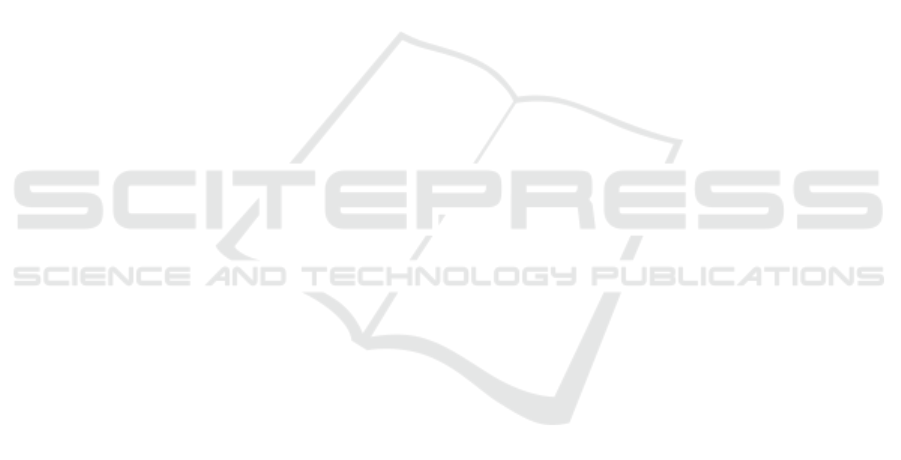
detected with IoU greater than 0.3. With IoU above
0.3, the task of locating animals becomes very easy in
extremely low light and low contrast images.
5 CONCLUSION
In this work, we address the empty frame removal
problem and the animal detection challenge in camera
trap sequences. In tandem, we investigate the applica-
bility of ViT, DETR, and Faster R-CNN for this task.
Our experiments reaffirm the generalisation gap in the
context of unseen test data. We culminate our experi-
mental study with proposal of a two-stage pipeline for
mining vital statistics from camera trap sequences. In
the first stage we filter out empty frames and in the
second stage, we perform wildlife detection and local-
isation. Balancing the trade-off between retaining all
frames containing animals and filtering out all empty
frames we adopt ViT(best model on ‘cis’) for remov-
ing empty frames and DETR for detecting animals.
Despite heavy background clutter, camouflage, size
and pose variations, occlusion, progressive illumina-
tion changes from day to night, and seasonal varia-
tions in flora and fauna in camera trap data we ob-
tain a competitive accuracy. We shall extend our work
to make the empty frame removal and animal detec-
tion pipeline even more robust, especially under ex-
treme low-light and low-contrast conditions. Hence,
develop practically deployable wildlife detection sys-
tems. Further, we plan to incorporate open set recog-
nition, zero-shot learning, and few-shot learning for
generalising to unseen locations.
ACKNOWLEDGEMENTS
This work is partially supported by National
Mission for Himalayan Studies (NMHS) grant
GBPNI/NMHS-2019-20/SG/314
REFERENCES
Bay, H., Tuytelaars, T., and Van Gool, L. (2006). Surf:
Speeded up robust features. In European conference
on computer vision, pages 404–417. Springer.
Beery, S., Van Horn, G., Mac Aodha, O., and Perona, P.
(2019). The iwildcam 2018 challenge dataset. arXiv
preprint arXiv:1904.05986.
Beery, S., Van Horn, G., and Perona, P. (2018). Recognition
in terra incognita. In Proceedings of the European
Conference on Computer Vision (ECCV), pages 456–
473.
Carion, N., Massa, F., Synnaeve, G., Usunier, N., Kirillov,
A., and Zagoruyko, S. (2020). End-to-end object de-
tection with transformers. In European Conference on
Computer Vision, pages 213–229. Springer.
Cortes, C. and Vapnik, V. (1995). Support-vector networks.
Machine learning, 20(3):273–297.
Cunha, F., dos Santos, E. M., Barreto, R., and Colonna,
J. G. (2021). Filtering empty camera trap images in
embedded systems. In Proceedings of the IEEE/CVF
Conference on Computer Vision and Pattern Recogni-
tion (CVPR) Workshops, pages 2438–2446.
Dalal, N. and Triggs, B. (2005). Histograms of oriented
gradients for human detection. In 2005 IEEE com-
puter society conference on computer vision and pat-
tern recognition (CVPR’05), volume 1, pages 886–
893. Ieee.
Dosovitskiy, A., Beyer, L., Kolesnikov, A., Weissenborn,
D., Zhai, X., Unterthiner, T., Dehghani, M., Minderer,
M., Heigold, G., Gelly, S., et al. (2020). An image is
worth 16x16 words: Transformers for image recogni-
tion at scale. arXiv preprint arXiv:2010.11929.
Emami, E. and Fathy, M. (2011). Object tracking using
improved camshift algorithm combined with motion
segmentation. pages 1–4.
Figueroa, K., Camarena-Ibarrola, A., Garc
´
ıa, J., and Vil-
lela, H. T. (2014). Fast automatic detection of wildlife
in images from trap cameras. In Iberoamerican
Congress on Pattern Recognition, pages 940–947.
Springer.
Girshick, R. (2015). Fast r-cnn. In Proceedings of the IEEE
international conference on computer vision, pages
1440–1448.
Girshick, R., Donahue, J., Darrell, T., and Malik, J. (2014).
Rich feature hierarchies for accurate object detec-
tion and semantic segmentation. In Proceedings of
the IEEE conference on computer vision and pattern
recognition, pages 580–587.
Guo, Z., Zhang, L., and Zhang, D. (2010). A completed
modeling of local binary pattern operator for texture
classification. IEEE transactions on image process-
ing, 19(6):1657–1663.
He, K., Zhang, X., Ren, S., and Sun, J. (2016). Deep resid-
ual learning for image recognition. In Proceedings of
the IEEE conference on computer vision and pattern
recognition, pages 770–778.
Hidayatullah, P. and Konik, H. (2011). Camshift im-
provement on multi-hue and multi-object tracking. In
Proceedings of the 2011 International Conference on
Electrical Engineering and Informatics, pages 1–6.
Krizhevsky, A., Sutskever, I., and Hinton, G. E. (2012). Im-
agenet classification with deep convolutional neural
networks. Advances in neural information processing
systems, 25:1097–1105.
Lin, M., Chen, Q., and Yan, S. (2013). Network in network.
arXiv preprint arXiv:1312.4400.
Lindeberg, T. (2012). Scale invariant feature transform.
Liu, W., Anguelov, D., Erhan, D., Szegedy, C., Reed, S.,
Fu, C.-Y., and Berg, A. C. (2016). Ssd: Single shot
multibox detector. In European conference on com-
puter vision, pages 21–37. Springer.
ICPRAM 2022 - 11th International Conference on Pattern Recognition Applications and Methods
478