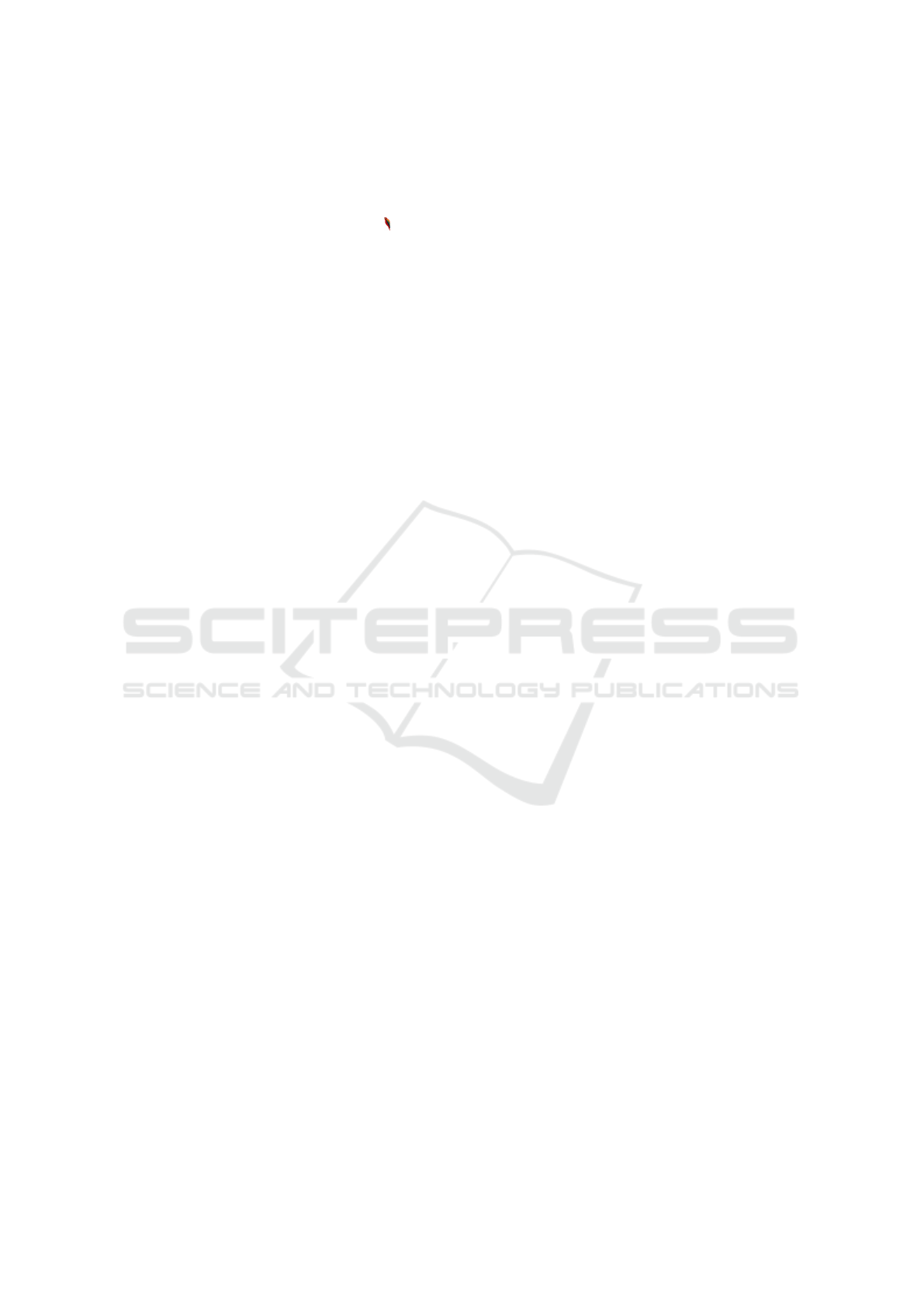
REFERENCES
Bender, E. M., Gebru, T., McMillan-Major, A., and
Shmitchell, S. (2021). On the dangers of stochastic
parrots: Can language models be too big? . In Proc.
of FAccT 2021.
Bommasani, R., Hudson, D. A., Adeli, E., Altman, R.,
Arora, S., von Arx, S., Bernstein, M. S., and et al.
(2021). On the opportunities and risks of foundation
models. CoRR.
Brixey, J., Hoegen, R., Lan, W., Rusow, J., Singla, K., Yin,
X., Artstein, R., and Leuski, A. (2017). SHIHbot:
A Facebook chatbot for sexual health information on
HIV/AIDS. In Proc. of the 18th Annual SIGdial Meet-
ing on Discourse and Dialogue. ACL.
Brown, T., Mann, B., Ryder, N., Subbiah, M., Kaplan, J. D.,
Dhariwal, P., Neelakantan, A., and et al. (2020). Lan-
guage models are few-shot learners. In NeurIPS.
Caliskan, A., Bryson, J., and Narayanan, A. (2017). Se-
mantics derived automatically from language corpora
contain human-like biases. Science.
Chen, H., Liu, X., Yin, D., and Tang, J. (2017). A survey on
dialogue systems: Recent advances and new frontiers.
