cam-
era identification using footprints from lens aberra-
tion. Proceedings of the SPIE, 6069.
Ding, X., Chen, Y., Tang, Z., and Huang, Y. (2019). Cam-
era identification based on domain knowledge-driven
deep multi-task learning. IEEE Access, 7:25878–
25890.
Farid, H. (2006). Digital image ballistics from jpeg quanti-
zation.
Galdi, C., Hartung, F., and Dugelay, J.-L. (2019). Socrates:
A database of realistic data for source camera recog-
nition on smartphones. In ICPRAM.
Galdi, C., Nappi, M., and Dugelay, J.-L. (2015). Combin-
ing hardwaremetry and biometry for human authen-
tication via smartphones. In Murino, V. and Puppo,
E., editors, Image Analysis and Processing — ICIAP
2015, pages 406–416, Cham. Springer International
Publishing.
Geradts, Z., Bijhold, J., Kieft, M., Kurosawa, K., Kuroki,
K., and Saitoh, N. (2001). Methods for identification
of images acquired with digital cameras. In SPIE Op-
tics East.
Gloe, T. and B
¨
ohme, R. (2010). The dresden image
database for benchmarking digital image forensics. J.
Digit. Forensic Pract., 3:150–159.
He, K., Zhang, X., Ren, S., and Sun, J. (2016). Deep resid-
ual learning for image recognition. In 2016 IEEE Con-
ference on Computer Vision and Pattern Recognition
(CVPR), pages 770–778.
Kharrazi, M., Sencar, H., and Memon, N. (2004). Blind
source camera identification. In 2004 International
Conference on Image Processing, 2004. ICIP ’04.,
volume 1, pages 709–712 Vol. 1.
Krizhevsky, A., Sutskever, I., and Hinton, G. E. (2017). Im-
agenet classification with deep convolutional neural
networks. Commun. ACM, 60(6):84–90.
Kroese, D. P., Brereton, T. J., Taimre, T., and Botev, Z. I.
(2014). Why the monte carlo method is so impor-
tant today. Wiley Interdisciplinary Reviews: Compu-
tational Statistics, 6:386–392.
Long, Y. and Huang, Y. (2006). Image based source cam-
era identification using demosaicking. In 2006 IEEE
Workshop on Multimedia Signal Processing, pages
419–424.
Lukas, J., Fridrich, J., and Goljan, M. (2006). Digital cam-
era identification from sensor pattern noise. IEEE
Transactions on Information Forensics and Security,
1(2):205–214.
Mandelli, S., Cozzolino, D., Bestagini, P., Verdoliva, L.,
and Tubaro, S. (2020). Cnn-based fast source de-
vice identification. IEEE Signal Processing Letters,
27:1285–1289.
Mayer, O. and Stamm, M. C. (2018). Learned forensic
source similarity for unknown camera models. In
2018 IEEE International Conference on Acoustics,
Speech and Signal Processing (ICASSP), pages 2012–
2016.
Mayer, O. and Stamm, M. C. (2020). Forensic similarity
for digital images. IEEE Transactions on Information
Forensics and Security, 15:1331–1346.
Tsai, M.-J. and Wu, G.-H. (2006). Using image features to
identify camera sources. In 2006 IEEE International
Conference on Acoustics Speech and Signal Process-
ing Proceedings, volume 2, pages II–II.
Van, L. T., Emmanuel, S., and Kankanhalli, M. S. (2007).
Identifying source cell phone using chromatic aberra-
tion. In 2007 IEEE International Conference on Mul-
timedia and Expo, pages 883–886.
Zhao, M., Wang, B., Wei, F., Zhu, M., and Sui, X. (2020).
Source camera identification based on coupling cod-
ing and adaptive filter. IEEE Access, 8:54431–54440.
ICPRAM 2022 - 11th International Conference on Pattern Recognition Applications and Methods
752