SIGKDD Explor. Newsl.
Denecke, K., Vaaheesan, S., and Arulnathan, A. (2021). A
mental health chatbot for regulating emotions (sermo)
- concept and usability test. IEEE Transactions on
Emerging Topics in Computing.
Devlin, J., Chang, M.-W., Lee, K., and Toutanova, K.
(2019). BERT: Pre-training of deep bidirectional
transformers for language understanding. In Proc. of
the NAACL 2019: Human Language Technologies.
Floridi, L. and Chiriatti, M. (2020). Gpt-3: Its nature, scope,
limits, and consequences. Minds and Machines.
Gatt, A. and Krahmer, E. (2018). Survey of the state of the
art in natural language generation: Core tasks, appli-
cations and evaluation. JAIR.
Gu, J.-C., Li, T., Liu, Q., Ling, Z.-H., Su, Z., Wei, S., and
Zhu, X. (2020). Speaker-aware bert for multi-turn re-
sponse selection in retrieval-based chatbots. In Proc.
of the CIKM’20. ACM.
Gururangan, S., Marasovi
´
c, A., Swayamdipta, S., Lo, K.,
Beltagy, I., Downey, D., and Smith, N. A. (2020).
Don’t stop pretraining: Adapt language models to do-
mains and tasks. In Proc. of the 58th ACL.
He, L., Basar, E., Wiers, R., Antheunis, M., and Krah-
mer, E. (2022). Can chatbots support smoking ces-
sation? a study on the effectiveness of motivational
interviewing on engagement and therapeutic alliance.
Manuscript submitted for publication.
Krahmer, E., Bosse, T., and Bruijn, G.-J. (2021). Chatbots
and health: General. The International Encyclopedia
of Health Communication.
Li, L., Li, C., and Ji, D. (2021). Deep context modeling
for multi-turn response selection in dialogue systems.
Information Processing & Management.
Mikolov, T., Sutskever, I., Chen, K., Corrado, G., and Dean,
J. (2013). Distributed representations of words and
phrases and their compositionality. In Proc. of the
26th International NeurIPS.
Novikova, J., Du
ˇ
sek, O., Cercas Curry, A., and Rieser, V.
(2017). Why we need new evaluation metrics for
NLG. In Proc. of EMNLP 2017. ACL.
Peters, M. E., Neumann, M., Iyyer, M., Gardner, M., Clark,
C., Lee, K., and Zettlemoyer, L. (2018). Deep contex-
tualized word representations. In Proc. of NAACL.
Rousseau, A.-L., Baudelaire, C., and Riera, K.
(2020). Doctor gpt-3: Hype or reality?
https://www.nabla.com/blog/gpt-3/. Accessed:
2021-10-18.
Saha, T., Chopra, S., Saha, S., Bhattacharyya, P., and Ku-
mar, P. (2021). A large-scale dataset for motivational
dialogue system: An application of natural language
generation to mental health. In IJCNN 2021.
Schlesinger, A., O’Hara, K., and Taylor, A. S. (2018). Let’s
talk about race: Identity, chatbots, and ai. In ACM
Conference on Human Factors in Computing Systems.
See, A., Roller, S., Kiela, D., and Weston, J. (2019). What
makes a good conversation? how controllable at-
tributes affect human judgments. In Proc. of NAACL:
Human Language Technologies. ACL.
Song, Y., Li, C.-T., Nie, J.-Y., Zhang, M., Zhao, D., and
Yan, R. (2018). An ensemble of retrieval-based and
generation-based human-computer conversation sys-
tems. In Proc. of IJCAI-18.
Sutskever, I., Vinyals, O., and Le, Q. V. (2014). Sequence to
sequence learning with neural networks. In NeurIPS.
Tao, C., Gao, S., Shang, M., Wu, W., Zhao, D., and Yan,
R. (2018). Get the point of my utterance! learning
towards effective responses with multi-head attention
mechanism. In Proc. of IJCAI-18.
van der Lee, C., Gatt, A., van Miltenburg, E., and Krahmer,
E. (2021). Human evaluation of automatically gener-
ated text: Current trends and best practice guidelines.
Computer Speech & Language.
Vinyals, O. and Le, Q. V. (2015). A neural conversational
model. In ICML Deep Learning Workshop.
Whang, T., Lee, D., Lee, C., Yang, K., Oh, D., and Lim,
H. (2020). An effective domain adaptive post-training
method for bert in response selection. In Proc. Inter-
speech 2020.
Xu, B. and Zhuang, Z. (2020). Survey on psychotherapy
chatbots. Concurrency and Computation: Practice
and Experience.
Yang, L., Hu, J., Qiu, M., Qu, C., Gao, J., Croft, W. B., Liu,
X., Shen, Y., and Liu, J. (2019). A hybrid retrieval-
generation neural conversation model. In Proc. of
CIKM’19. ACM.
Zhou, L., Gao, J., Li, D., and Shum, H.-Y. (2020). The
design and implementation of xiaoice, an empathetic
social chatbot. Computational Linguistics.
Hints of Independence in a Pre-scripted World: On Controlled Usage of Open-domain Language Models for Chatbots in Highly Sensitive
Domains
407